Optimal Portfolio Allocation with Statistical Learning ∗
semanticscholar(2018)
摘要
This paper exploits the idea of pretesting to choose between competing portfolio strategies. We propose a strategy that optimally trades off between the risk of going for a false positive strategy choice versus the risk of making a false negative choice. Various different data driven approaches are proposed based on an optimal choice of the pretested certainty equivalent and Sharpe Ratio. Our approach belongs to the class of shrinkage portfolio estimators. However, contrary to previous approaches the shrinkage intensity is continuously updated to incorporate the most recent information in the rolling window forecasting set-up. We show that the bagged pretest estimator performs exceptionally well, especially when combined with adaptive smoothing. The resulting strategy allows for a flexible and smooth switch between the underlying strategies and is shown to outperform the corresponding stand-alone strategies.
更多查看译文
AI 理解论文
溯源树
样例
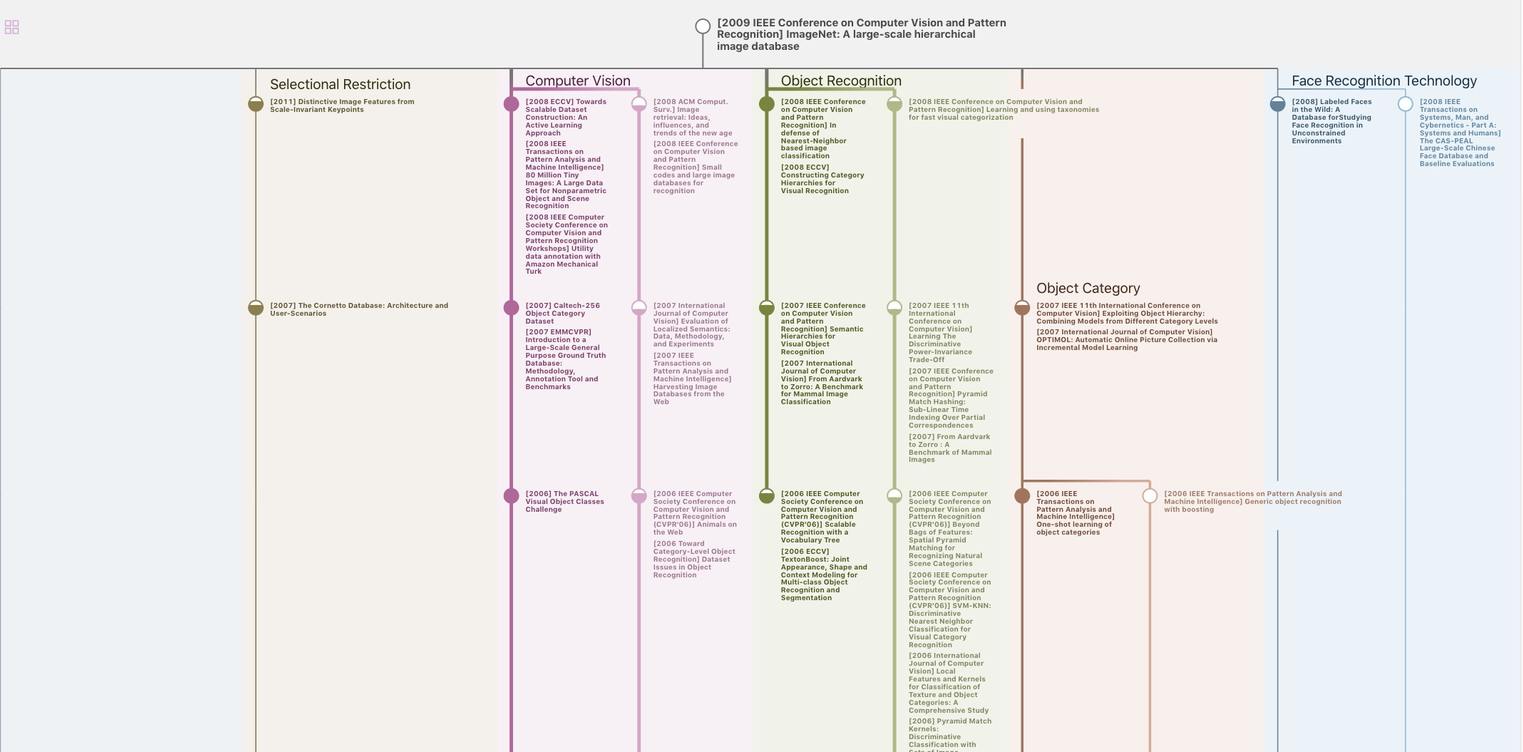
生成溯源树,研究论文发展脉络
Chat Paper
正在生成论文摘要