Robust support vector data description for novelty detection with contaminated data
Engineering Applications of Artificial Intelligence(2020)
摘要
Support vector data description (SVDD) is a widely used novelty detection algorithm. It provides excellent predictions even in the absence of negative samples and retains the mathematical elegance of Support Vector Machines. The decision boundary can be very flexible due to the incorporation of kernel functions. However, SVDD can suffer a lot from contaminated data containing, for example, outliers or mislabeled observations. Although several weighting schemes have been proposed to find a more reliable description of the target data, the calculation of the weight are themselves affected by the outliers and does not provide much insight in the data. The masked outliers fail to receive lower weight values. The Stahel–Donoho (SD) outlyingness from multivariate statistics is a very robust measure to expose the outliers. To avoid the masking effect, we propose to assign weight to each observation based on the SD outlyingness in an arbitrary kernel space. A robust SVDD is defined down-weighting the samples with large outlyingness. The experimental results demonstrate superiority of the proposed method in terms of AUC for contaminated data.
更多查看译文
关键词
Outlier detection,Support vector data description,Stahel–Donoho outlyingness,Robustness
AI 理解论文
溯源树
样例
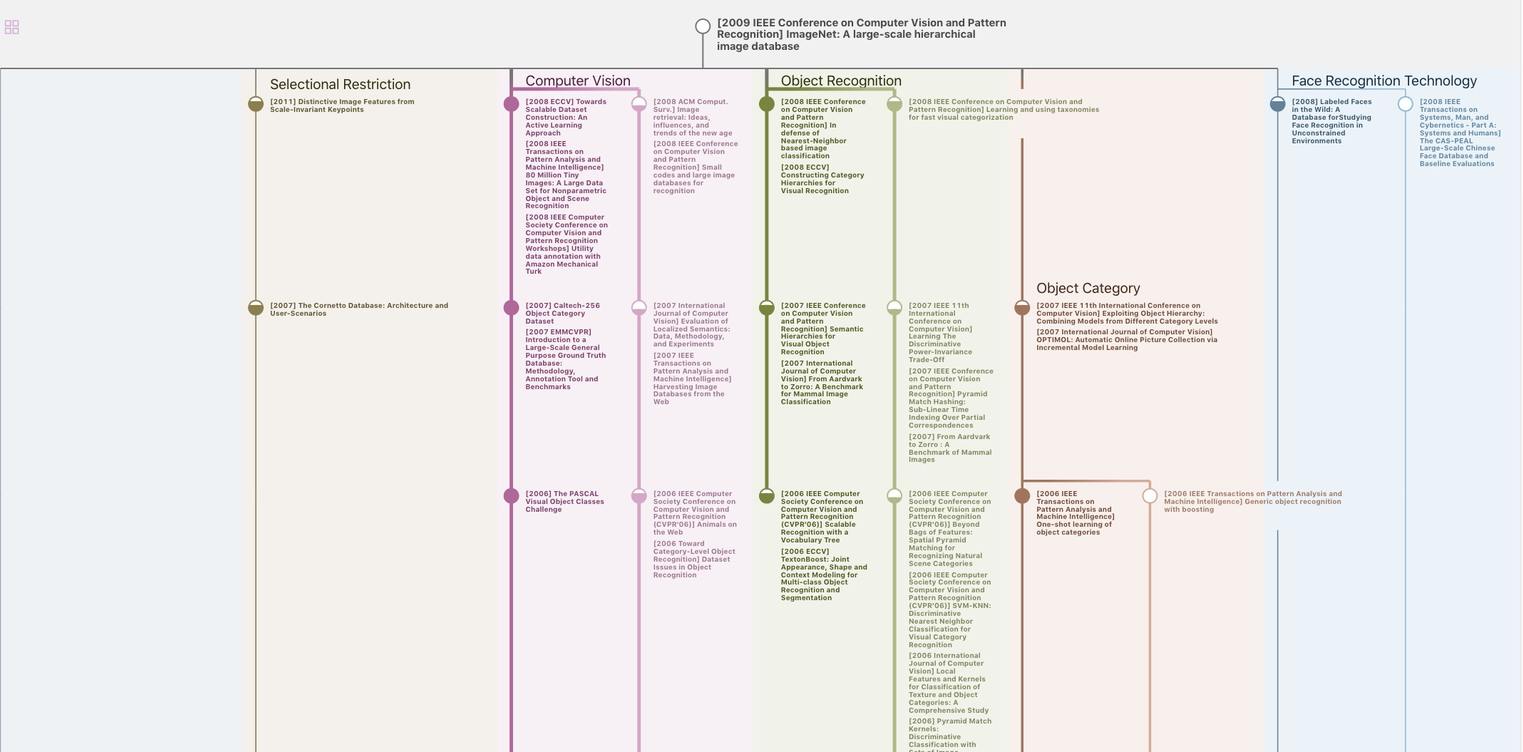
生成溯源树,研究论文发展脉络
Chat Paper
正在生成论文摘要