Learning-based Coordination Model for Spontaneous Self-Composition of Reliable Services in a Distributed System
International journal on software tools for technology transfer(2020)
摘要
Context-aware, pervasive systems, mobile devices, intelligent virtual assistants activating services or controlling connected devices are pervading our everyday life. These systems rely on centralized services provided by servers in a cloud gathering all requests, performing pre-defined computations and involving pre-defined devices. Large-scale scenarios, involving unanticipated devices, adaptation to dynamically changing conditions, call for alternative solutions favoring edge computing and decentralized behavior. For several years, we have worked on a new type of applications, built and spontaneously composed on-demand. Applications arise from the interactions of multiple sensors and devices, working together as a decentralized collective adaptive system. Our solution relies on a learning-based coordination model providing decentralized communication platforms among agents working on behalf of heterogeneous devices. Each device provides few simple services and data regarding itself (properties and capabilities). In this article, we discuss first the design of complex services, arising from the spontaneous self-composition of simpler services. Second, we present our learning-based coordination model combining coordination and reinforcement learning, and how this approach ensures reliable self-composition of services in terms of functionality and expected quality of services. On the basis of a humanitarian scenario, we show the feasibility of the approach and discuss our current implementation. Preliminary results show convergence toward learning and correct functionality. Spontaneous self-composition and learning provide a self-adaptive solution for creating on-demand complex services evolving in highly dynamic scenarios comprising large numbers of connected devices.
更多查看译文
关键词
Coordination model,Collective adaptive system,On-demand services,Multi-agent learning,Reinforcement learning,QLearning
AI 理解论文
溯源树
样例
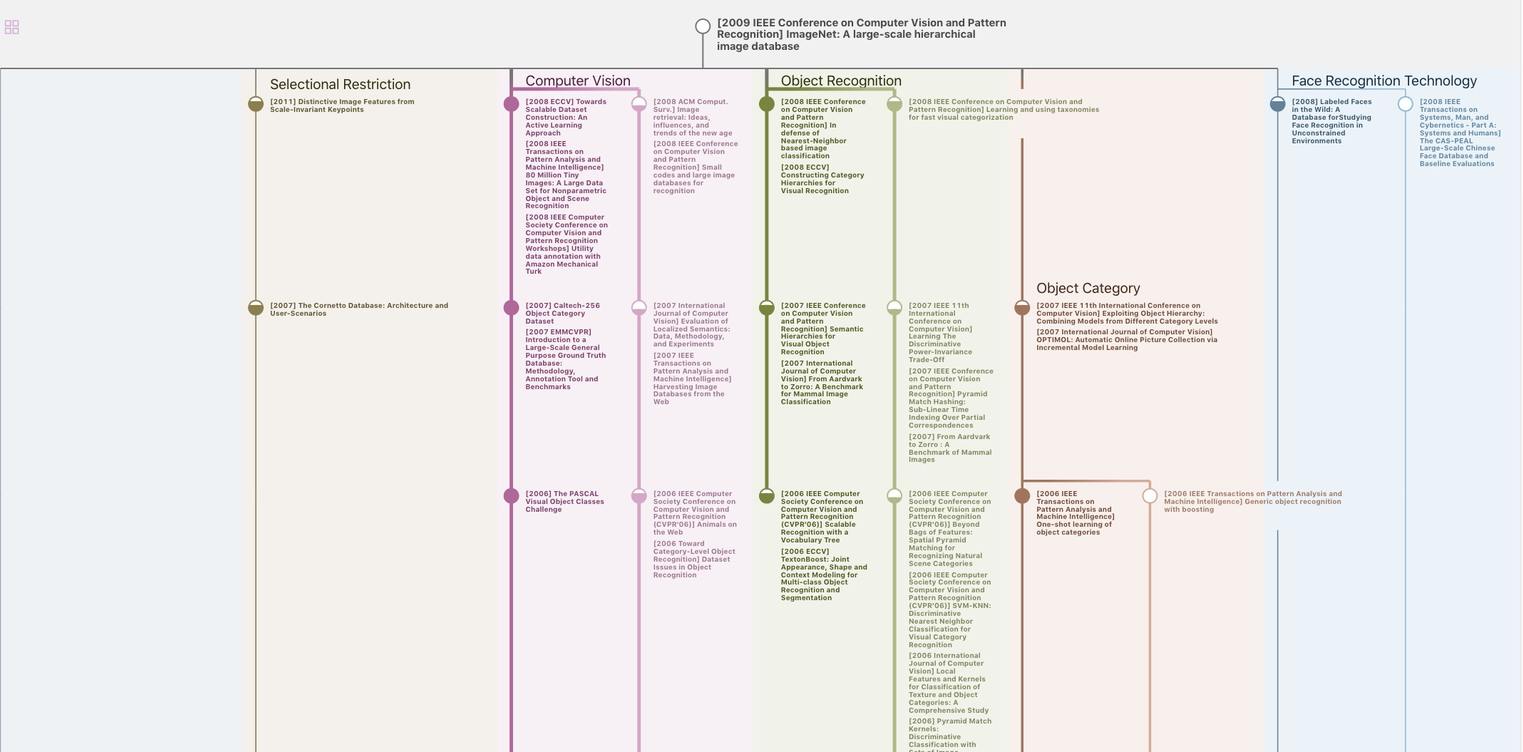
生成溯源树,研究论文发展脉络
Chat Paper
正在生成论文摘要