Estimating Time to Progression of Chronic Obstructive Pulmonary Disease With Tolerance
IEEE Journal of Biomedical and Health Informatics(2021)
摘要
We defined tolerance range as the distance of observing similar disease conditions or functional status from the upper to the lower boundaries of a specified time interval. A tolerance range was identified for linear regression and support vector machines to optimize the improvement rate (defined as IR) on accuracy in predicting mortality risk in patients with chronic obstructive pulmonary disease using clinical notes. The corpus includes pulmonary, cardiology, and radiology reports of 15,500 patients who died between 2011 and 2017. Their performance was compared against a long short-term memory recurrent neural network. The results demonstrate an overall improvement by those basic machine learning approaches after considering an optimal tolerance range: the average IR of linear regression was 90.1% and the maximum IR of support vector machines was 66.2%. There was a similitude between the time segments produced by our tolerance algorithms and those produced by the long short-term memory.
更多查看译文
关键词
Algorithms,Humans,Machine Learning,Neural Networks, Computer,Pulmonary Disease, Chronic Obstructive,Support Vector Machine
AI 理解论文
溯源树
样例
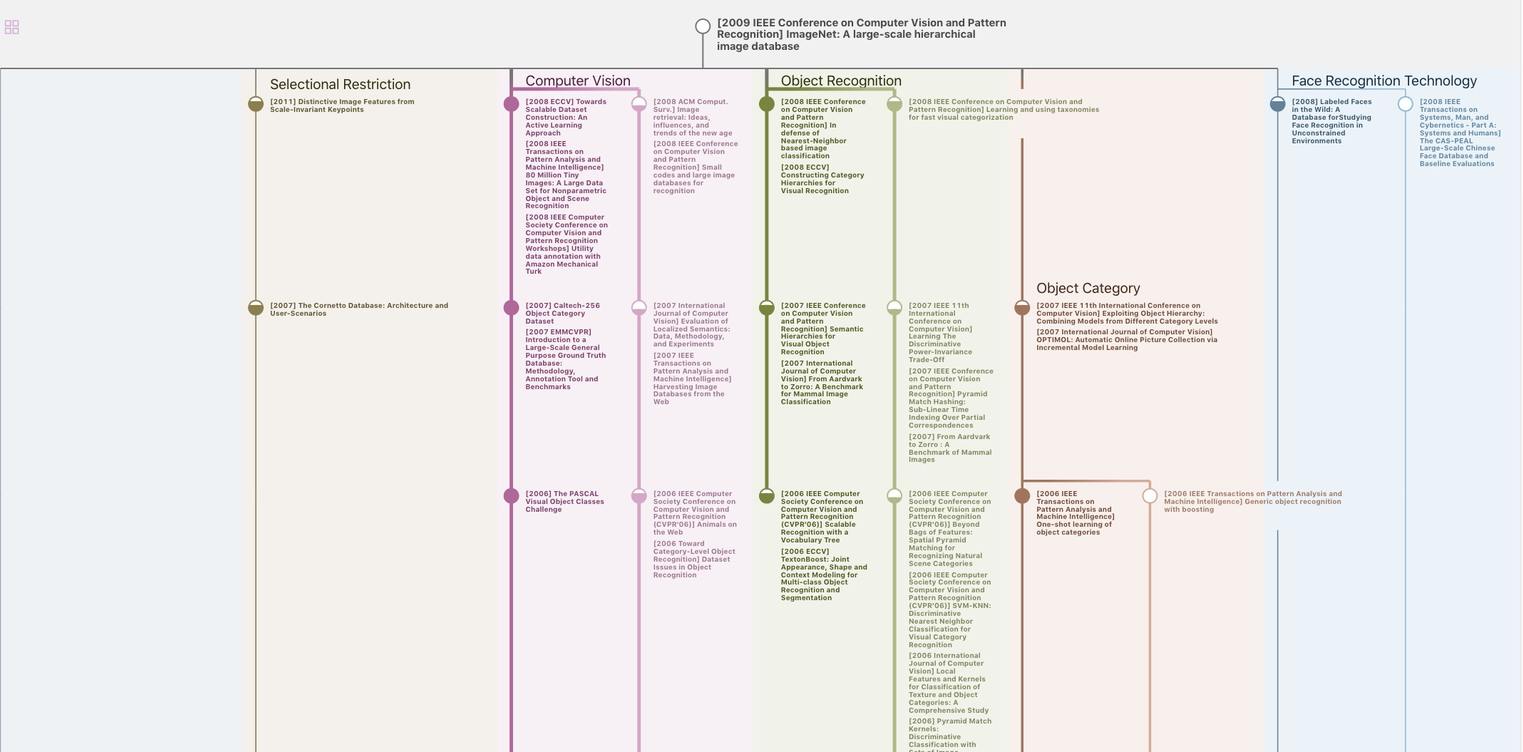
生成溯源树,研究论文发展脉络
Chat Paper
正在生成论文摘要