The Impact Of Smote And Grid Search On Maintainability Prediction Models
2019 IEEE/ACS 16TH INTERNATIONAL CONFERENCE ON COMPUTER SYSTEMS AND APPLICATIONS (AICCSA 2019)(2019)
摘要
Software maintainability has gained more attention in recent years. It can be defined as the ease with which modifications can be performed. In this study, we are interested in the changes of a class due to bug fixing. We performed an experimental study using five Machine learning techniques; K-Nearest Neighbor (KNN), Support Vector Machine (SVM), Decision Trees (DT), Multilayer Perceptron (MLP), and Naive Bayes (NB). The main focus in this study is to propose the use of Grid search method for tuning hyper-parameters and to balance datasets using SMOTE technique. The results show that there is no evidence concerning the best ML technique in all datasets. However, we found that balanced data and tuning parameters are suitable in order to obtain the best performance of ML techniques.
更多查看译文
关键词
Maintainability,Prediction,Bug,Tuning parameters,Smote,Grid search,Balanced data
AI 理解论文
溯源树
样例
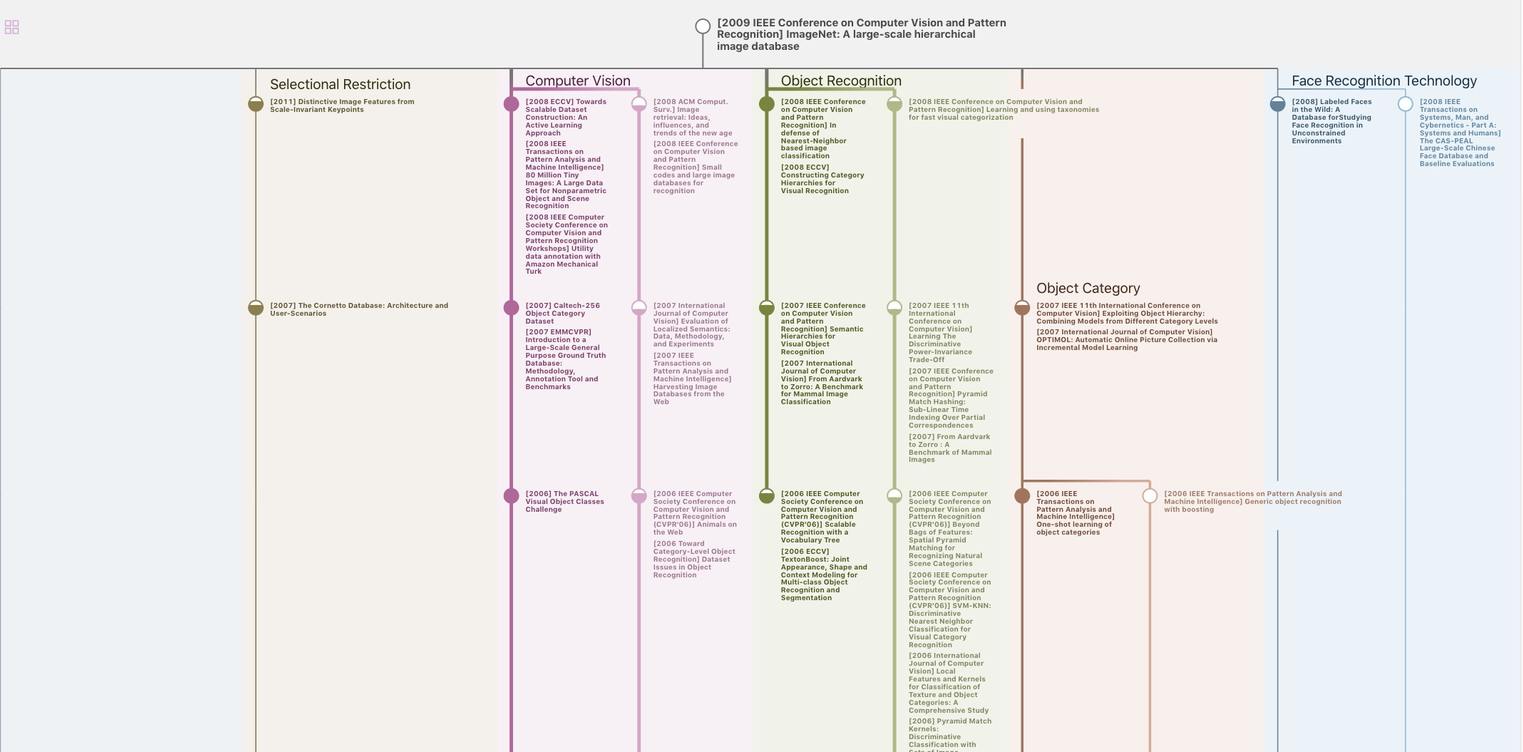
生成溯源树,研究论文发展脉络
Chat Paper
正在生成论文摘要