Linking the Evolution of Catalytic Properties and Structural Changes in Copper-Zinc Nanocatalysts using Operando EXAFS and Neural-Networks
CHEMICAL SCIENCE(2020)
摘要
Understanding the evolution of unique structural motifs in bimetallic catalysts under reaction conditions, and linking them to the observed catalytic properties is necessary for the rational design of the next generation of catalytic materials. Extended X-ray absorption fine structure (EXAFS) spectroscopy is a premier experimental method to address this issue, providing the possibility to track the changes in the structure of working catalysts. Unfortunately, the intrinsic heterogeneity and enhanced disorder characteristic of catalytic materials experiencing structural transformations under reaction conditions, as well as the low signal-to-noise ratio that is common for in situ EXAFS spectra hinder the application of conventional data analysis approaches. Here we address this problem by employing machine learning methods (artificial neural networks) to establish the relationship between EXAFS features and structural motifs in metals as well as oxide materials. We apply this approach to time-dependent EXAFS spectra acquired from copper-zinc nanoparticles during the electrochemical reduction of CO2 to reveal the details of the composition-dependent structural evolution and brass alloy formation, and their correlation with the catalytic selectivity of these materials.
更多查看译文
关键词
copper–zinc nanocatalysts,catalytic properties,neural-networks
AI 理解论文
溯源树
样例
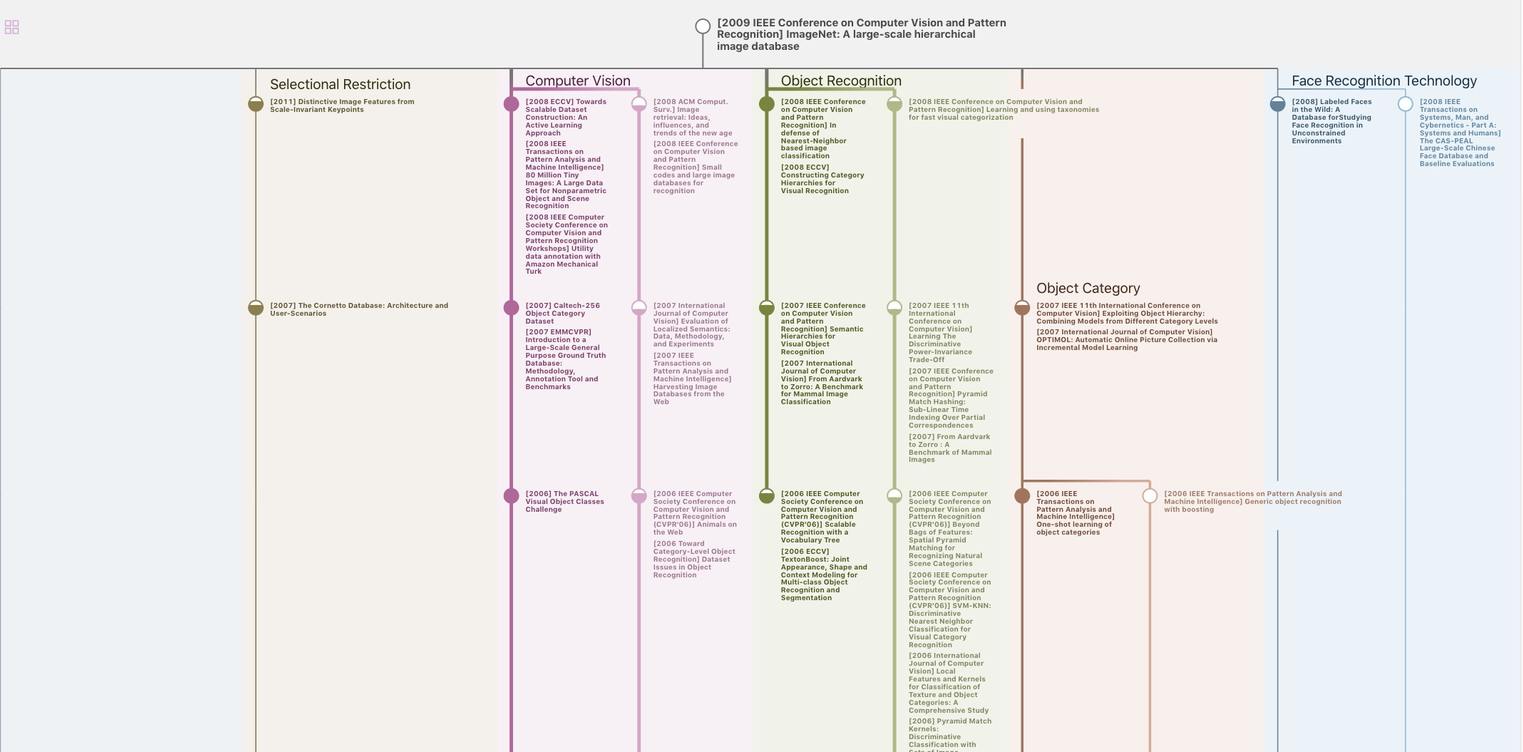
生成溯源树,研究论文发展脉络
Chat Paper
正在生成论文摘要