Nonparametric method for space conditional density estimation in moderately large dimensions
arxiv(2018)
摘要
In this paper, we consider the problem of estimating a conditional density in moderately large dimensions. Much more informative than regression functions, conditional densities are of main interest in recent methods, particularly in the Bayesian framework (studying the posterior distribution, finding its modes...). Considering a recently studied family of kernel estimators, we select a pointwise multivariate bandwidth by revisiting the greedy algorithm Rodeo (Regularisation Of Derivative Expectation Operator). The method addresses several issues: being greedy and computationally efficient by an iterative procedure, avoiding the curse of high dimensionality under some suitably defined sparsity conditions by early variable selection during the procedure, converging at a quasi-optimal minimax rate.
更多查看译文
AI 理解论文
溯源树
样例
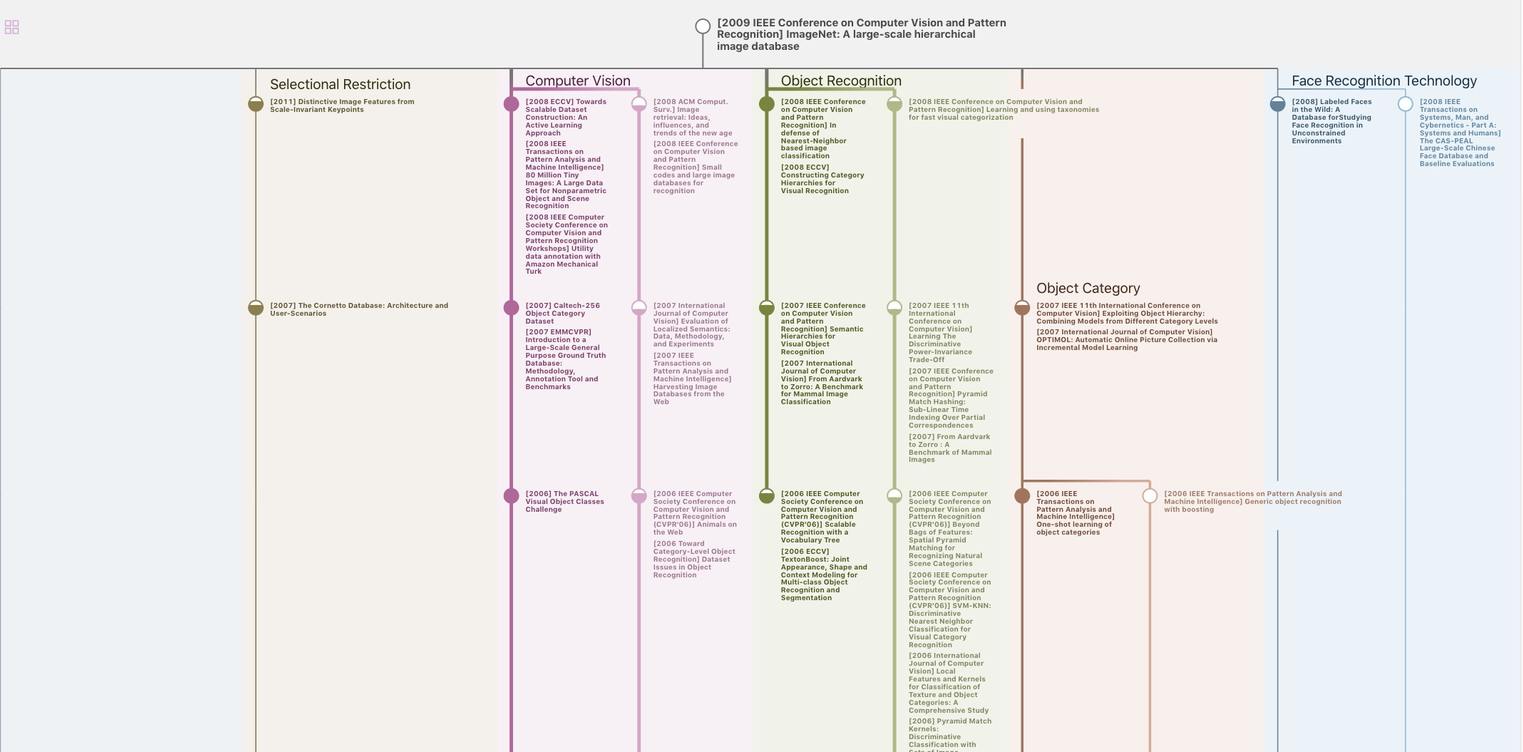
生成溯源树,研究论文发展脉络
Chat Paper
正在生成论文摘要