DAMER: a novel diagnosis aggregation method with evidential reasoning rule for bearing fault diagnosis
JOURNAL OF INTELLIGENT MANUFACTURING(2020)
摘要
Ensemble learning method has shown its superiority in bearing fault diagnosis based on the condition based monitoring. Nevertheless, features extracted from the monitoring signals of bearing systems often contain interrelated and redundant components, leading to poor performances of the base classifiers in the ensemble. Moreover, the current ensemble methods rely on voting strategies to aggregate the diagnostic predictions of these base classifiers without considering their reliabilities and weights simultaneously. To address the aforementioned issues, we propose a novel Diagnosis Aggregation Method with Evidential Reasoning rule, i.e., DAMER, for bearing fault diagnosis. In this method, a semi-random subspace approach using a structured sparsity learning model is developed to decrease the negative effect of interrelated and redundant features, and in the meanwhile to generate accurate and diverse base classifiers. Furthermore, an adaptive evidential reasoning rule (ER rule) incorporating with ensemble learning theory is utilized to aggregate the diagnostic predictions of the base classifiers by taking both their weights and reliabilities into account. To validate the proposed DAMER, an empirical study is conducted on Case Western Reserve University bearing vibration dataset, and the experimental results verify the effectiveness of the proposed DAMER as well as its superiority over commonly used ensemble methods. The performances of feature subsets from multiple domains and the aggregation capability of the adaptive ER rule were also investigated. Results illustrate that DAMER can be utilized as an effective method for bearing fault diagnosis.
更多查看译文
关键词
Bearing fault diagnosis,Condition based monitoring,Ensemble learning,Structured sparsity learning,Evidential reasoning rule
AI 理解论文
溯源树
样例
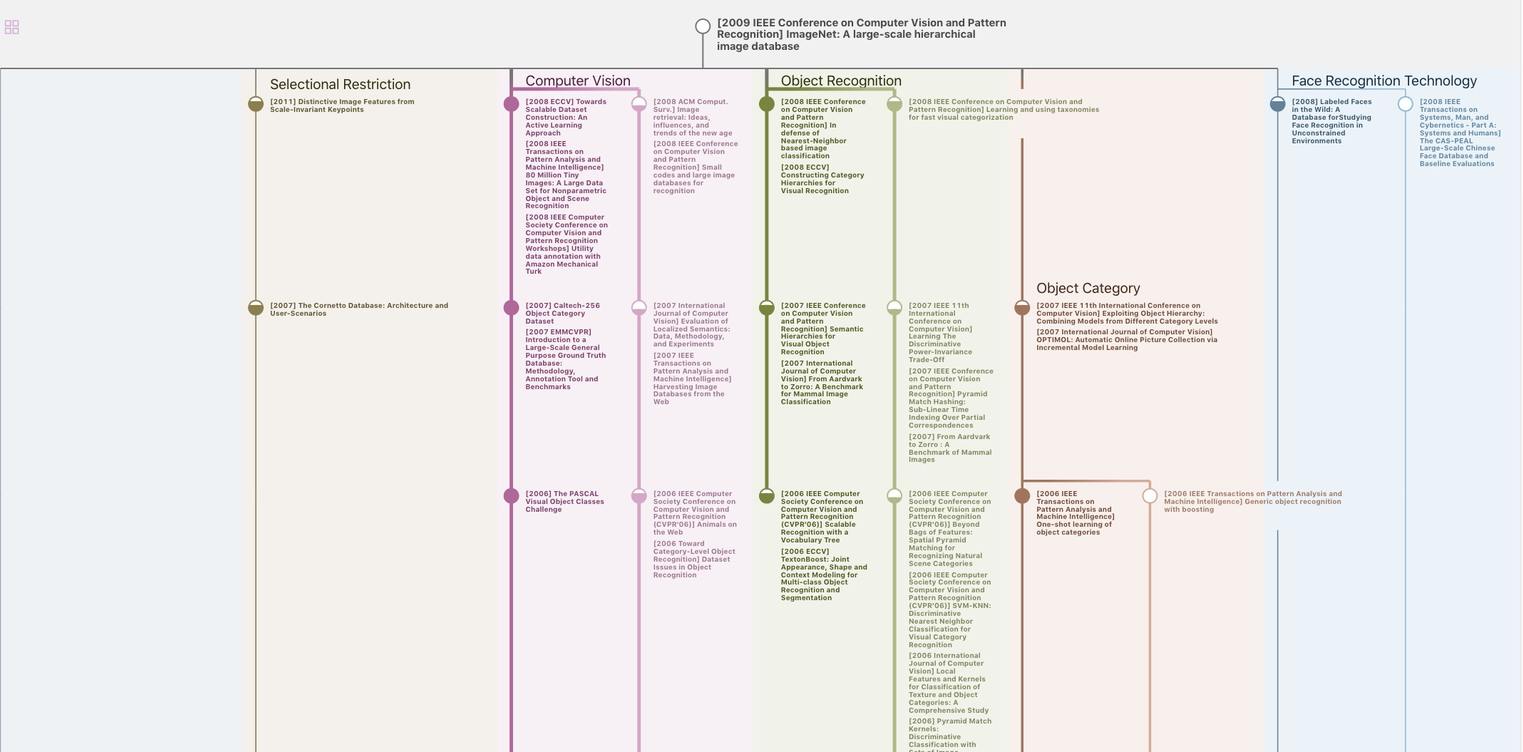
生成溯源树,研究论文发展脉络
Chat Paper
正在生成论文摘要