Machine-Learning-Enabled Exploration of Morphology Influence on Wire-Array Electrodes for Electrochemical Nitrogen Fixation.
The journal of physical chemistry letters(2020)
摘要
Neural networks, trained on data generated by a microkinetic model and finite-element simulations, expand explorable parameter space by significantly accelerating the predictions of electrocatalytic performance. In addition to modeling electrode reactivity, we use micro/nanowire arrays as a well-defined, easily tuned, and experimentally relevant exemplary morphology for electrochemical nitrogen fixation. This model system provides the data necessary for training neural networks which are subsequently exploited for electrocatalytic material morphology optimizations and explorations into the influence of geometry on nitrogen fixation electrodes, feats untenable without large-scale simulations, on both a global and a local basis.
更多查看译文
AI 理解论文
溯源树
样例
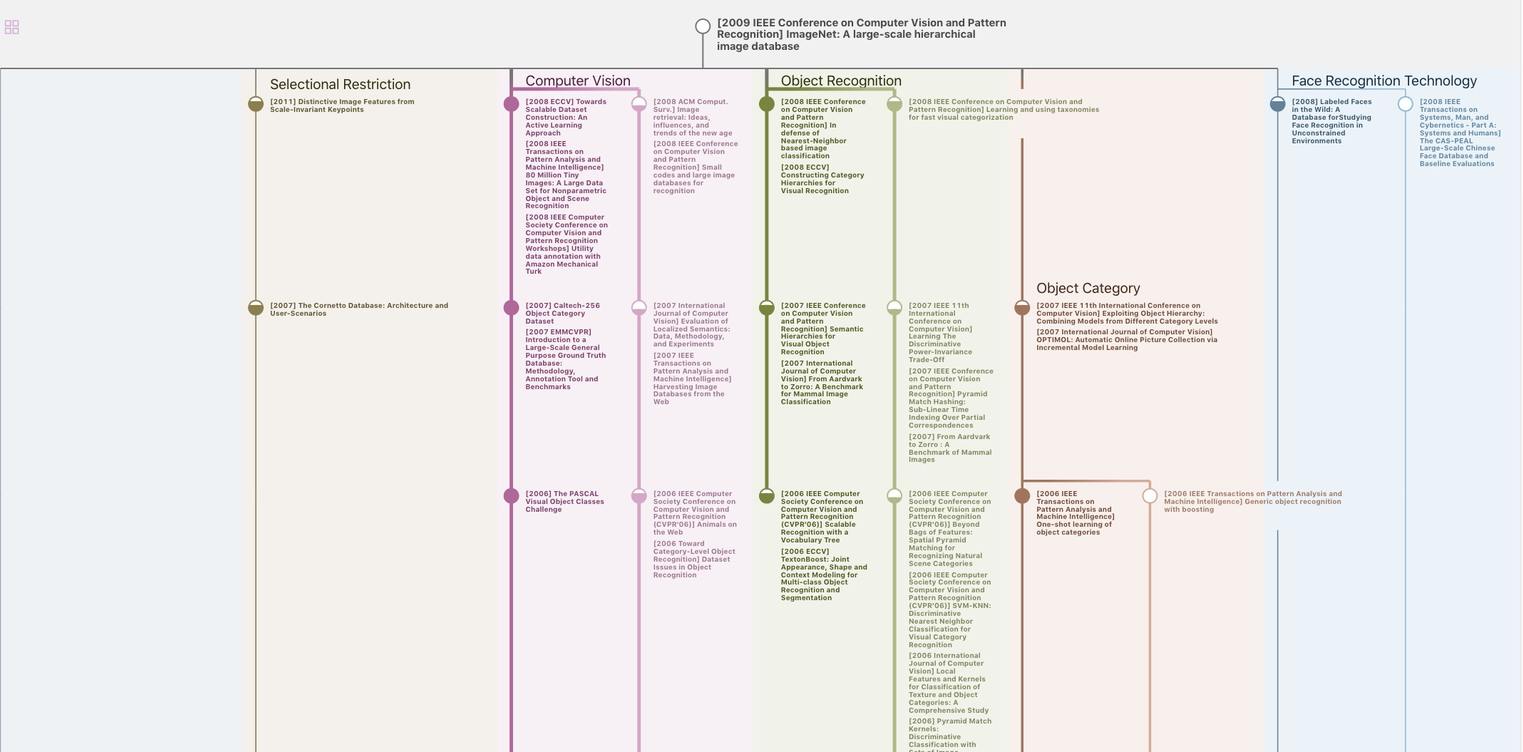
生成溯源树,研究论文发展脉络
Chat Paper
正在生成论文摘要