Improving Deep Learning By Regularized Scale-Free Mse Of Representations
NEURAL INFORMATION PROCESSING (ICONIP 2019), PT I(2019)
摘要
Nowadays, the research for learning representations with deep neural networks (DNNs) is attracting more and more attentions. In general, most of studies focus on designing principles for learning representations when the learning model is stochastic, which is not widely used for all kinds of machine learning tasks. In this paper, we try to seek a principle to learn efficient representations for deterministic DNNs. Considering that the last DNN layer is often a linear mapping, we utilize the generalized linear model (GLM) theory to design a principle for learning representations. First, via choosing proper link functions, we build a connection between representation learning and the optimization of the last linear layer. Next, we propose a representation learning principle, scale-free mean square error (scale-free MSE), by leveraging the MSE analysis in the GLM. Here, we find that representations with a smaller scale-free MSE can lead to a better estimation of the parameters. Then, we further propose an algorithm to learn representations with a small scale-free MSE. Here, a regularization term is employed to make the scale-free MSE get small. Finally, we test our algorithm with typical DNN models in both supervised and unsupervised learning tasks. The experiment results show that our proposed algorithm is suitable to learn efficient representations over various of experiment settings.
更多查看译文
关键词
Representation learning, Deep neural networks, Generalized linear model
AI 理解论文
溯源树
样例
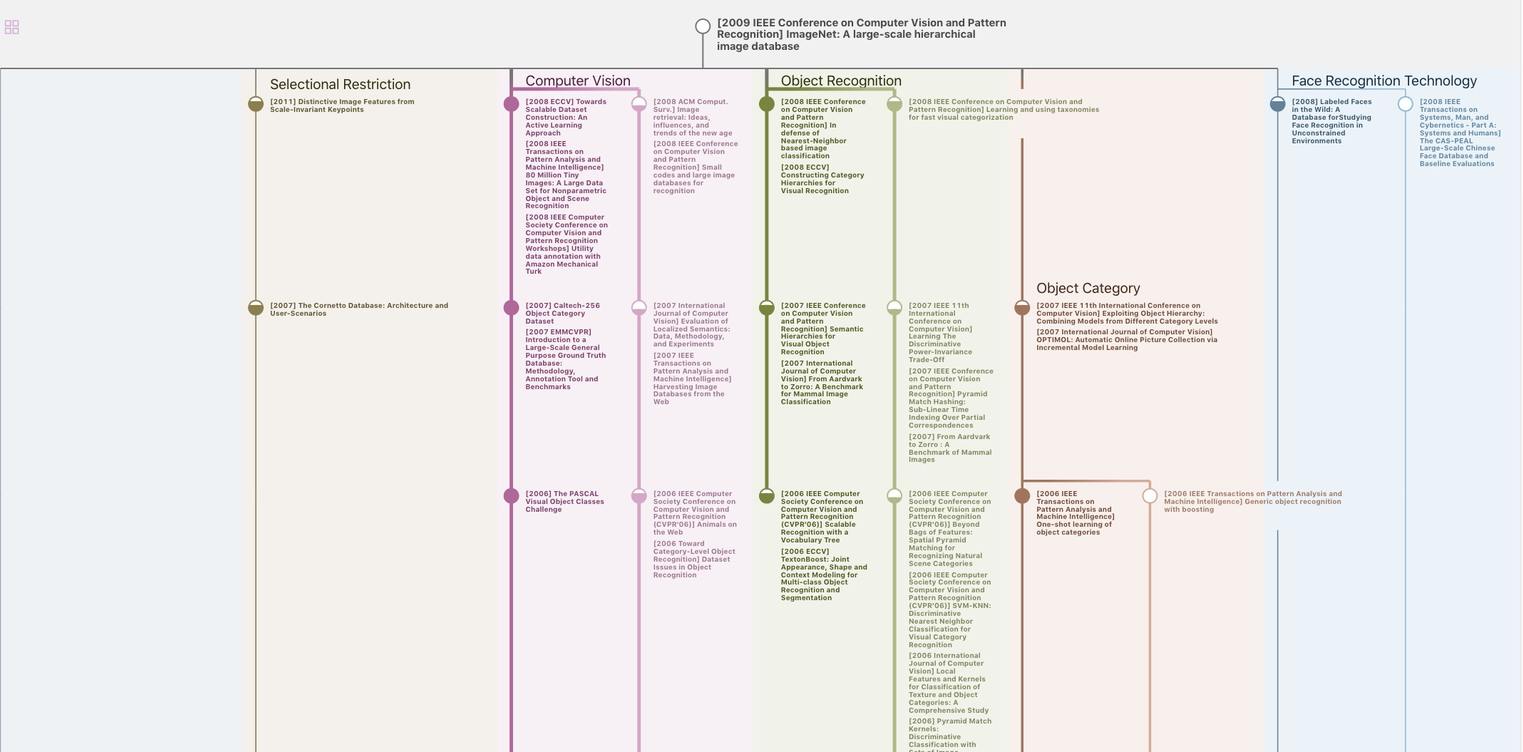
生成溯源树,研究论文发展脉络
Chat Paper
正在生成论文摘要