Oil Spill Detection In Quad-Polarimetric Sar Images Using An Advanced Convolutional Neural Network Based On Superpixel Model
REMOTE SENSING(2020)
Abstract
Oil spill detection plays an important role in marine environment protection. Quad-polarimetric Synthetic Aperture Radar (SAR) has been proved to have great potential for this task, and different SAR polarimetric features have the advantages to recognize oil spill areas from other look-alikes. In this paper we proposed an oil spill detection method based on convolutional neural network (CNN) and Simple Linear Iterative Clustering (SLIC) superpixel. Experiments were conducted on three Single Look Complex (SLC) quad-polarimetric SAR images obtained by Radarsat-2 and Spaceborne Imaging Radar-C/X-Band Synthetic Aperture Radar (SIR-C/X-SAR). Several groups of polarized parameters, including H/A/Alpha decomposition, Single-Bounce Eigenvalue Relative Difference (SERD), correlation coefficients, conformity coefficients, Freeman 3-component decomposition, Yamaguchi 4-component decomposition were extracted as feature sets. Among all considered polarimetric features, Yamaguchi parameters achieved the highest performance with total Mean Intersection over Union (MIoU) of 90.5%. It is proved that the SLIC superpixel method significantly improved the oil spill classification accuracy on all the polarimetric feature sets. The classification accuracy of all kinds of targets types were improved, and the largest increase on mean MIoU of all features sets was on emulsions by 21.9%.
MoreTranslated text
Key words
oil spill,Synthetic Aperture Radar,polarimetric decomposition,superpixel,convolutional neural networks
AI Read Science
Must-Reading Tree
Example
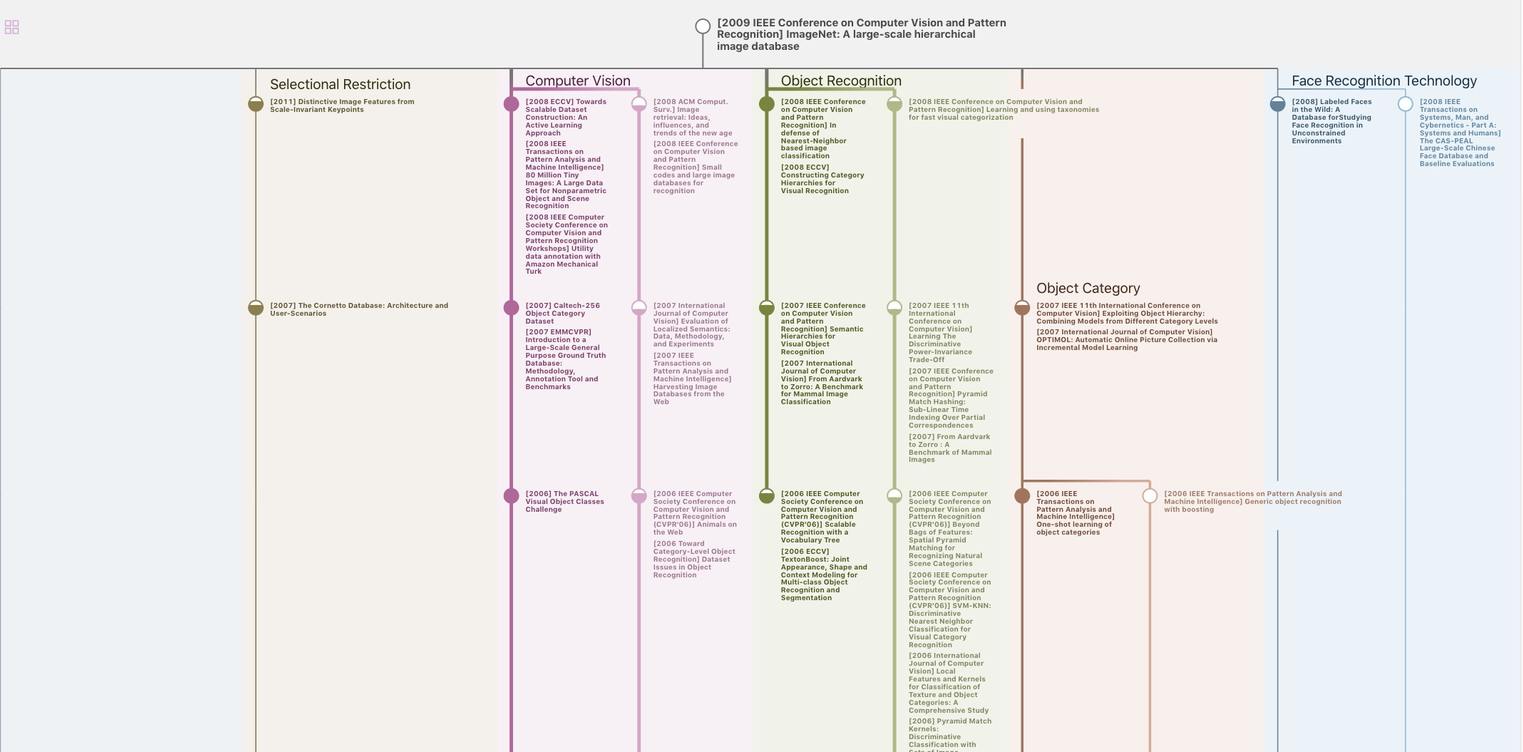
Generate MRT to find the research sequence of this paper
Chat Paper
Summary is being generated by the instructions you defined