Coupled Learning Enabled Stochastic Programming with Endogenous Uncertainty
MATHEMATICS OF OPERATIONS RESEARCH(2022)
摘要
Predictive analytics, empowered by machine learning, is usually followed by decision-making problems in prescriptive analytics. We extend the previous sequential prediction-optimization paradigm to a coupled scheme such that the prediction model can guide the decision problem to produce coordinated decisions yielding higher levels of performance. Specifically, for stochastic programming (SP) models with latently decision-dependent uncertainty, without any parametric assumption of the latent dependency, we develop a coupled learning enabled optimization (CLEO) algorithm in which the learning step of predicting the local dependency and the optimization step of computing a candidate decision are conducted interactively. The CLEO algorithm automatically balances the exploration and exploitation via the trust region method with active sampling. Under certain assumptions, we show that the sequence of solutions provided by CLEO converges to a directional stationary point of the original nonconvex and nonsmooth SP problem with probability 1. In addition, we present preliminary experimental results which demonstrate the computational potential of this data-driven approach.
更多查看译文
关键词
stochastic programming,endogenous uncertainty,trust region method,local linear regression
AI 理解论文
溯源树
样例
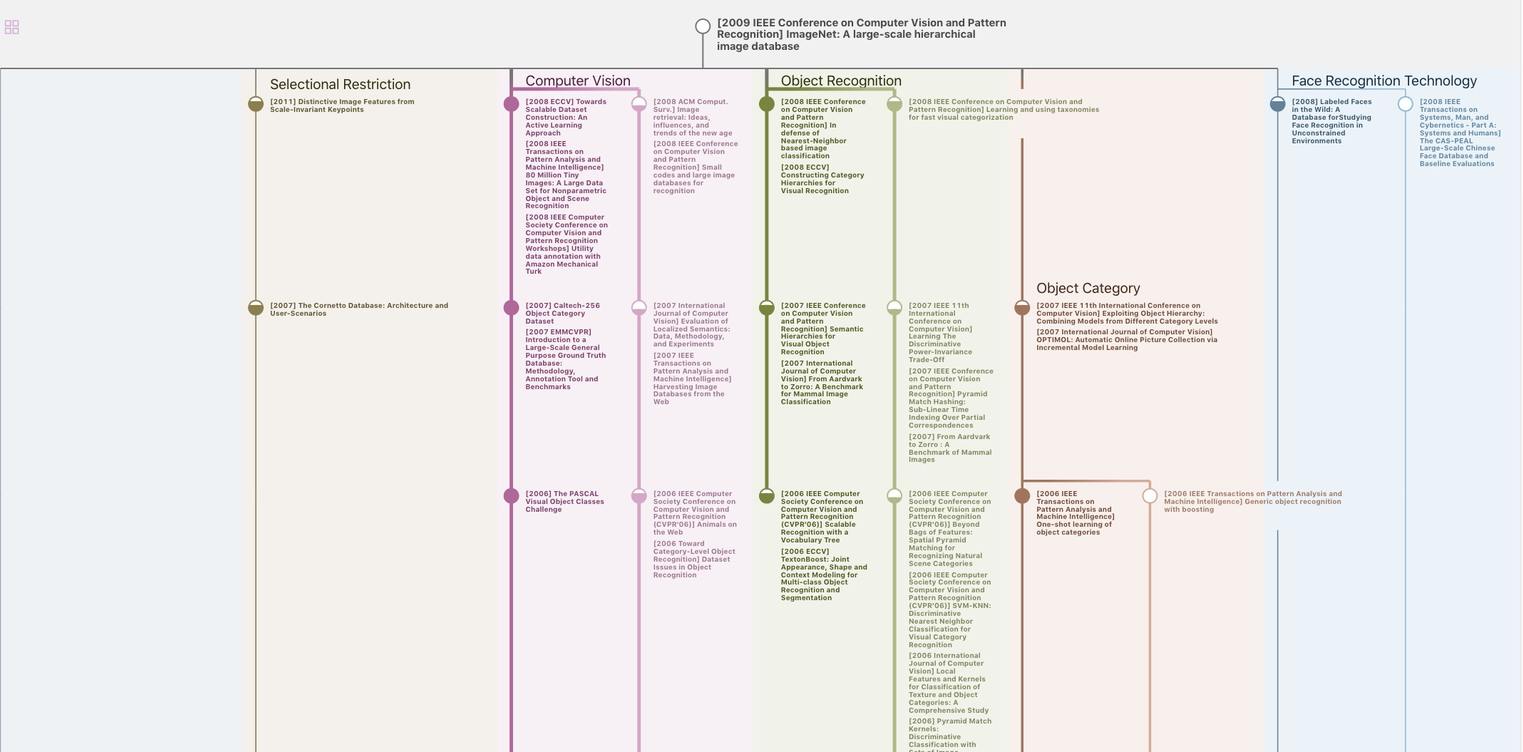
生成溯源树,研究论文发展脉络
Chat Paper
正在生成论文摘要