Quantum Approximate Optimization of Non-Planar Graph Problems on a Planar Superconducting Processor
Nature physics(2021)
摘要
We demonstrate the application of the Google Sycamore superconducting qubitquantum processor to combinatorial optimization problems with the quantumapproximate optimization algorithm (QAOA). Like past QAOA experiments, we studyperformance for problems defined on the (planar) connectivity graph of ourhardware; however, we also apply the QAOA to the Sherrington-Kirkpatrick modeland MaxCut, both high dimensional graph problems for which the QAOA requiressignificant compilation. Experimental scans of the QAOA energy landscape showgood agreement with theory across even the largest instances studied (23qubits) and we are able to perform variational optimization successfully. Forproblems defined on our hardware graph we obtain an approximation ratio that isindependent of problem size and observe, for the first time, that performanceincreases with circuit depth. For problems requiring compilation, performancedecreases with problem size but still provides an advantage over randomguessing for circuits involving several thousand gates. This behaviorhighlights the challenge of using near-term quantum computers to optimizeproblems on graphs differing from hardware connectivity. As these graphs aremore representative of real world instances, our results advocate for moreemphasis on such problems in the developing tradition of using the QAOA as aholistic, device-level benchmark of quantum processors.
更多查看译文
关键词
Computational science,Quantum information,Physics,general,Theoretical,Mathematical and Computational Physics,Classical and Continuum Physics,Atomic,Molecular,Optical and Plasma Physics,Condensed Matter Physics,Complex Systems
AI 理解论文
溯源树
样例
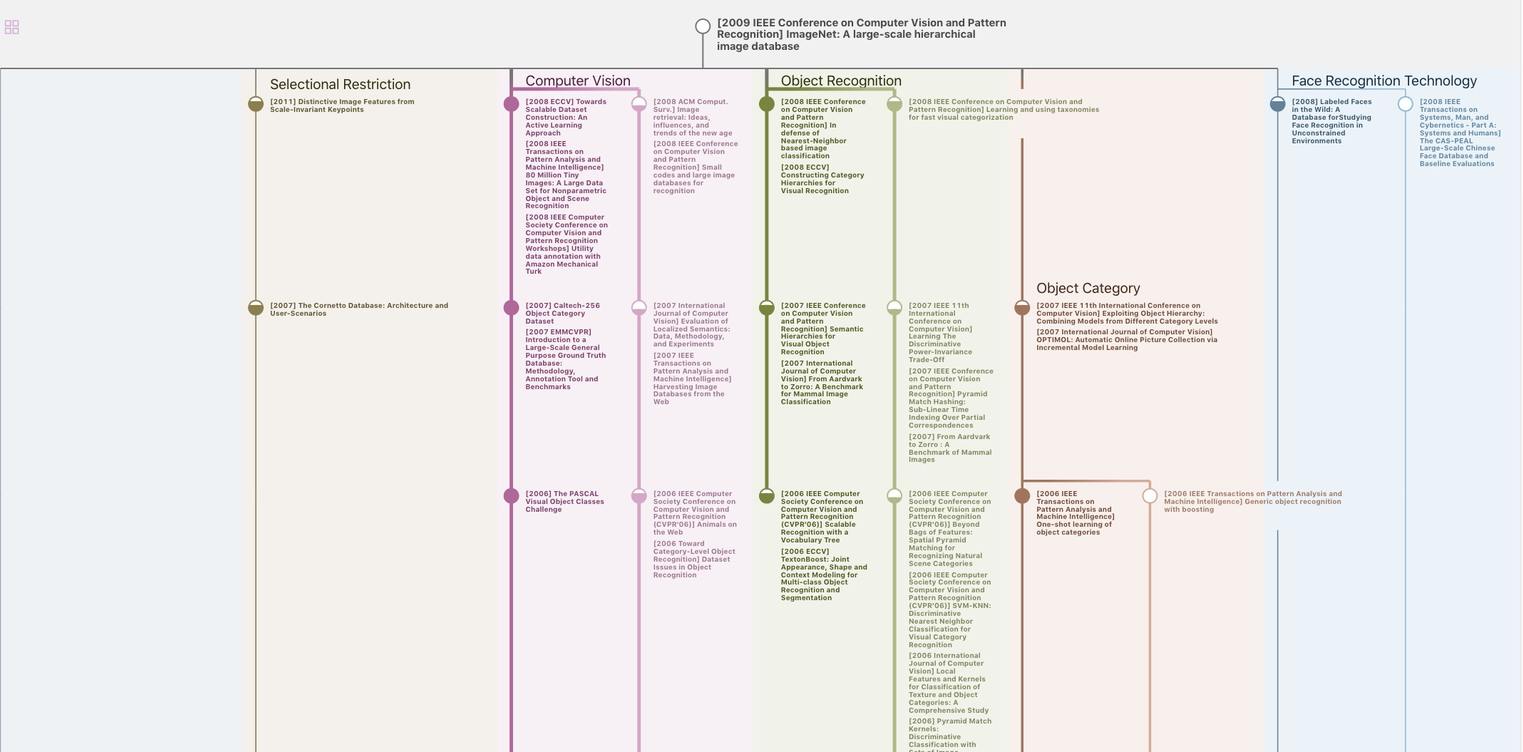
生成溯源树,研究论文发展脉络
Chat Paper
正在生成论文摘要