DeLoc: A Locality and Memory-Congestion-Aware Task Mapping Method for Modern NUMA Systems
IEEE access(2020)
摘要
The mapping of tasks to processor cores, called task mapping, is crucial to achieving scalable performance on multicore processors. On modern NUMA (non-uniform memory access) systems, the memory congestion problem could degrade the performance more severely than the data locality problem because heavy congestion on shared caches and memory controllers could cause long latencies. Conventional work on task mapping mostly focuses on improving the locality of memory accesses. However, our previous work showed that on modern NUMA systems, maximizing the locality can degrade the performance due to memory congestion. In this work, we propose a task mapping method that addresses the locality and the memory congestion problems to improve the performance of parallel applications. In the proposed method, first, the spatial and temporal communication behaviors of the applications are analyzed from the time-series dataset of communications among the parallel tasks. Then, a data clustering technique is employed to detect groups of tasks that potentially cause the memory congestion. Finally, this information is used to compute the task mapping to improve the locality and reduce the memory congestion. We also provide a set of metrics to describe the communication behaviors and to evaluate if the target application can benefit from our method. The proposed method is evaluated with the NPB and PARSEC applications on a real NUMA system and a multicore simulator. A detailed analysis of the sources of performance gain is also provided. Experimental results show that our method can achieve up to a 61% performance improvement compared with the state-of-the-art locality-based method.
更多查看译文
关键词
High-performance computing,locality,memory congestion,NUMA,process mapping,task mapping,thread mapping
AI 理解论文
溯源树
样例
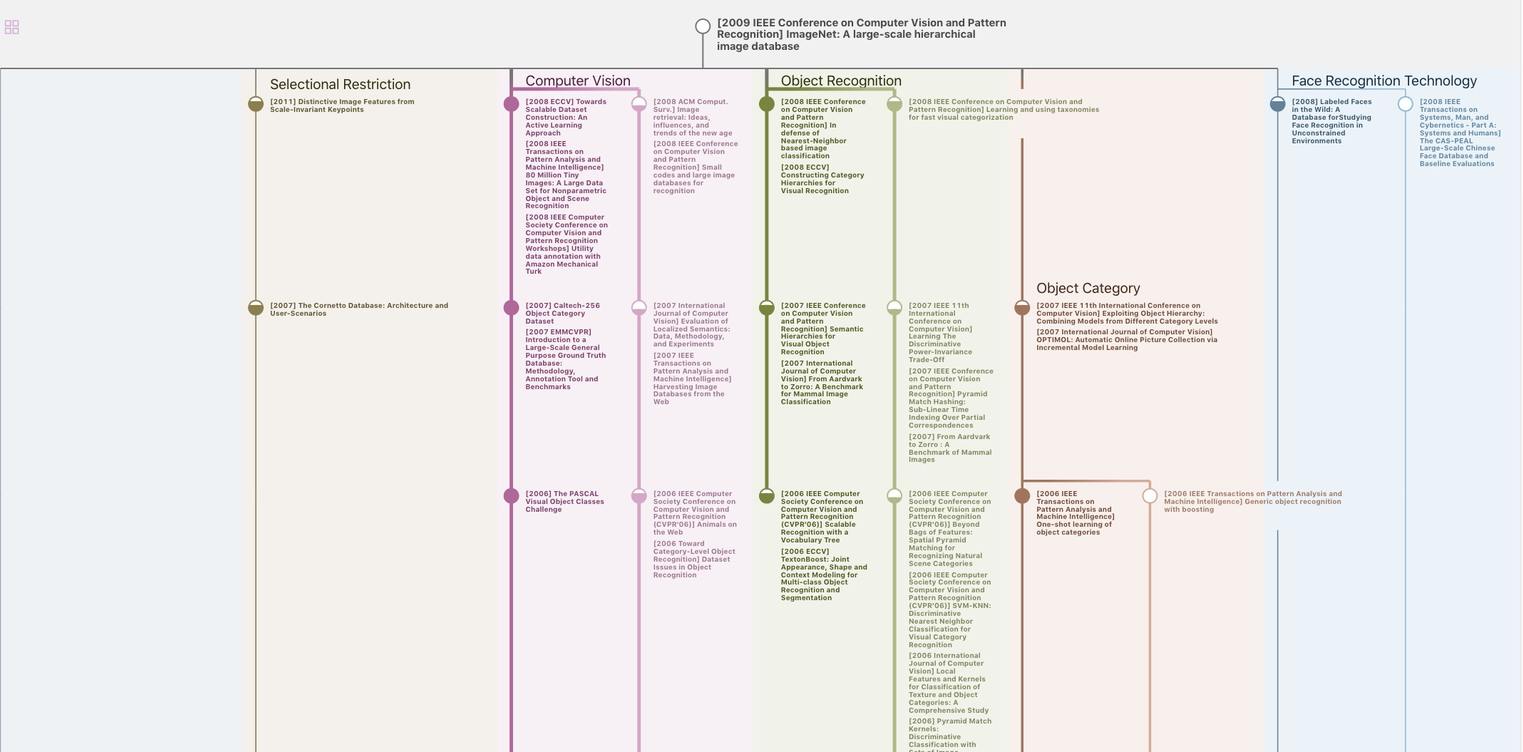
生成溯源树,研究论文发展脉络
Chat Paper
正在生成论文摘要