Understanding Finite-State Representations of Recurrent Policy Networks
arxiv(2020)
摘要
We introduce an approach for understanding finite-state machine (FSM) representations of recurrent policy networks. Recent work focused on minimizing FSMs to gain high-level insight, however, minimization can obscure a deeper understanding by merging states that are semantically distinct. Conversely, our approach starts with an unminimized machine and applies more-interpretable reductions that preserve the key decision points of the policy. We also contribute a saliency tool to attain a deeper understanding of the role of observations in the decisions. Our case studies on policies from 7 Atari games and 3 control benchmarks demonstrate that the approach can reveal insights that have not been noticed in prior work.
更多查看译文
关键词
policy,finite-state
AI 理解论文
溯源树
样例
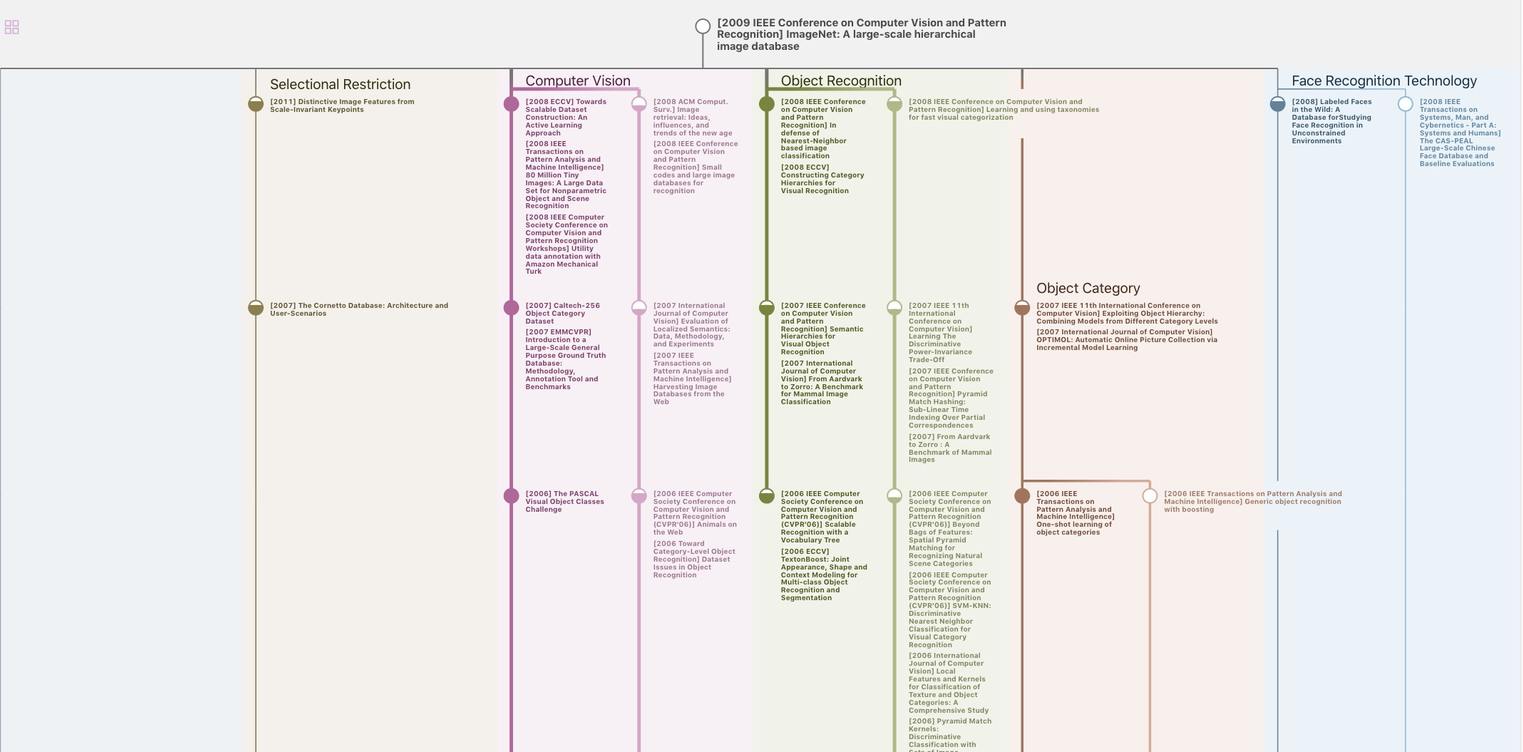
生成溯源树,研究论文发展脉络
Chat Paper
正在生成论文摘要