City-Scale Multi-Camera Vehicle Tracking by Semantic Attribute Parsing and Cross-Camera Tracklet Matching
CVPR Workshops(2020)
摘要
This paper focuses on the Multi-Target Multi-Camera Tracking (MTMCT) task in a city-scale multi-camera network. As the trajectory of each target is naturally split into multiple sub-trajectories (namely local tracklets) in different cameras, the key issue of MTMCT is how to match local tracklets belonging to the same target across different cameras. To this end, we propose an efficient two-step MTMCT approach to robustly track vehicles in a camera network. It first generates all local tracklets and then matches the ones belonging to the same target across different cameras. More specifically, in the local tracklet generation phase, we follow the tracking-by-detection paradigm and link the detections to local tracklets by graph clustering. In the cross-camera tracklet matching phase, we first develop a spatial-temporal attention mechanism to produce robust tracklet representations. We then prune false matching candidates by traffic topology reasoning and match tracklets across cameras using the recently proposed TRACklet-to-Target Assignment (TRACTA) algorithm. The proposed method is evaluated on the City-Scale Multi-Camera Vehicle Tracking task at the 2020 AI City Challenge and achieves the second-best results.
更多查看译文
关键词
multitarget multicamera tracking task,city-scale multicamera network,local tracklets,two-step MTMCT approach,track vehicles,local tracklet generation phase,tracking-by-detection paradigm,robust tracklet representations,false matching candidates,traffic topology reasoning,city-scale multicamera vehicle tracking task,TRACklet-to-target assignment algorithm,cross-camera tracklet matching,target trajectory,graph clustering,spatial-temporal attention mechanism,prune false matching,TRACTA algorithm
AI 理解论文
溯源树
样例
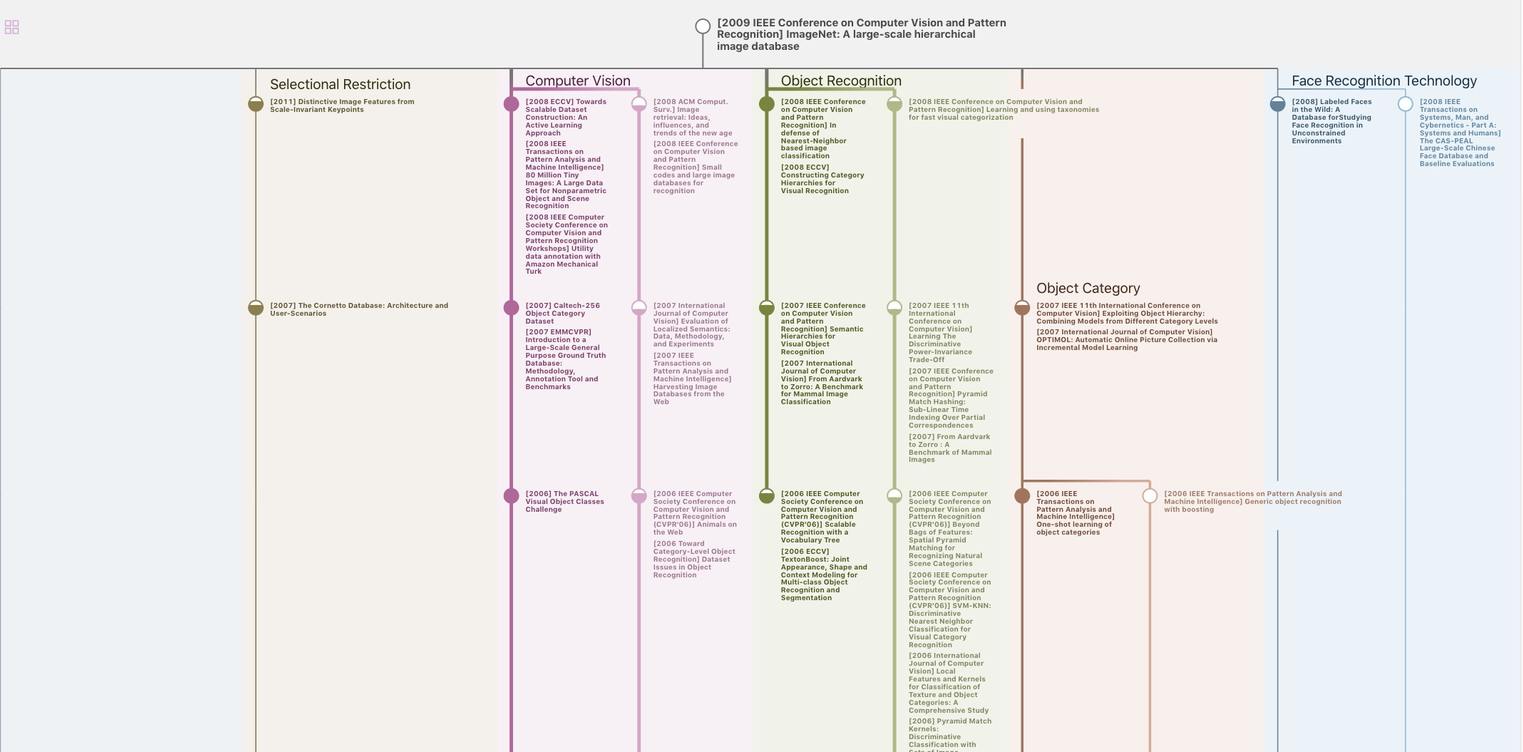
生成溯源树,研究论文发展脉络
Chat Paper
正在生成论文摘要