Drowsy Driving Detection Based On Fused Data And Information Granulation
IEEE ACCESS(2019)
摘要
To detect drowsy driving accurately, this paper collects the characteristic parameters of driver's operating behavior and vehicle's running state through simulation experiments. Then, the factor analysis was adopted to reduce the dimensionality of the characteristic parameters, and the composite factor scores was computed under both normal and drowsy states, forming a time series. Next, the time series of composite factor scores was divided into information granules and the particle swarm optimization (PSO) was implemented to optimize the dynamic time window for the characteristic parameters. After that, the mean and standard deviation were computed for each composite factor score in each sub-time window, and fused into one data. Finally, a drowsy driving model was established with LIBSVM based on the fused data. The experimental results demonstrate that our model achieved an accuracy of 86.47% in detecting drowsy driving. The research finding shed new lights on the detection of drowsy driving.
更多查看译文
关键词
Drowsy driving,information granulation,support vector machine (SVM),dynamic time window
AI 理解论文
溯源树
样例
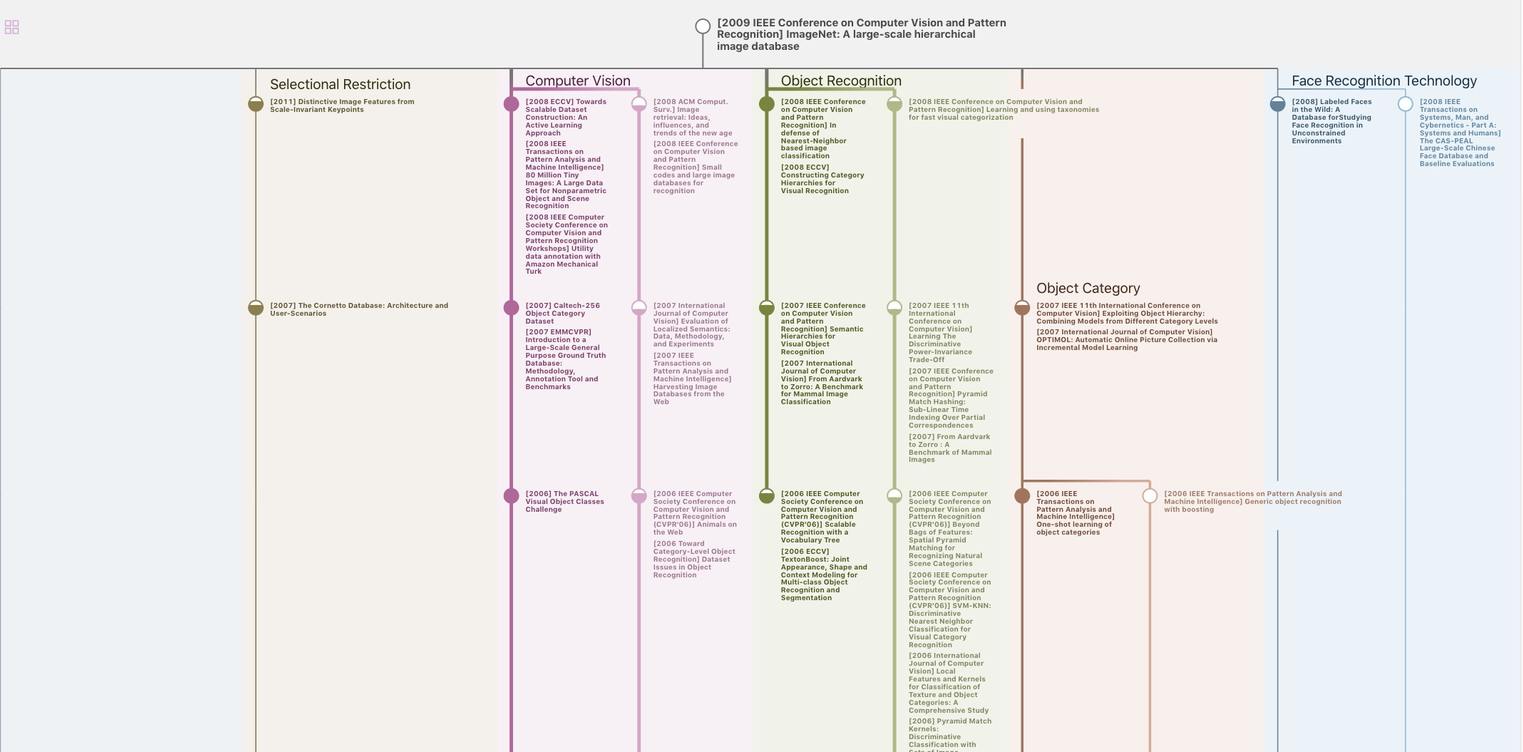
生成溯源树,研究论文发展脉络
Chat Paper
正在生成论文摘要