Semi-supervised Disentanglement with Independent Vector Variational Autoencoders
CoRR(2020)
摘要
We aim to separate the generative factors of data into two latent vectors in a variational autoencoder. One vector captures class factors relevant to target classification tasks, while the other vector captures style factors relevant to the remaining information. To learn the discrete class features, we introduce supervision using a small amount of labeled data, which can simply yet effectively reduce the effort required for hyperparameter tuning performed in existing unsupervised methods. Furthermore, we introduce a learning objective to encourage statistical independence between the vectors. We show that (i) this vector independence term exists within the result obtained on decomposing the evidence lower bound with multiple latent vectors, and (ii) encouraging such independence along with reducing the total correlation within the vectors enhances disentanglement performance. Experiments conducted on several image datasets demonstrate that the disentanglement achieved via our method can improve classification performance and generation controllability.
更多查看译文
关键词
independent vector variational
AI 理解论文
溯源树
样例
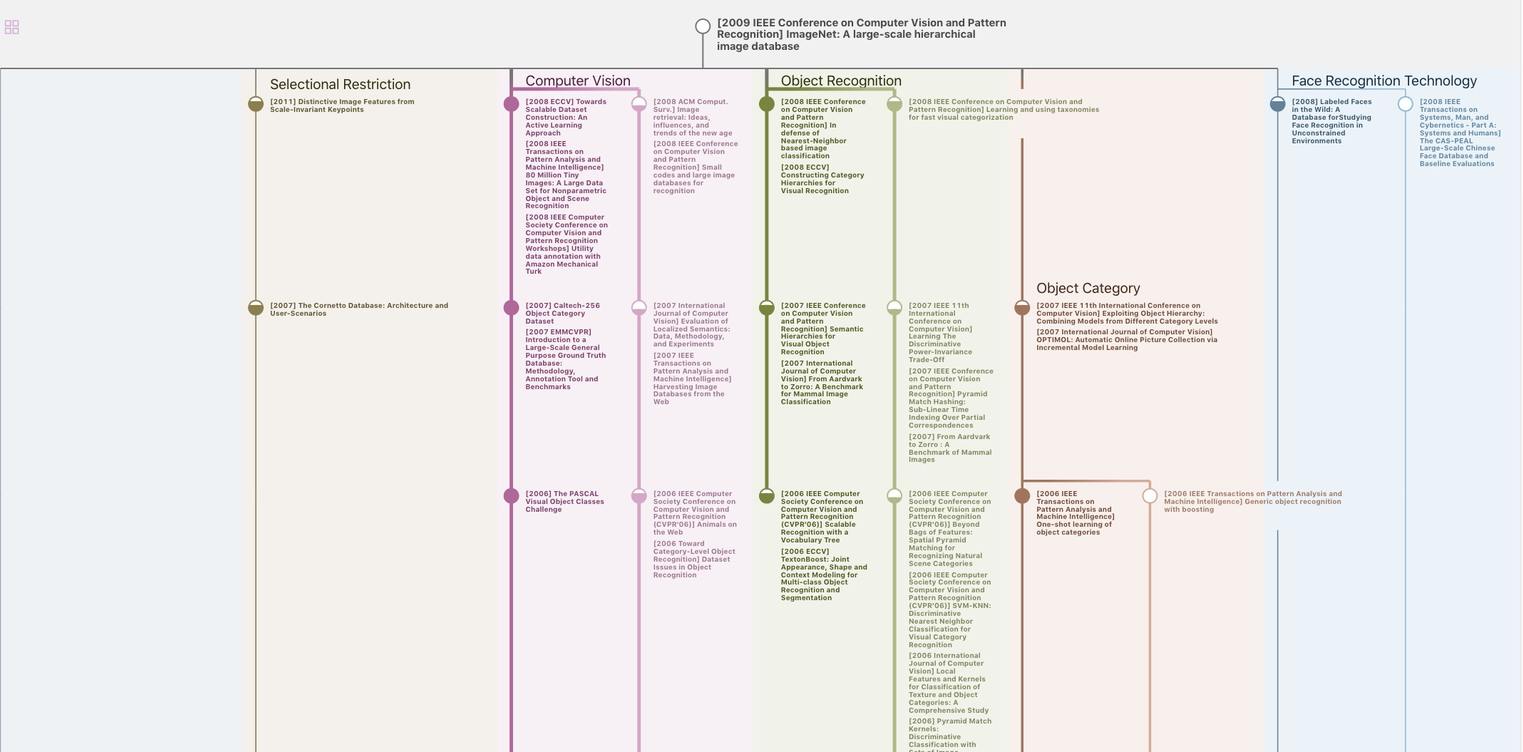
生成溯源树,研究论文发展脉络
Chat Paper
正在生成论文摘要