Partial Least Squares for Sparsely Observed Curves with Measurement Errors
arxiv(2020)
摘要
Functional partial least squares (FPLS) is commonly used for fittingscalar-on-function regression models. For the sake of accuracy, FPLS demandsthat each realization of the functional predictor is recorded as densely aspossible over the entire time span; however, this condition is sometimesviolated in, e.g., longitudinal studies and missing data research. Targetingthis point, we adapt FPLS to scenarios in which the number of measurements persubject is small and bounded from above. The resulting proposal is abbreviatedas PLEASS. Under certain regularity conditions, we establish the consistency ofestimators and give confidence intervals for scalar responses. Simulationstudies and real-data applications illustrate the competitive accuracy ofPLEASS
更多查看译文
AI 理解论文
溯源树
样例
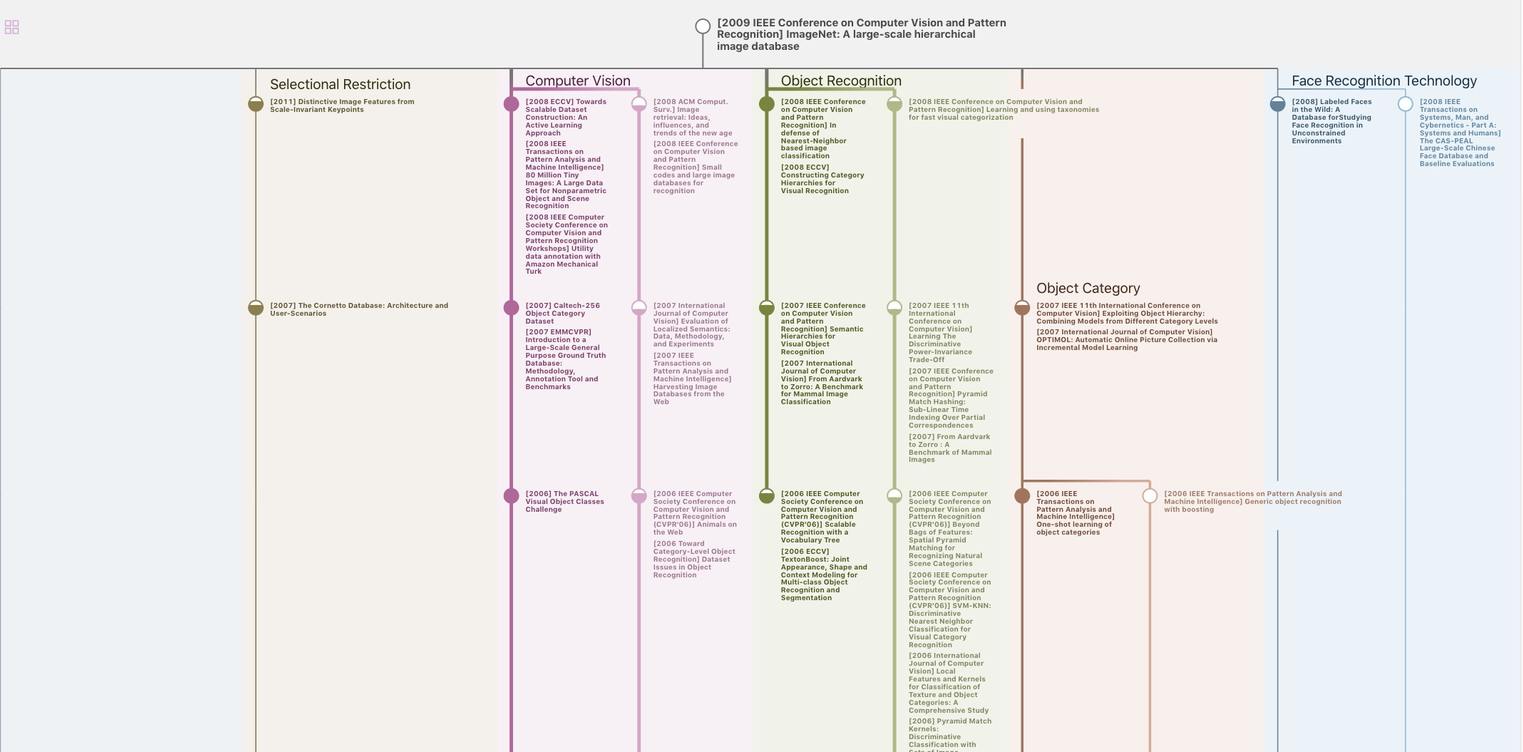
生成溯源树,研究论文发展脉络
Chat Paper
正在生成论文摘要