Self-Organizing Maps Hybrid Implementation Based on Stochastic Computing
2019 XXXIV Conference on Design of Circuits and Integrated Systems (DCIS)(2019)
摘要
Internet of Things (IoT) applications and mobile systems are more and more dependent on Machine Learning based solutions, thus requiring a big computational power with a low cost in terms of power consumption. This fact has revived the interest in nonconventional hardware computing methods capable to implement complex functions in a simple way in contrast with the conventional ones. This work proposes a novel hardware/software hybrid Self-Organizing Map (SOM) implementation using stochastic computing. In turn, to support this development, several stochastic block designs are presented as the squared Euclidian distance, and the Winner-Take-All (WTA) similarity check. The capabilities and performance of the methodology is tested over a well-known classification task as the Iris flower benchmark, archiving the same classification performance than the software solutions. The proposed solution presents a low-cost methodology in terms of hardware resources and power, due to its inherent capacity to implement complex functions in a simple way. This enables the methodology to implement large self-learning classifiers based on SOM with low hardware requirements.
更多查看译文
关键词
Stochastic computing,Probabilistic logic,Unsupervised learning,Self-organizing feature maps,Field programmable gate arrays
AI 理解论文
溯源树
样例
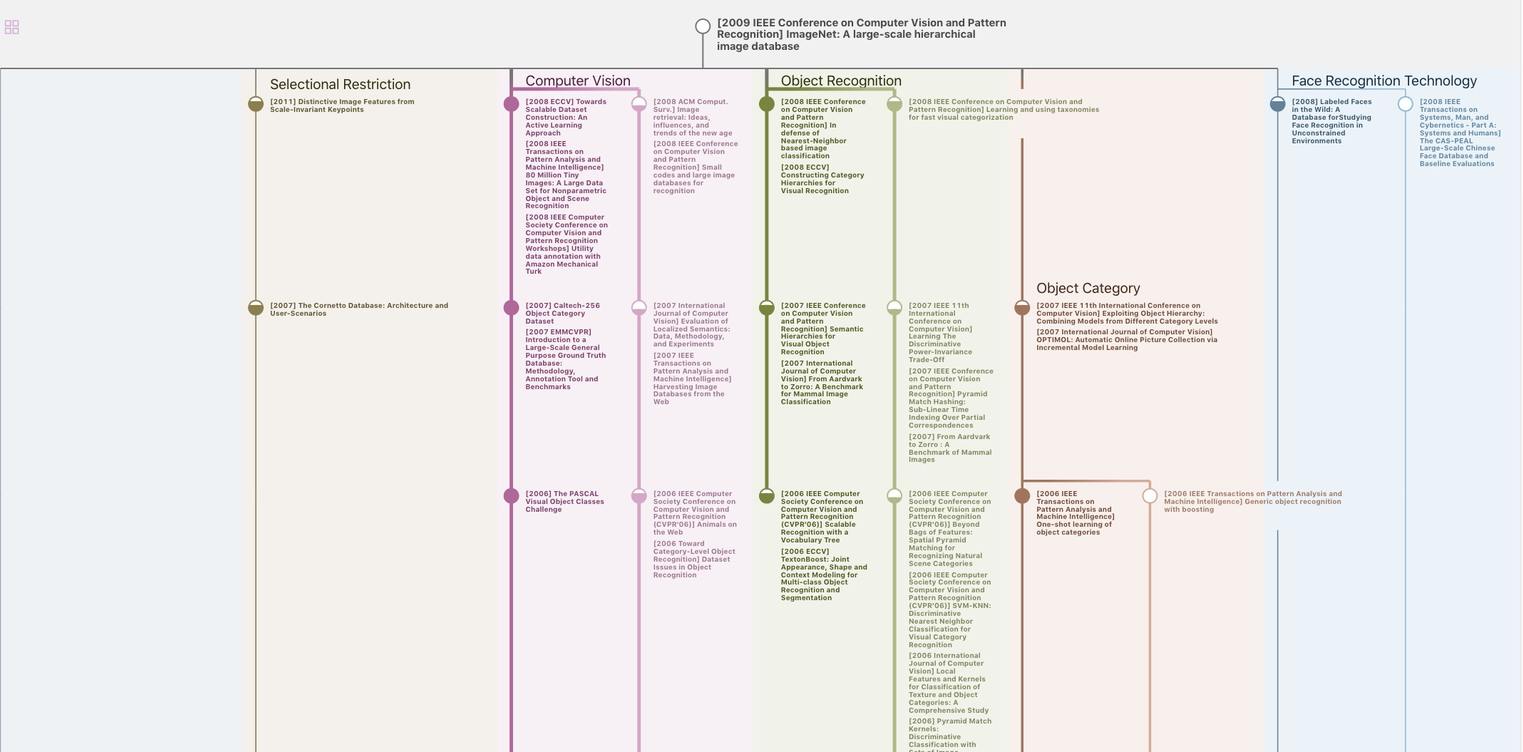
生成溯源树,研究论文发展脉络
Chat Paper
正在生成论文摘要