VSEC-LDA: Boosting Topic Modeling with Embedded Vocabulary Selection
CoRR(2020)
摘要
Topic modeling has found wide application in many problems where latent structures of the data are crucial for typical inference tasks. When applying a topic model, a relatively standard pre-processing step is to first build a vocabulary of frequent words. Such a general pre-processing step is often independent of the topic modeling stage, and thus there is no guarantee that the pre-generated vocabulary can support the inference of some optimal (or even meaningful) topic models appropriate for a given task, especially for computer vision applications involving "visual words". In this paper, we propose a new approach to topic modeling, termed Vocabulary-Selection-Embedded Correspondence-LDA (VSEC-LDA), which learns the latent model while simultaneously selecting most relevant words. The selection of words is driven by an entropy-based metric that measures the relative contribution of the words to the underlying model, and is done dynamically while the model is learned. We present three variants of VSEC-LDA and evaluate the proposed approach with experiments on both synthetic and real databases from different applications. The results demonstrate the effectiveness of built-in vocabulary selection and its importance in improving the performance of topic modeling.
更多查看译文
关键词
embedded vocabulary selection,topic modeling,vsec-lda
AI 理解论文
溯源树
样例
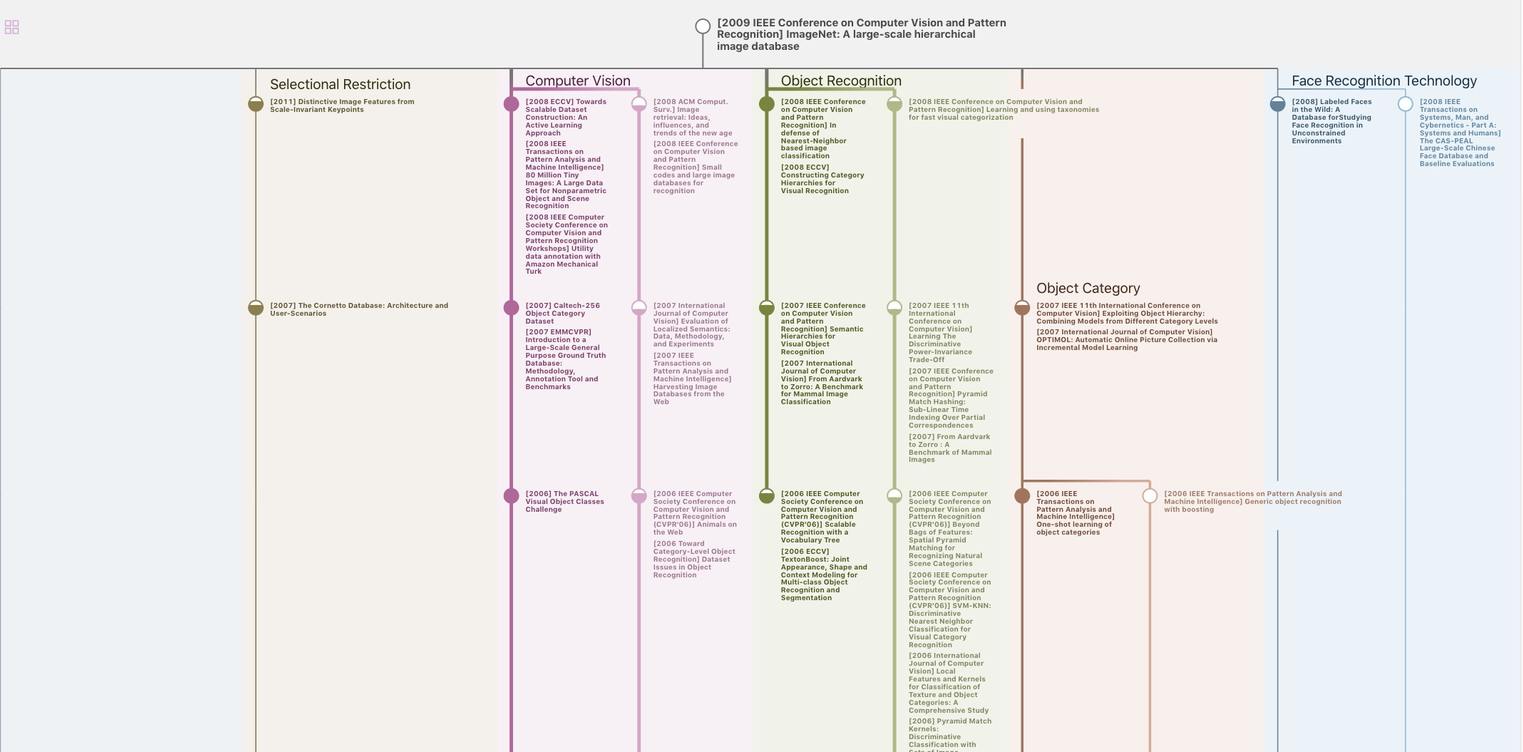
生成溯源树,研究论文发展脉络
Chat Paper
正在生成论文摘要