Human activity classification based on sound recognition and residual convolutional neural network
Automation in Construction(2020)
摘要
Human activity recognition is crucial for a better understanding of workers in construction sites and people in the built environment. Previous studies have been proposed various ways in which sensing and machine learning techniques can be utilized to collect human activity data automatically. Sound recognition has the potential to be utilized in ways that complement the limitations of the previous methods because sound signals are easy to propagate in indoor environments where many physical obstacles exist, and this method can simultaneously recognize not only sounds from human activities but also sounds from related objects. Therefore, this study develops a sound recognition-based human activity classification model using a residual neural network. A sound data is collected based on ten classes representing people's daily activities in the indoor environment. Then, the features of the sound data were extracted using the Log Mel-filter bank energies method, and a residual neural network model with 34 convolutional layers was trained using the data. The results showed the following: the accuracy of the model was 87.6%, and the Precision score for each class ranged from 76.8% to 92.6%, the Recall scores ranged from 75.8% to 98.6%, and the F1-score ranged from 78.6% to 93.7%. The contribution of this study is to demonstrate that sound recognition can classify people's indoor activities successfully, but this study leaves the limitation that it is based on a monophonic method that only one activity can be classified at a time.
更多查看译文
关键词
Human activity recognition,Sound recognition,Residual neural network,Convolutional neural network
AI 理解论文
溯源树
样例
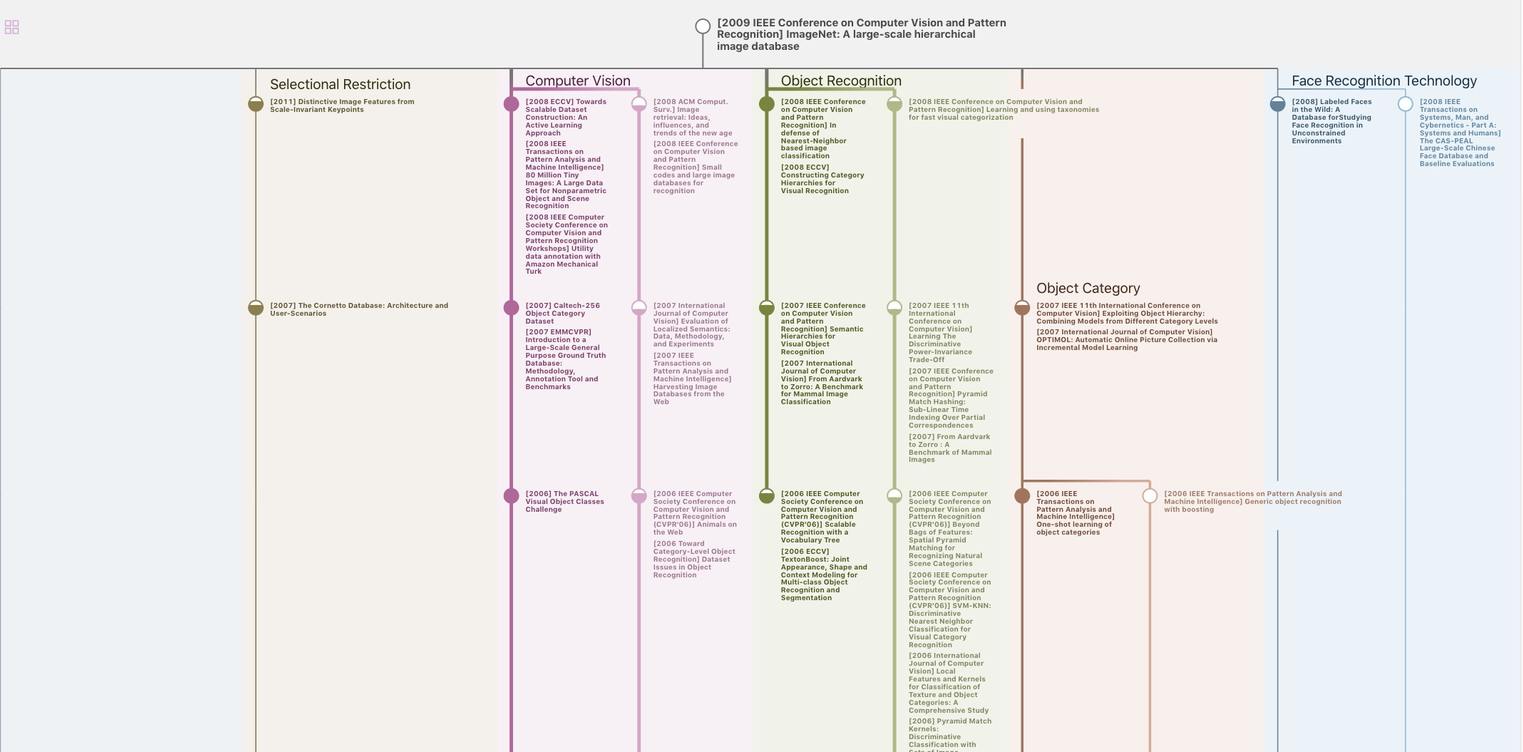
生成溯源树,研究论文发展脉络
Chat Paper
正在生成论文摘要