A Comparative Analysis of 3D User Interaction: How to Move Virtual Objects in Mixed Reality
IEEE Conference on Virtual Reality and 3D User Interfaces (VR)(2020)CCF A
Abstract
Using one’s hands can be a natural and intuitive method for interacting with 3D objects in a mixed reality environment. This study explores three hand-interaction techniques, including the gaze and pinch, touch and grab, and worlds-in-miniature interaction for selecting and moving virtual furniture in the 3D scene. Overall, a comparative analysis reveals that the worlds-in-miniature provided the best usability and task performance than other studied techniques. We also conducted in-depth interviews and analyzed participants’ hand gestures in order to identify desired attributes for 3D hand interaction design. Findings from interviews suggest that, when it comes to enjoyment and discoverability, users prefer directly manipulating the virtual furniture to interacting with objects remotely or using in-direct interactions such as gaze. Another insight this study provides is the critical roles of the virtual object’s visual appearance in designing natural hand interaction. Gesture analysis reveals that shapes of furniture, as well as its perceived features such as weight, largely determined the participant’s instinctive form of hand interaction (i.e., lift, grab, push). Based on these findings, we present design suggestions that can aid 3D interaction designers to develop a natural and intuitive hand interaction for mixed reality.
MoreTranslated text
Key words
Human-centered computing,Human computer interaction (HCI),Interaction paradigms,Virtual reality,Human-centered computing,Human computer interaction (HCI),HCI design and evaluation methods,User studies
PDF
View via Publisher
AI Read Science
AI Summary
AI Summary is the key point extracted automatically understanding the full text of the paper, including the background, methods, results, conclusions, icons and other key content, so that you can get the outline of the paper at a glance.
Example
Background
Key content
Introduction
Methods
Results
Related work
Fund
Key content
- Pretraining has recently greatly promoted the development of natural language processing (NLP)
- We show that M6 outperforms the baselines in multimodal downstream tasks, and the large M6 with 10 parameters can reach a better performance
- We propose a method called M6 that is able to process information of multiple modalities and perform both single-modal and cross-modal understanding and generation
- The model is scaled to large model with 10 billion parameters with sophisticated deployment, and the 10 -parameter M6-large is the largest pretrained model in Chinese
- Experimental results show that our proposed M6 outperforms the baseline in a number of downstream tasks concerning both single modality and multiple modalities We will continue the pretraining of extremely large models by increasing data to explore the limit of its performance
Try using models to generate summary,it takes about 60s
Must-Reading Tree
Example
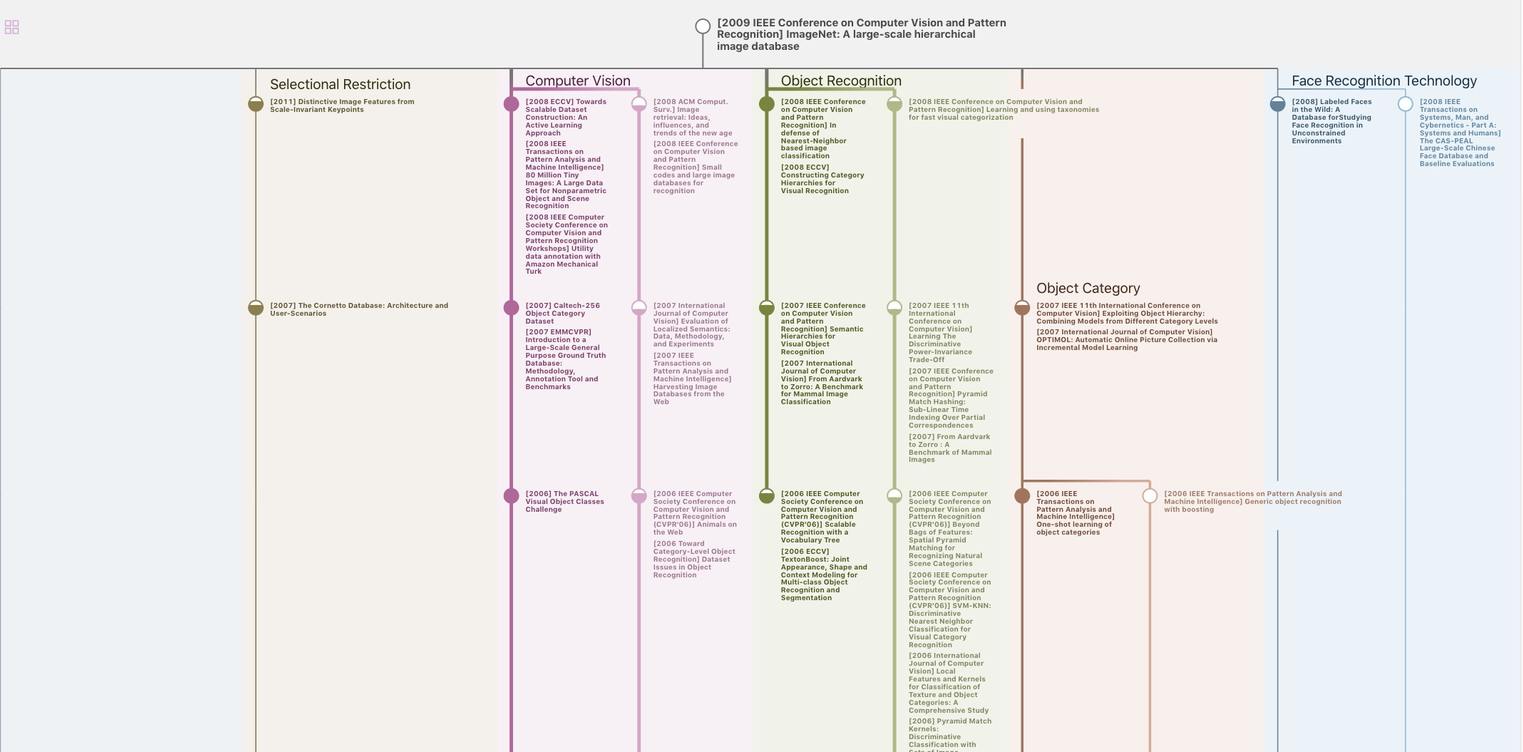
Generate MRT to find the research sequence of this paper
Related Papers
2002
被引用562 | 浏览
2010
被引用54 | 浏览
2011
被引用115 | 浏览
1988
被引用18392 | 浏览
2015
被引用115 | 浏览
2014
被引用126 | 浏览
2016
被引用31 | 浏览
2016
被引用29 | 浏览
Data Disclaimer
The page data are from open Internet sources, cooperative publishers and automatic analysis results through AI technology. We do not make any commitments and guarantees for the validity, accuracy, correctness, reliability, completeness and timeliness of the page data. If you have any questions, please contact us by email: report@aminer.cn
Chat Paper
Summary is being generated by the instructions you defined