Predicting Water Quality For The Woronora Delivery Network With Sparse Samples
2019 19TH IEEE INTERNATIONAL CONFERENCE ON DATA MINING (ICDM 2019)(2019)
摘要
Monitoring drinking water quality in the entire delivery network, mainly indicated by total chlorine (TC), is a critical component of overall water supply management. However, it is extremely difficult to collect sufficient TC data from the network at customer sites, which makes it sparse for comprehensive modelling. This paper details an approach that provides TC prediction within the entire Woronora delivery network in Sydney in the next 24 hours. First, the hydraulic system is employed to capture the topology of the delivery network, so that the water travel time can be estimated using predicted water demand. The travel time links the upstream (reservoir) data to the downstream (resident) data. Then, a two-step strategy is proposed as a semi-parametric method to determine the crucial factors and build Bayesian model for TC decay to predict TC with the travel time. Lastly, the uncertainties of both data and the model are analysed to define the boundaries of prediction for better decision making. Several operational stages are involved when the approach is being deployed, including prediction interpretation, interactive tool development for water quality mapping and visualisation, and proactive optimisation. This has established a successful initiative to improve the overall water supply management for the entire Woronora delivery network.
更多查看译文
关键词
Bayesian models,uncertainty analysis,sparse sample,data mining model deployment,water quality prediction
AI 理解论文
溯源树
样例
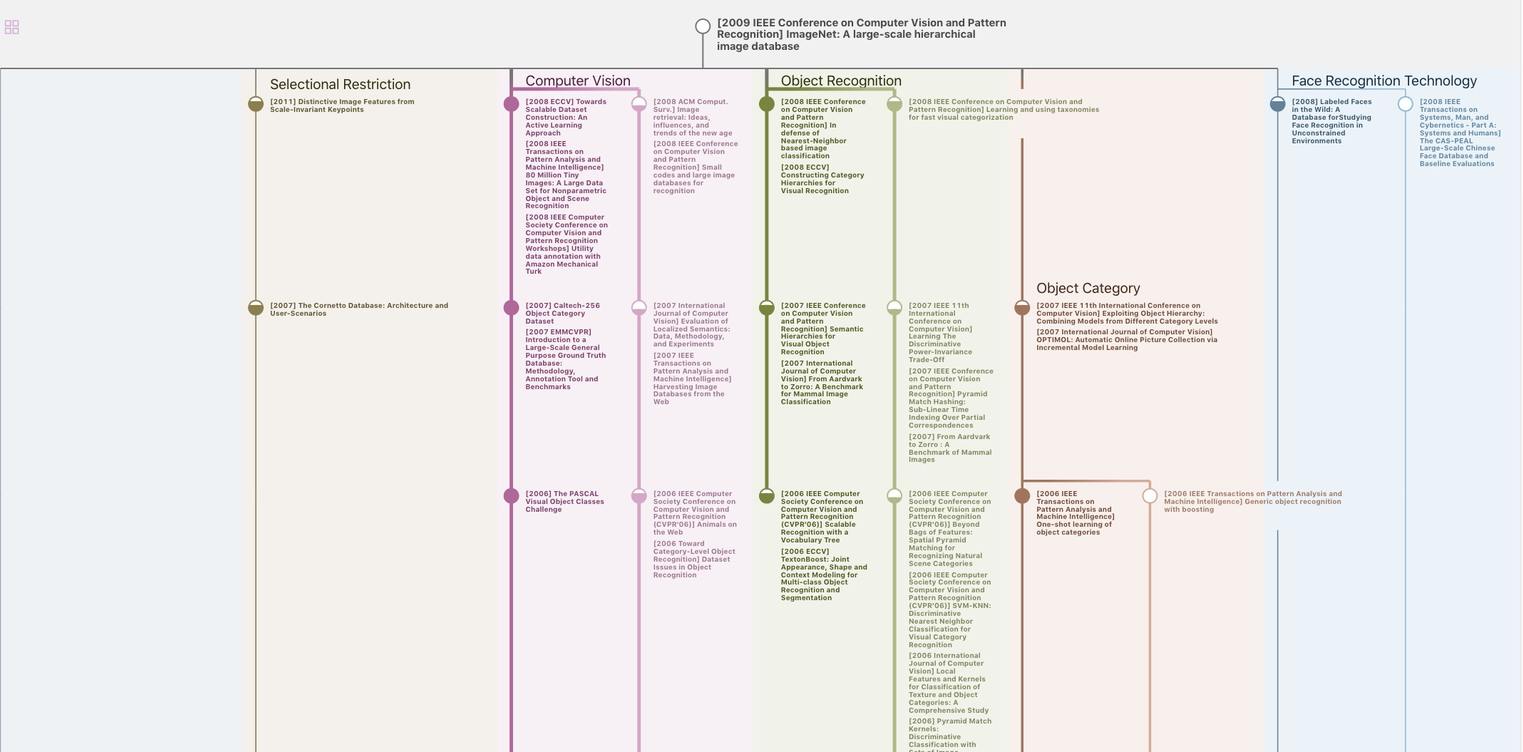
生成溯源树,研究论文发展脉络
Chat Paper
正在生成论文摘要