Robust Low Rate Speech Coding Based on Cloned Networks and Wavenet
ICASSP(2020)
摘要
Rapid advances in machine-learning based generative modeling of speech make its use in speech coding attractive. However, the current performance of such models drops rapidly with noise contamination of the input, preventing use in practical applications. We present a new speech-coding scheme that is based on features that are robust to the distortions occurring in speech-coder input signals. To this purpose, we encourage the feature encoder to provide the same independent features for each of a set of linguistically equivalent signals, obtained by adding various noises to a common clean signal. The independent features, subjected to scalar quantization, are used as a conditioning vector sequence for WaveNet. Our experiments show that a 1.8 kb/s implementation of the resulting coder provides state-of-the-art performance for clean signals, and is additionally robust to noisy input.
更多查看译文
关键词
robust low rate speech coding,cloned networks,machine-learning based generative modeling,noise contamination,speech-coder input signals,feature encoder,independent features,linguistically equivalent signals,clean signals,noisy input,wavenet networks,scalar quantization,conditioning vector sequence
AI 理解论文
溯源树
样例
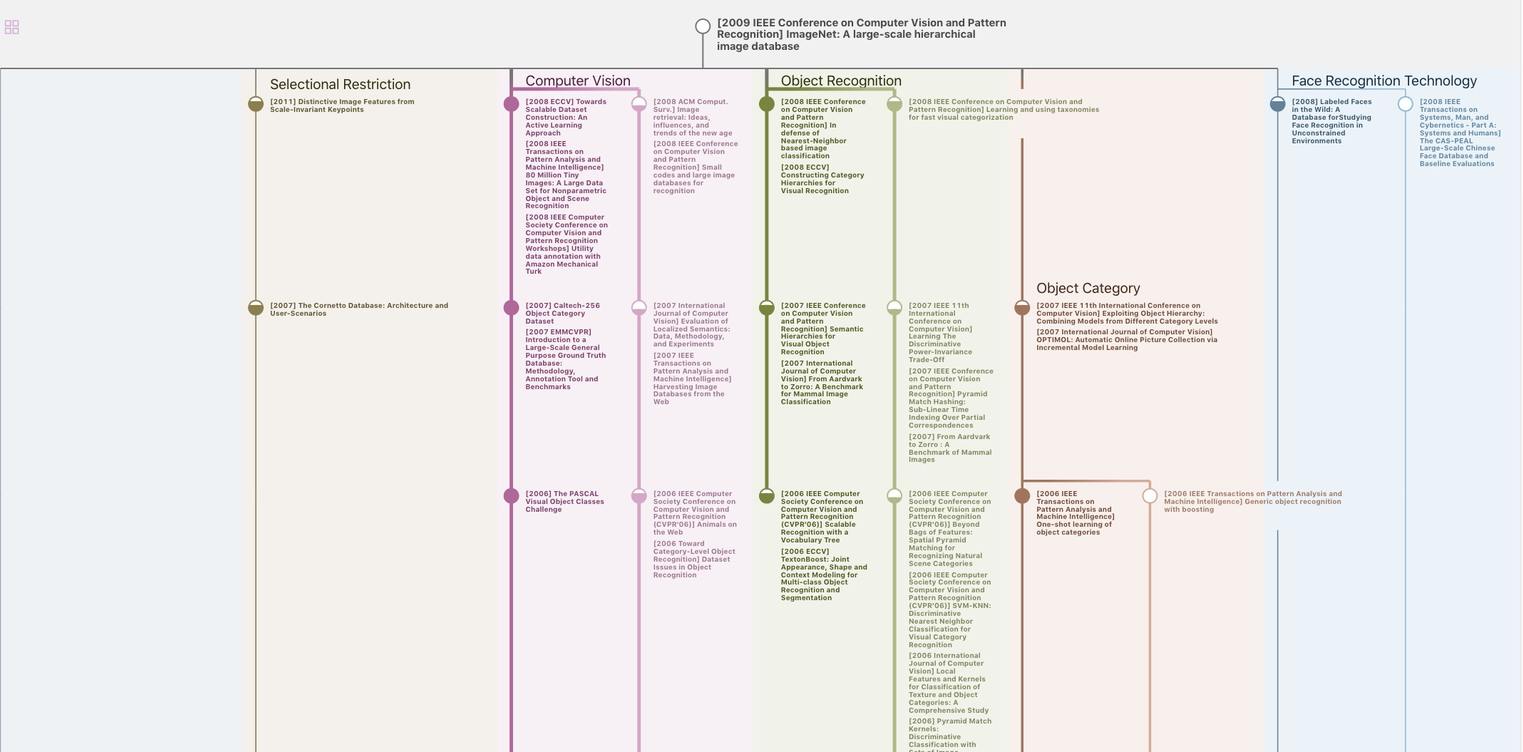
生成溯源树,研究论文发展脉络
Chat Paper
正在生成论文摘要