Mder_a_221029 13..22
semanticscholar(2020)
摘要
Magd Ahmed Kotb 1 Hesham Nabih Elmahdy 2 Hadeel Mohamed Seif El Dein Fatma Zahraa Mostafa 1 Mohammed Ahmed Refaey Khaled Waleed Younis Rjoob 2 Iman H Draz Christine William Shaker Basanti 1 1Department of Pediatrics, Faculty of Medicine, Cairo University, Cairo, Egypt; 2Information Technology Department, Vice-Dean of Faculty of Computers and Information, Cairo University, Giza, Egypt; 3Department of Diagnostic and Interventional Radiology, Faculty of Medicine, Cairo University, Cairo, Egypt Introduction: Contemporary stethoscope has limitations in diagnosis of chest conditions, necessitating further imaging modalities. Methods: We created 2 diagnostic computer aided non-invasive machine-learning models to recognize chest sounds. Model Awas interpreter independent based on hidden markov model and mel frequency cepstral coefficient (MFCC). Model B was based onMFCC, hidden markov model, and chest sound wave image interpreter dependent analysis (phonopulmonography (PPG)). Results: We studied 464 records of actual chest sounds belonging to 116 children diagnosed by clinicians and confirmed by other imaging diagnostic modalities. Model A had 96.7% overall correct classification rate (CCR), 100% sensitivity and 100% specificity in discrimination between normal and abnormal sounds. CCR was 100% for normal vesicular sounds, crepitations 89.1%, wheezes 97.6%, and bronchial breathing 100%. Model B's CCR was 100% for normal vesicular sounds, crepitations 97.3%, wheezes 97.6%, and bronchial breathing 100%. The overall CCR was 98.7%, sensitivity and specificity were 100%. Conclusion: Both models demonstrated very high precision in the diagnosis of chest conditions and in differentiating normal from abnormal chest sounds irrespective of operator expertise. Incorporation of computer-aided models in stethoscopes promises prompt, precise, accurate, cost-effective, non-invasive, operator independent, objective diagnosis of chest conditions and reduces number of unnecessary imaging studies.
更多查看译文
AI 理解论文
溯源树
样例
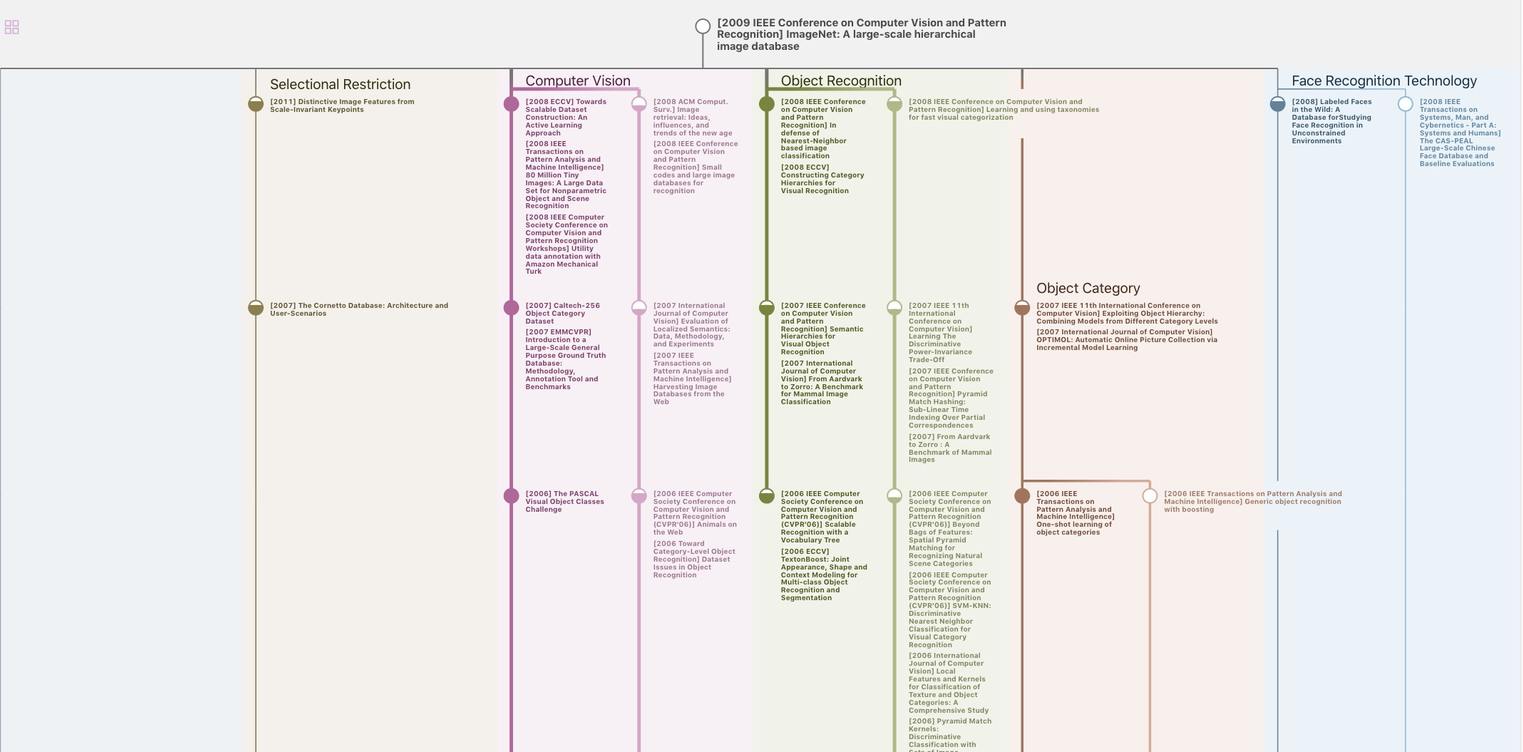
生成溯源树,研究论文发展脉络
Chat Paper
正在生成论文摘要