Traffic Agent Trajectory Prediction Using Social Convolution and Attention Mechanism
2020 IEEE Intelligent Vehicles Symposium (IV)(2020)
摘要
The trajectory prediction is significant for the decision-making of autonomous driving vehicles. In this paper, we propose a model to predict the trajectories of target agents around an autonomous vehicle. The main idea of our method is considering the history trajectories of the target agent and the influence of surrounding agents on the target agent. To this end, we encode the target agent history trajectories as an attention mask and construct a social map to encode the interactive relationship between the target agent and its surrounding agents. Given a trajectory sequence, the LSTM networks are firstly utilized to extract the features for all agents, based on which the attention mask and social map are formed. Then, the attention mask and social map are fused to get the fusion feature map, which is processed by the social convolution to obtain a fusion feature representation. Finally, this fusion feature is taken as the input of a variable-length LSTM to predict the trajectory of the target agent. We note that the variable-length LSTM enables our model to handle the case that the number of agents in the sensing scope is highly dynamic in traffic scenes. To verify the effectiveness of our method, we widely compare with several methods on a public dataset, achieving a 20% error decrease. In addition, the model satisfies the real-time requirement with the 32 fps.
更多查看译文
关键词
traffic agent trajectory prediction,social convolution,surrounding agents,target agent history trajectories,attention mask,social map,trajectory sequence,variable-length LSTM,public dataset,real-time requirement
AI 理解论文
溯源树
样例
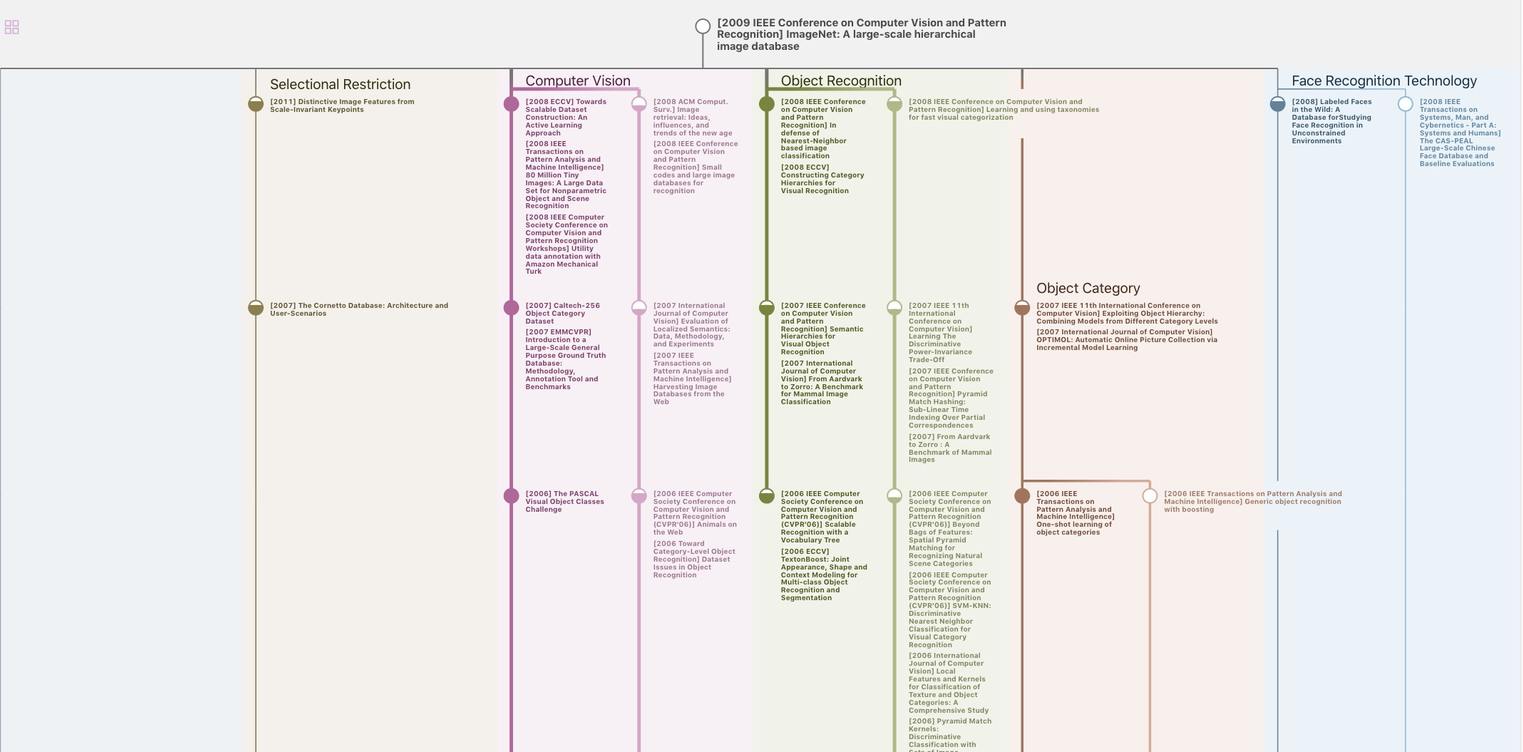
生成溯源树,研究论文发展脉络
Chat Paper
正在生成论文摘要