Improving the representation of the spatial distribution of population for coastal impact and vulnerability assessments
semanticscholar(2020)
AI Read Science
Must-Reading Tree
Example
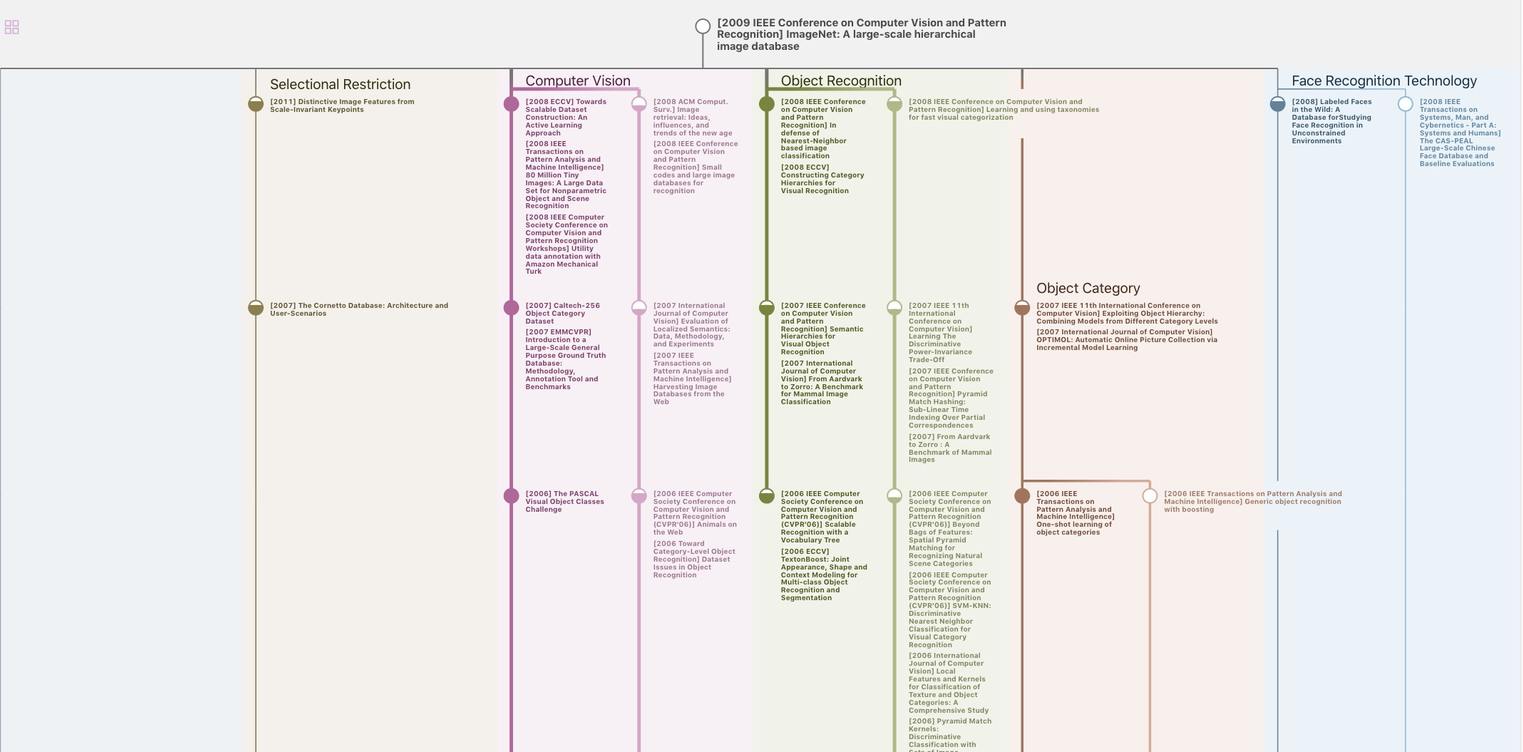
Generate MRT to find the research sequence of this paper
Chat Paper
Summary is being generated by the instructions you defined