Multiobjective Behavioral Portfolio Selection with Efficient ESG Factors and Learning Network Estimation of Asset Returns
semanticscholar(2020)
摘要
This paper provides an aspirational multiple objective framework for behavioral portfolio modeling (BPM) for both the portfolio selection and rebalancing decision. The aim is to extend BPM to a framework that has the flexibility to incorporate newly extracted ESG factors that proxy investor sustainability bias. The proposed framework complements the transition from modern portfolio theory to behavioral portfolio theory with support for dynamic rebalancing. Assuming sustainability bias is proxied by investor attention for firm ESG production, we extract three new ESG factors from the Thompson Reuters large-cap ESG portfolio database. These same factors also serve as stylistic control variables in a machine-learning algorithm when modeling Fama-French asset returns. As such, this research incapsulates recent findings that challenge characteristic-based asset return prediction by implicating a shallow learning radial basis function neural network with production-theoretic signals. Lastly, we model loss aversion bias by dynamically estimating an option-priced CVaR metric. The results of solving the aspirational multiobjective BPM provide three important observations. We find that when only two hierarchical goal objectives are specified the BPM approximates the traditional mean-variance solution. Secondly, alternate model specifications demonstrate the importance of wealth goal setting when there is investor ESG sustainability bias. Lastly, research findings demonstrate the importance of dynamically estimating CVaR metrics to achieve aspirational goals related to loss-aversion bias.
更多查看译文
AI 理解论文
溯源树
样例
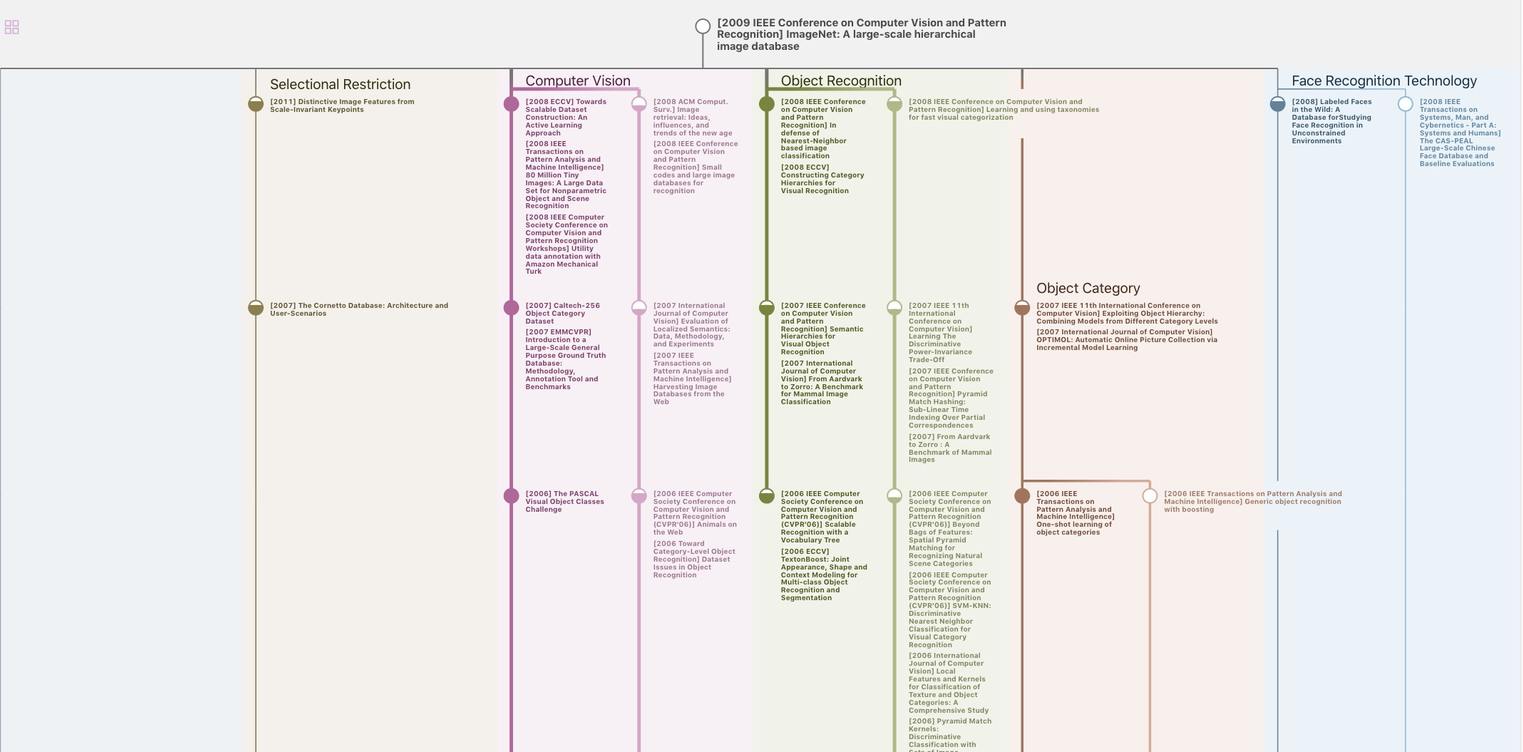
生成溯源树,研究论文发展脉络
Chat Paper
正在生成论文摘要