Drug Repurposing Using a Semantic Knowledge Graph
semanticscholar(2017)
摘要
Given the significant time and financial costs of developing a commercial drug, it remains important to constantly reform the drug discovery pipeline with novel technologies that can narrow the candidates down to the most promising lead compounds for clinical testing. Computational approaches are used to expedite the drug discovery processes. Semantic knowledge graphs can assist these computational approaches, because they connect different biological databases and reflect the relationships between genes, pathways and diseases. Here, we took advantage of the Euretos Knowledge Platform (EKP), a commercial database that integrates more than 170 different biological resources including DrugBank, and evaluated the usefulness of the underlying semantic knowledge graphs to predict novel drug-disease associations. As a positive set, we extracted 403 drug-disease associations from an independent resource, Metab2MeSH. An equally sized negative set was created by reshuffling of these drug-disease triples. For prediction of new associations, it is important to assess which paths in the knowledge graph optimally represent novel drug-disease associations. All 403 drug-disease associations in the positive and negative dataset were connected by at least one intermediate concept from 12 out of the 14 semantic types available in EKP. ‘Chemicals & Drugs’ was the most informative intermediate semantic type and distinguished the positive and negative sets best (Kolmogorov-Smirnov p-value: 7.4. 10-23). Also graph network features such as the total number of intermediate concepts (count), the number of different semantic categories (diversity), and the predicates connecting a drug-disease pair were successful in separating the positive from the negative sets. These features can be used to build a classifier for the prediction of novel drug-disease associations from the Euretos Knowledge Platform facilitating drug repurposing in preclinical research.
更多查看译文
AI 理解论文
溯源树
样例
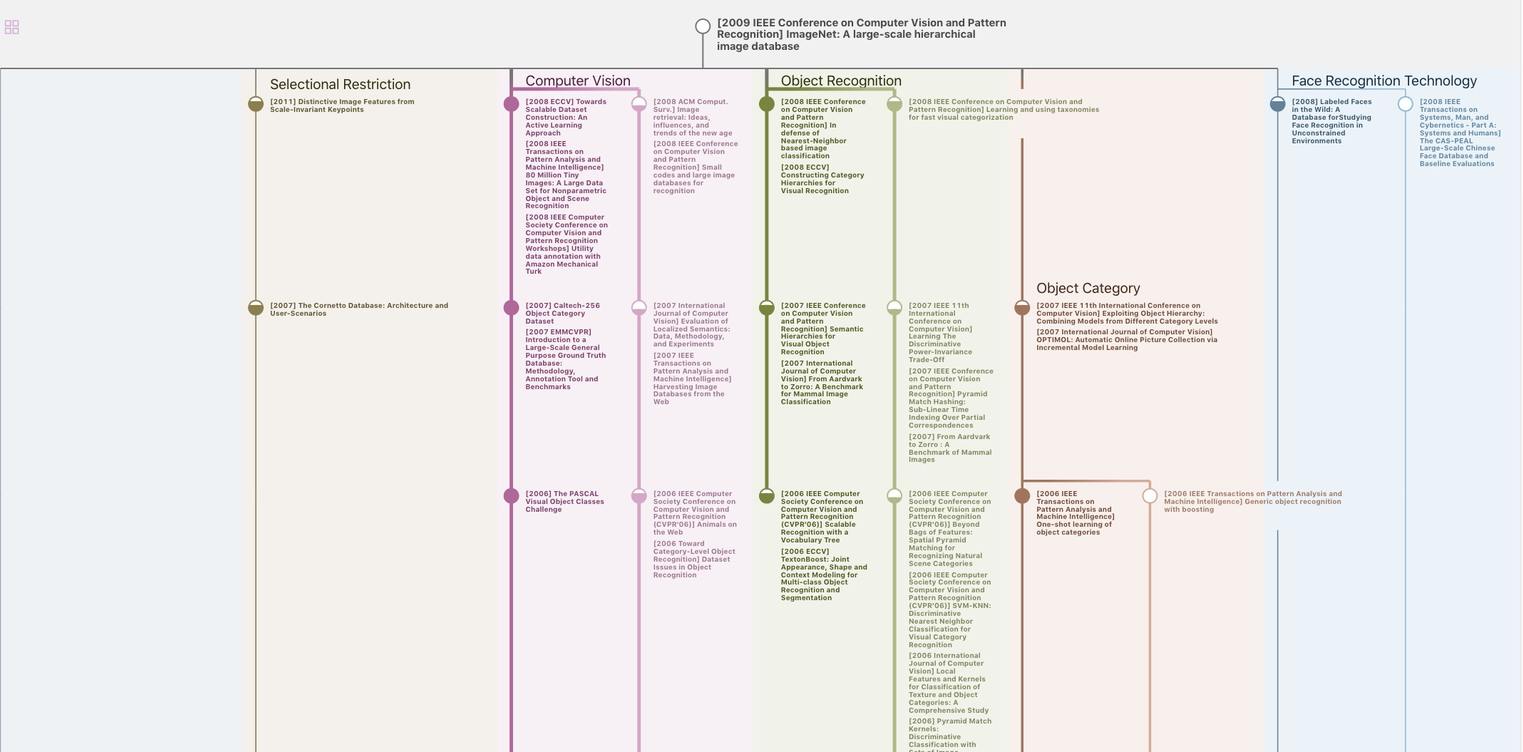
生成溯源树,研究论文发展脉络
Chat Paper
正在生成论文摘要