Inferring Causation from Correlation in Sparse Networks
semanticscholar(2014)
摘要
We discuss the applicability of a recently proposed method to reconstruct cerebral network structure (Pernice and Rotter, 2013) on the data supplied in the Neural Connectomics Challenge. This method is based on a model of linear dynamics which provides a relation between activity covariances and the underlying network. Even for very limited sampling resolution, an estimate of the directed connections can be obtained under the assumption of sparse connectivity, if indirect connections contribute significantly to the covariance matrix. Surprisingly, and possibly due to the low importance of indirect connections in the provided data, the sparsest estimator resulted in only limited performance. Our best estimation relies on the inverse covariance matrix which has been adapted based on insights gained from the model.
更多查看译文
AI 理解论文
溯源树
样例
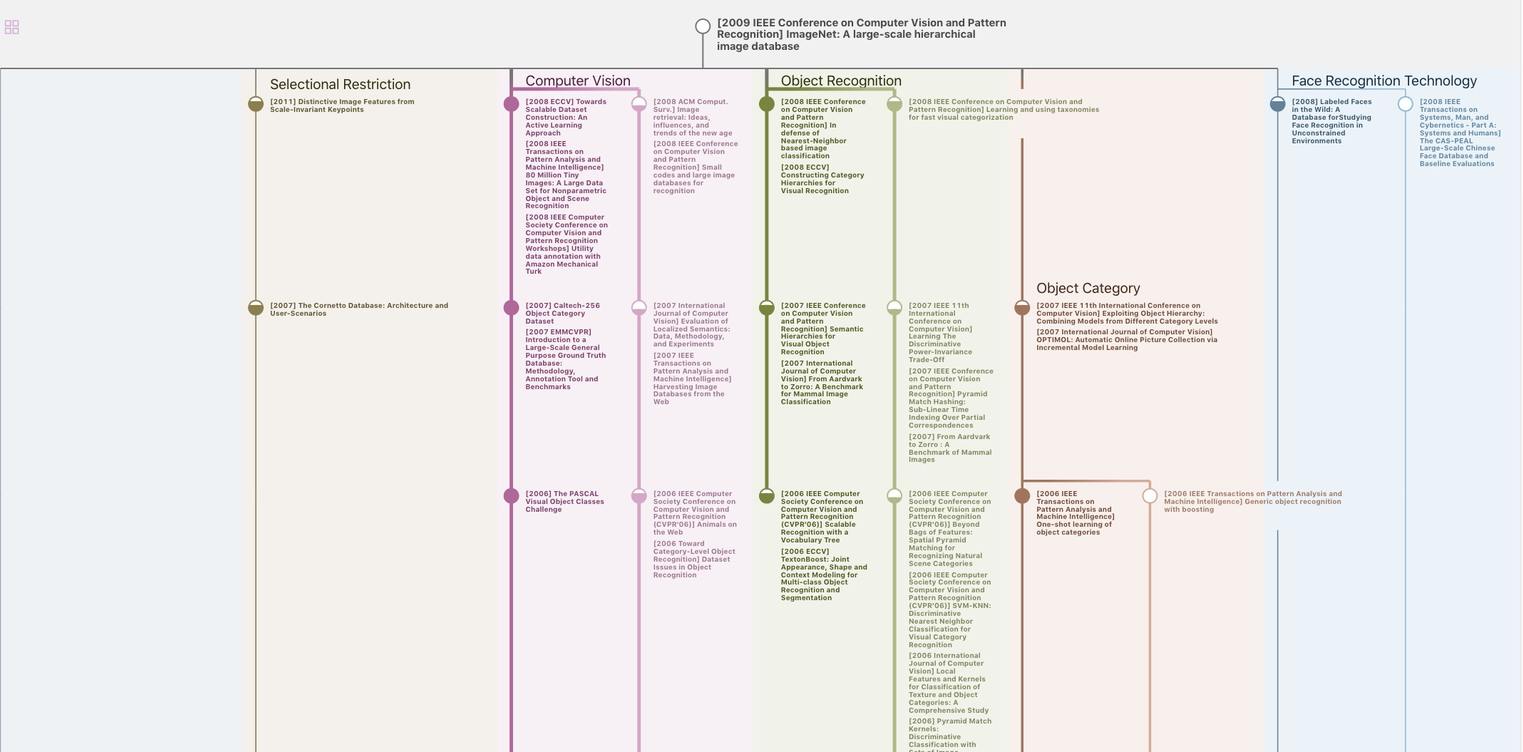
生成溯源树,研究论文发展脉络
Chat Paper
正在生成论文摘要