Controlling Overestimation Bias with Truncated Mixture of Continuous Distributional Quantile Critics
INTERNATIONAL CONFERENCE ON MACHINE LEARNING, VOL 119(2020)
摘要
The overestimation bias is one of the major impediments to accurate off-policy learning. This paper investigates a novel way to alleviate the overestimation bias in a continuous control setting. Our method-Truncated Quantile Critics, TQC,-blends three ideas: distributional representation of a critic, truncation of critics prediction, and ensembling of multiple critics. Distributional representation and truncation allow for arbitrary granular overestimation control, while ensembling provides additional score improvements. TQC outperforms the current state of the art on all environments from the continuous control benchmark suite, demonstrating 25% improvement on the most challenging Humanoid environment.
更多查看译文
AI 理解论文
溯源树
样例
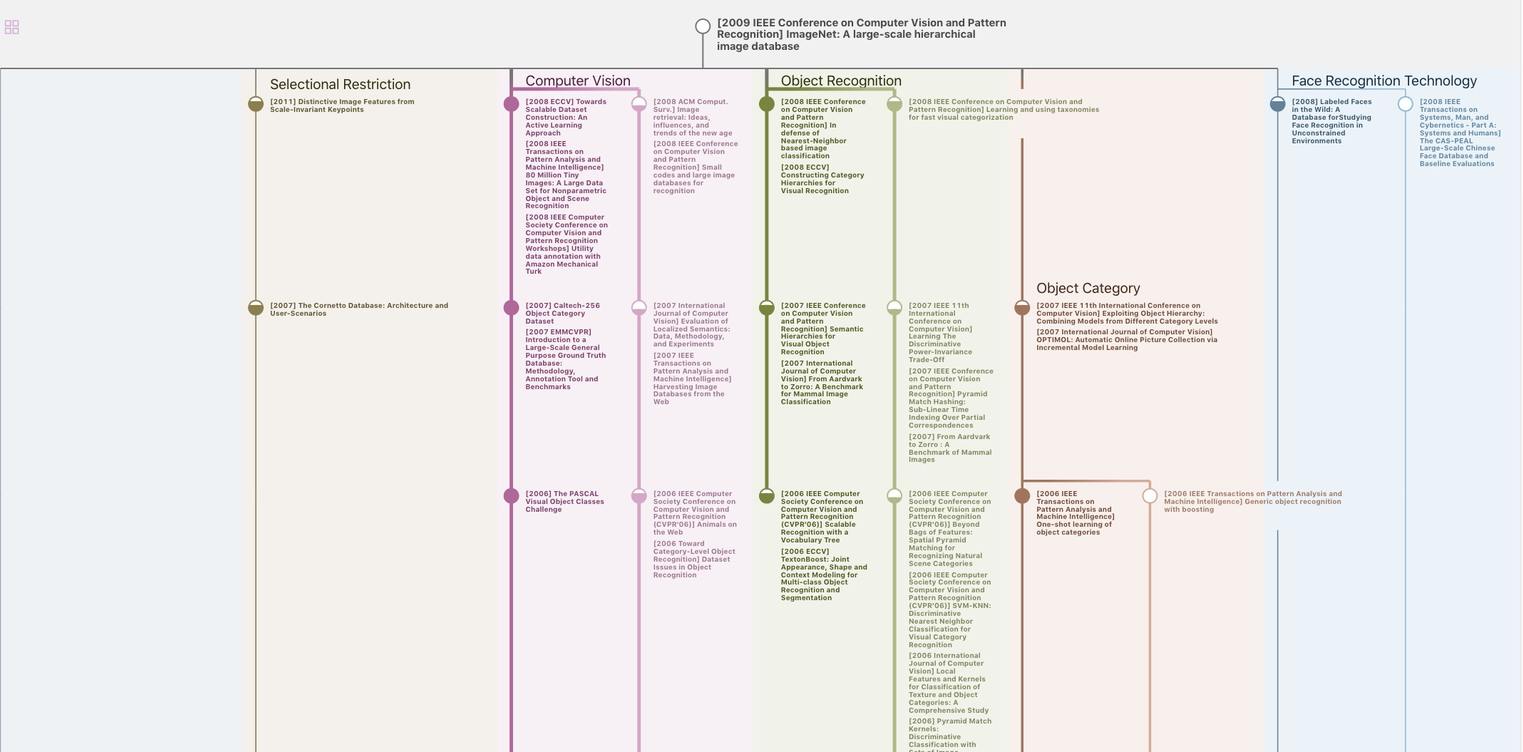
生成溯源树,研究论文发展脉络
Chat Paper
正在生成论文摘要