Efficient Transfer Learning on Modeling Physical Unclonable Functions
PROCEEDINGS OF THE TWENTYFIRST INTERNATIONAL SYMPOSIUM ON QUALITY ELECTRONIC DESIGN (ISQED 2020)(2020)
摘要
Physical Unclonable Function (PUF) is seen as a promising alternative to traditional cryptographic algorithms for secure and lightweight device authentication for the diverse IoT use cases. However, the essential security of PUF is threatened by a kind of machine learning (ML) based modeling attacks which could successfully impersonate the PUF by using known challenge and response pairs (CPRs). However, existing modeling methods require access to an extremely large set of CRPs which makes them unrealistic and impractical in the real world scenarios. To handle the limitation of available CRPs from the attack perspective, we explore the possibility to transfer a well-tuned model trained with unlimited CRPs to a target PUF with limited number of CRPs. Experimental results show that the proposed transfer learning-based scheme could achieve the same accuracy level with 64% less of CRPs in average. Besides, we also evaluate the proposed transfer learning method with side-channel information and it demonstrates in reducing the number of CRPs significantly.
更多查看译文
关键词
PUF,Modeling Attacks,Transfer Learning
AI 理解论文
溯源树
样例
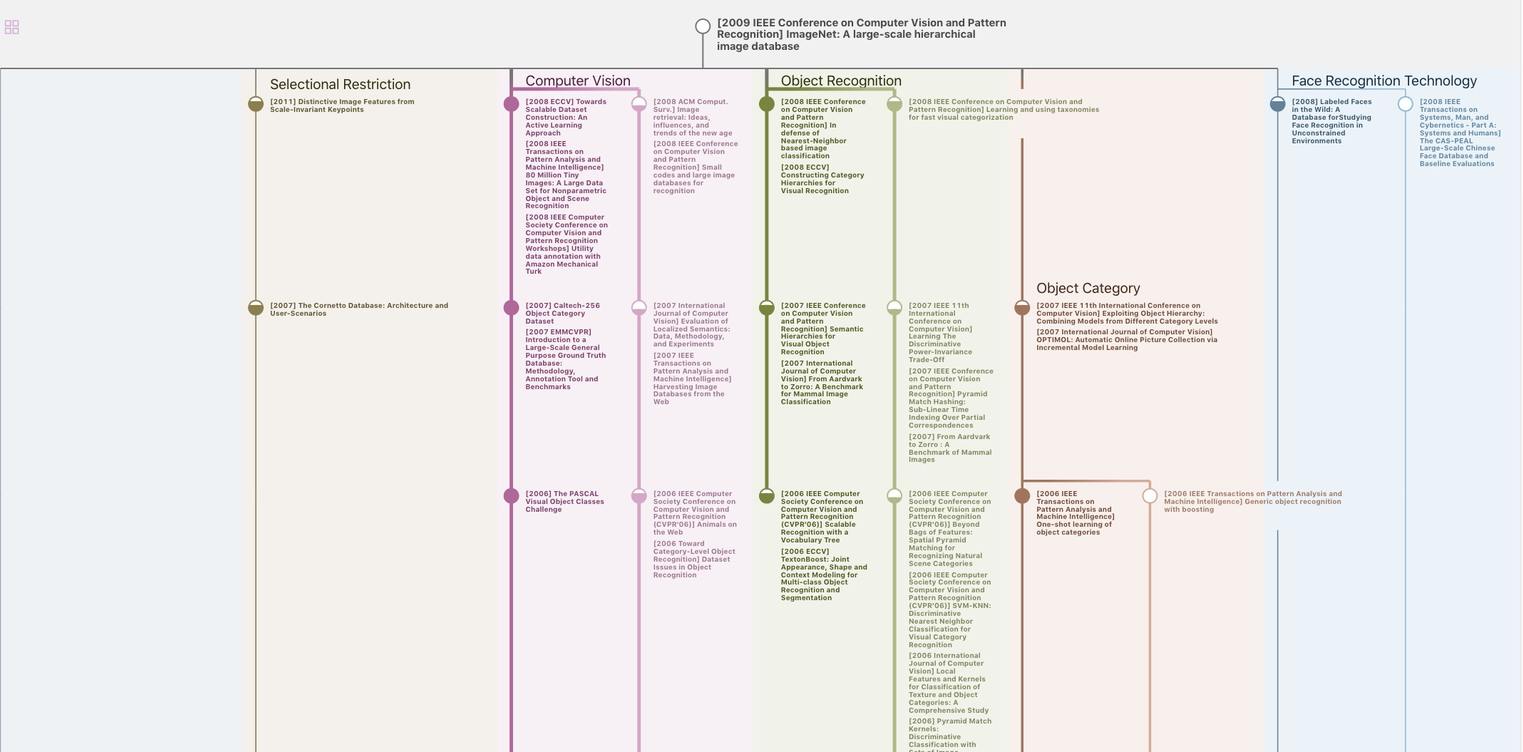
生成溯源树,研究论文发展脉络
Chat Paper
正在生成论文摘要