Pattern Recognition Applications and Methods - 8th International Conference, ICPRAM 2019, Prague, Czech Republic, February 19-21, 2019, Revised Selected Papers
Lecture Notes in Computer Science(2020)
摘要
Functional principal component analysis (FPCA) is a natural tool for investigating the functional pattern of functional data. Clustering functional data using FPCA can be an important area in machine learning for signal processing, in particular, for signals that contain a deterministic component. Also, FPCA can be useful for analysis of random signals if an appropriate transformation is applied. In this work, we propose a novel approach by extracting key features of EEG signals in the Fourier spectral domain using FPCA. By first transforming EEG signals into their Fourier power spectra, the functionality of signals is greatly enhanced. Due to this improvement, the application of FPCA becomes much more meaningful in signal feature extraction. Our study shows a great potential of using spectral domain FPCA as a feature extractor for processing EEG signals in both epilepsy diagnosis and epileptic seizure detection.
更多查看译文
AI 理解论文
溯源树
样例
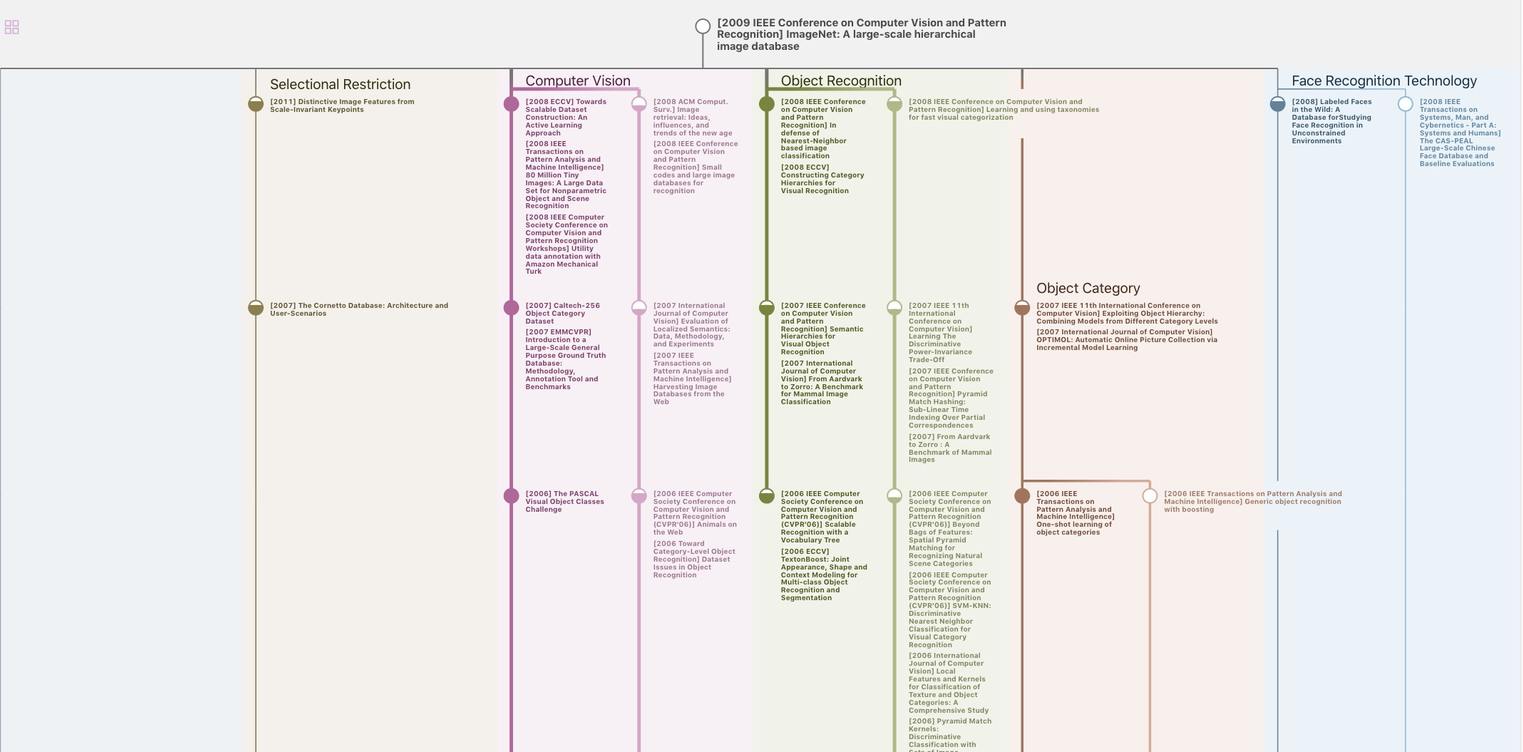
生成溯源树,研究论文发展脉络
Chat Paper
正在生成论文摘要