Comparison of Short-Term Streamflow Forecasting using Stochastic Time Series, Neural Networks, Process-Based, and Bayesian Models
Environmental Modelling & Software(2020)
摘要
Streamflow forecasts are essential for water resources management. Although there are many methods for forecasting streamflow, real-time forecasts remain challenging. This study evaluates streamflow forecasts using a process-based model (Soil and Water Assessment Tool-Variable Source Area model-SWAT-VSA), a stochastic model (Artificial Neural Network -ANN), an Auto-Regressive Moving-Average (ARMA) model, and a Bayesian ensemble model that utilizes the SWAT-VSA, ANN, and ARMA results. Streamflow is forecast from 1 to 8 d, forced with Quantitative Precipitation Forecasts from the US National Weather Service. Of the individual models, SWAT-VSA and the ANN provide better predictions of total streamflow (NSE 0.60–0.70) and peak flow, but underpredicted low flows. During the forecast period the ANN had the highest predictive power (NSE 0.44–0.64), however all three models underpredicted peak flow. The Bayesian ensemble forecast streamflow with the most skill for all forecast lead times (NSE 0.49–0.67) and provided a quantification of prediction uncertainty.
更多查看译文
关键词
SWAT-VSA,ANNs,ARMA,Forecasting,Stochastic model,Process-based model,Bayesian model
AI 理解论文
溯源树
样例
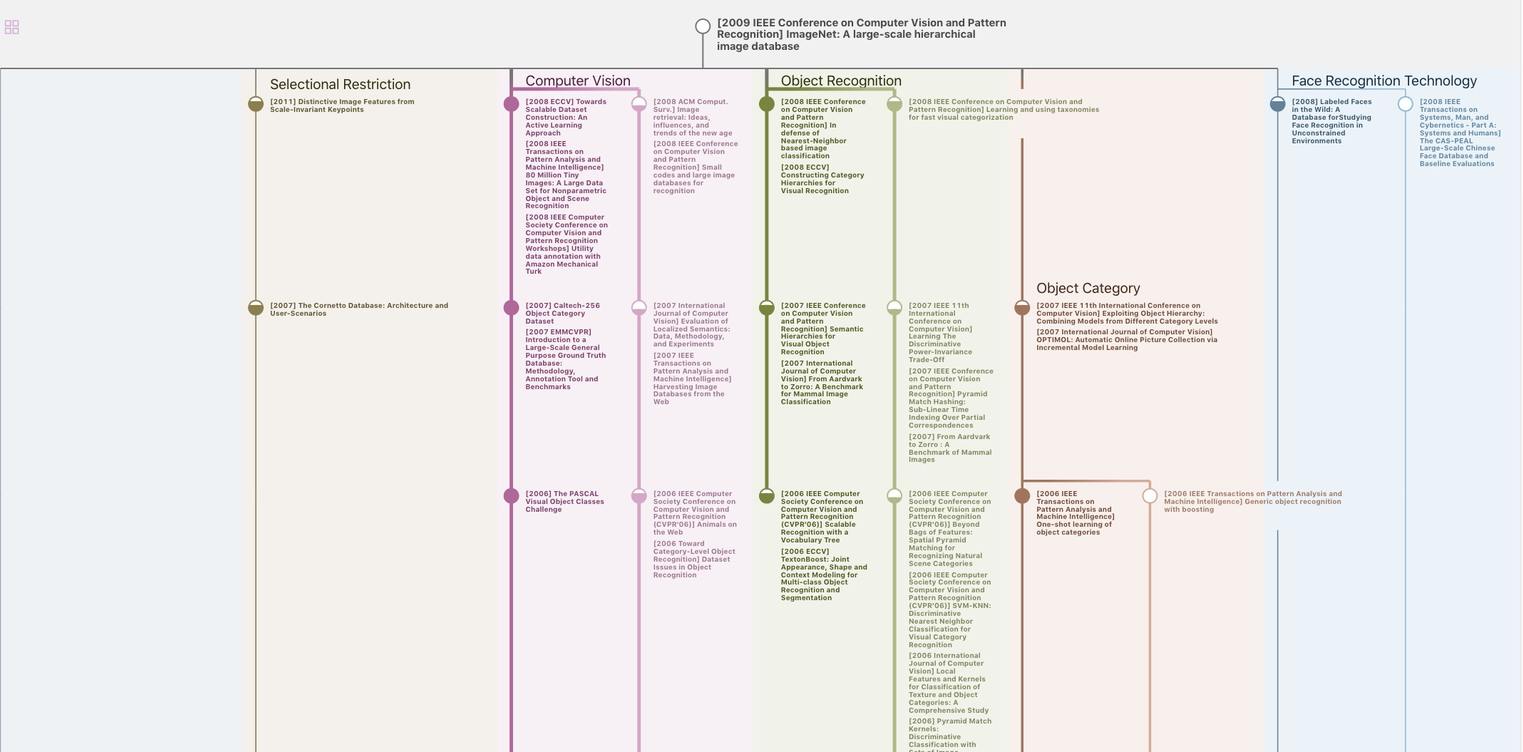
生成溯源树,研究论文发展脉络
Chat Paper
正在生成论文摘要