Multimodal Learning with Incomplete Modalities by Knowledge Distillation
KDD '20: The 26th ACM SIGKDD Conference on Knowledge Discovery and Data Mining Virtual Event CA USA July, 2020(2020)
Abstract
Multimodal learning aims at utilizing information from a variety of data modalities to improve the generalization performance. One common approach is to seek the common information that is shared among different modalities for learning, whereas we can also fuse the supplementary information to leverage modality-specific information. Though the supplementary information is often desired, most existing multimodal approaches can only learn from samples with complete modalities, which wastes a considerable amount of data collected. Otherwise, model-based imputation needs to be used to complete the missing values and yet may introduce undesired noise, especially when the sample size is limited. In this paper, we proposed a framework based on knowledge distillation, utilizing the supplementary information from all modalities, and avoiding imputation and noise associated with it. Specifically, we first train models on each modality independently using all the available data. Then the trained models are used as teachers to teach the student model, which is trained with the samples having complete modalities. We demonstrate the effectiveness of the proposed method in extensive empirical studies on both synthetic datasets and real-world datasets.
MoreTranslated text
Key words
Multimodal Learning, Knowledge Distillation, Incomplete Modalities
AI Read Science
Must-Reading Tree
Example
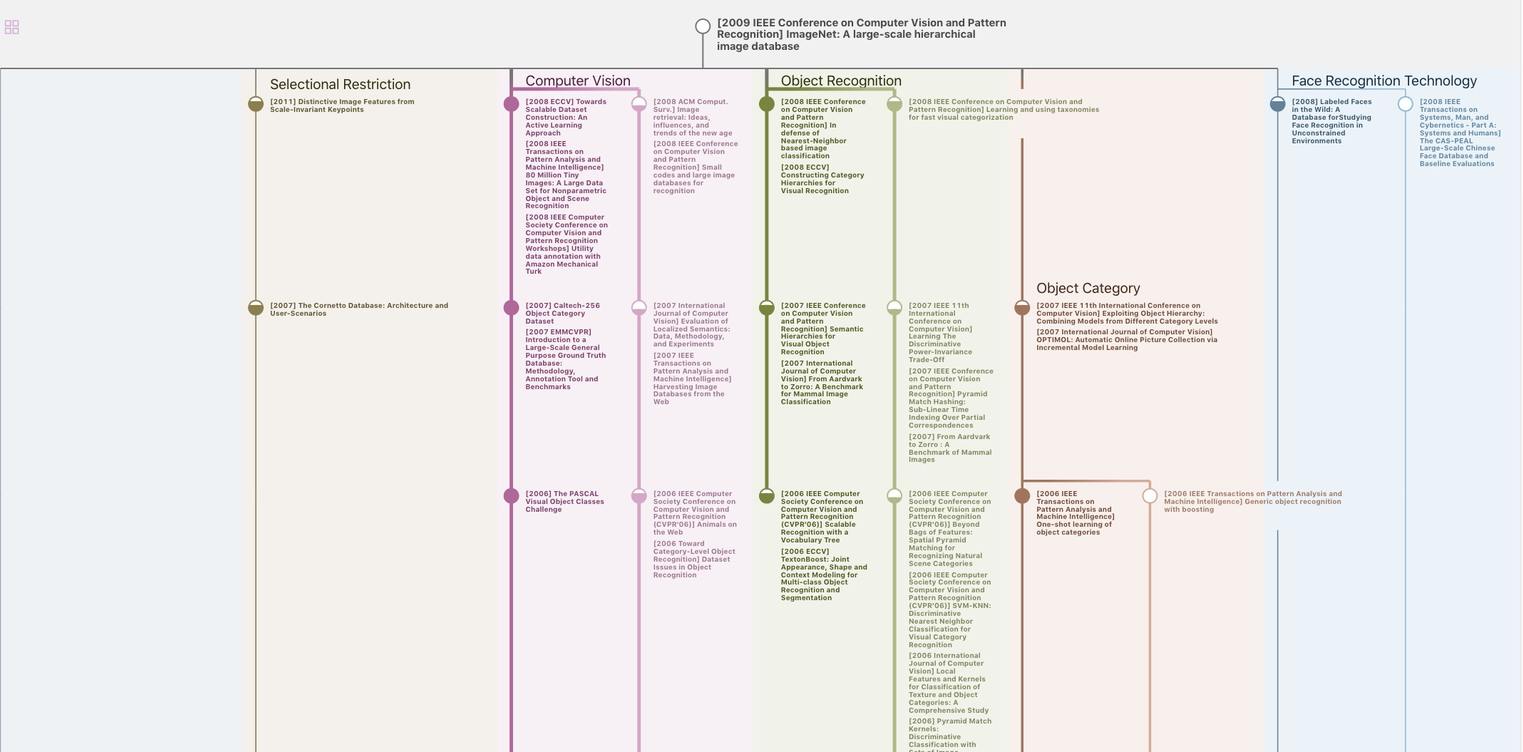
Generate MRT to find the research sequence of this paper
Chat Paper
Summary is being generated by the instructions you defined