Learning-based Local-to-global Landmark Annotation for Automatic 3D Cephalometry
Physics in medicine & biology/Physics in medicine and biology(2020)
摘要
The annotation of three-dimensional (3D) cephalometric landmarks in 3D computerized tomography (CT) has become an essential part of cephalometric analysis, which is used for diagnosis, surgical planning, and treatment evaluation. The automation of 3D landmarking with high-precision remains challenging due to the limited availability of training data and the high computational burden. This paper addresses these challenges by proposing a hierarchical deep-learning method consisting of four stages: 1) a basic landmark annotator for 3D skull pose normalization, 2) a deep-learning-based coarse-to-fine landmark annotator on the midsagittal plane, 3) a low-dimensional representation of the total number of landmarks using variational autoencoder (VAE), and 4) a local-to-global landmark annotator. The implementation of the VAE allows two-dimensional-image-based 3D morphological feature learning and similarity/dissimilarity representation learning of the concatenated vectors of cephalometric landmarks. The proposed method achieves an average 3D point-to-point error of 3.63 mm for 93 cephalometric landmarks using a small number of training CT datasets. Notably, the VAE captures variations of craniofacial structural characteristics.
更多查看译文
关键词
cephalometric landmark,computerized tomography,deep learning
AI 理解论文
溯源树
样例
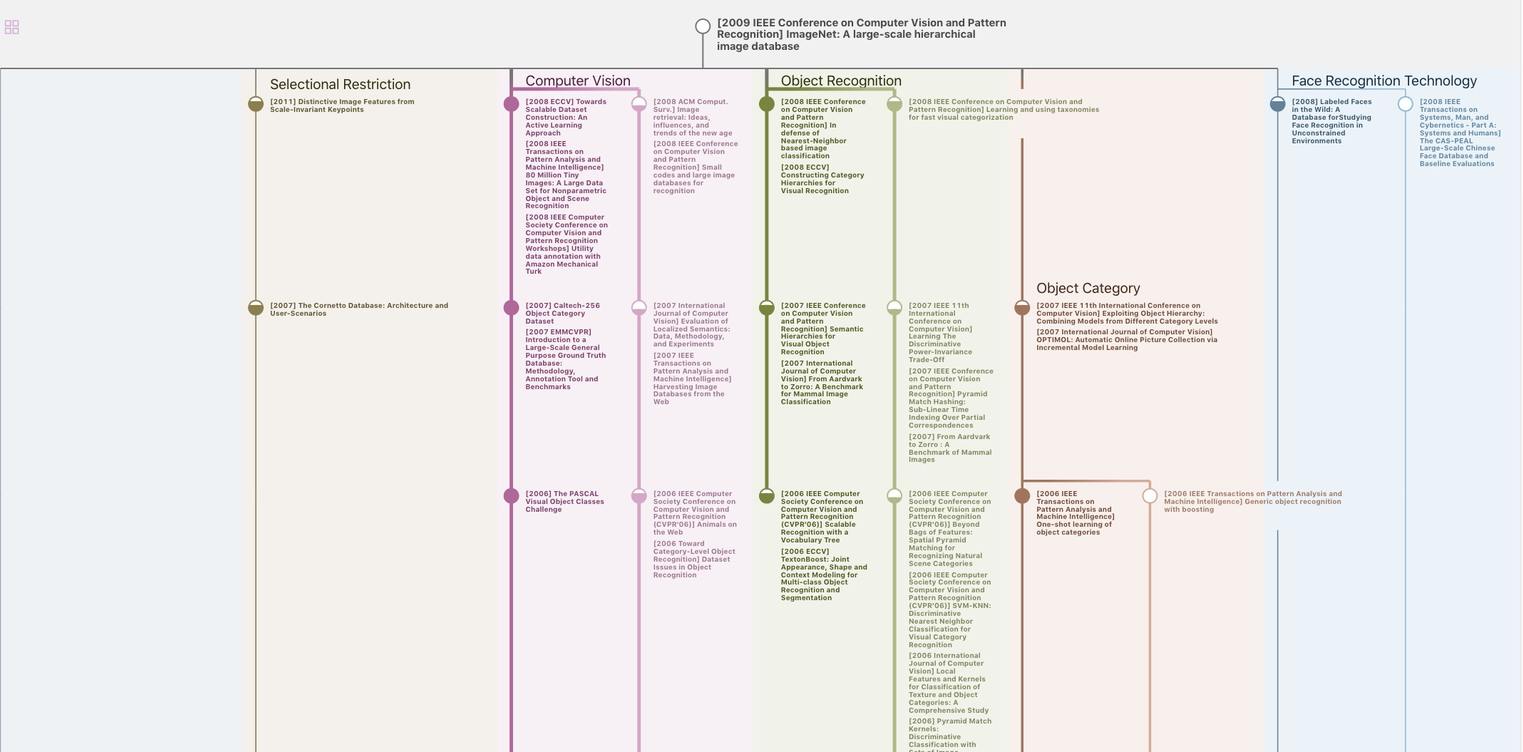
生成溯源树,研究论文发展脉络
Chat Paper
正在生成论文摘要