GraphCast-Qtf: an Improved Weather Prediction Model Based on Uncertainty Quantification Methods
2024 12th International Conference on Agro-Geoinformatics (Agro-Geoinformatics)(2024)
摘要
In the field of climate prediction, although the traditional numerical weather forecasting (NWP) method has made significant progress in the processing of historical data and improving the prediction accuracy, it still has significant limitations in using a large amount of historical climate data and improving the credibility of the prediction results. With the rapid development of deep learning technologies, deep learning-based climate prediction models, such as GraphCast, have shown their potential in global mid-term (10-day) climate variable prediction. However, existing methods often ignore the quantification of prediction uncertainty, which is crucial to improving the prediction’s reliability and practical application value. This study proposes an improved GraphCast model called GraphCast-Qtf, mainly by introducing uncertainty quantification mechanisms designed to improve the accuracy and credibility of climate predictions. This research is divided into data set construction, model construction, and system development. First, this study constructed a global climate dataset based on the ERA5 reanalysis data set of ECMWF, including historical weather states and associated climate variables from 1979 to the present. This dataset covers the global scope and provides highresolution climate variable data, providing rich input features for the training of deep learning models. Secondly, based on the GraphCast model, this study introduces the Bayesian neural network and Monte Carlo dropout technology to enable the model to output the confidence interval of the prediction value to quantify the uncertainty of the prediction results. These improvements enhance the model’s ability to learn from the intrinsic changing laws of climate systems and enable the model to handle better and reflect the uncertainty of prediction outcomes. Finally, we developed a simple climate prediction system based on the GraphCast-Qtf model. The system provides mediumterm climate forecasts and assessments of their uncertainty. The system’s front end is developed using JavaScript and the Vue framework. It is user-friendly and easy to operate, enabling users to provide scientific and reasonable decision support according to the prediction results and confidence intervals provided by the model. Through extensive experimental validation, the GraphCast-Qtf model performed better than traditional methods in terms of prediction accuracy and uncertainty quantification of multiple key climate variables. The application examples in the system development section further demonstrate the model’s effectiveness and potential in practical climate prediction scenarios.
更多查看译文
关键词
climate prediction,deep learning,uncertainty quantification,GraphCast,bias correction
AI 理解论文
溯源树
样例
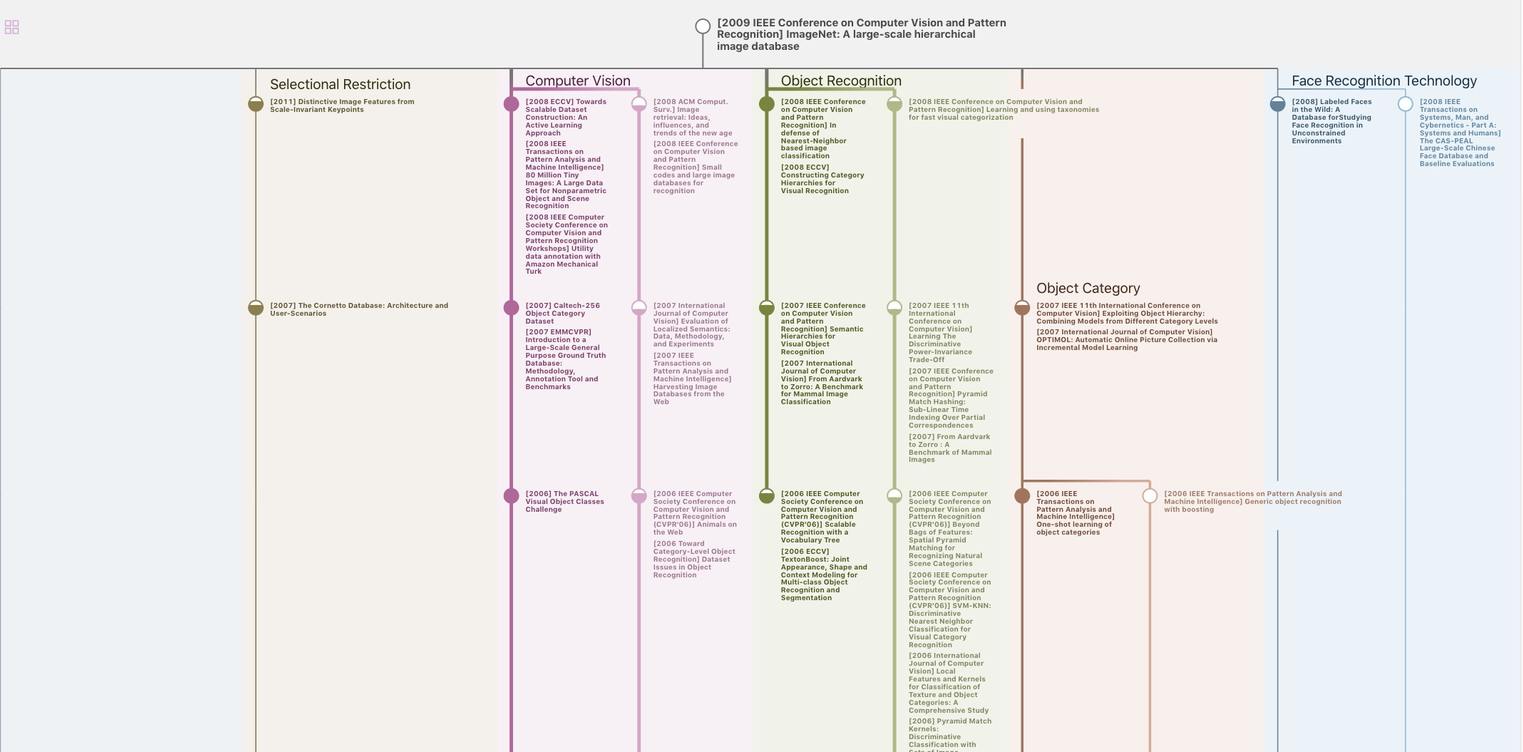
生成溯源树,研究论文发展脉络
Chat Paper
正在生成论文摘要