AGN Feedback in the FR II Galaxy 3C 220.1
Monthly Notices of the Royal Astronomical Society(2020)
Univ Alabama | Harvard Smithsonian Ctr Astrophys | Univ Bristol | Univ Virginia
Abstract
ABSTRACT We present results from a deep (174 ks) Chandra observation of the FR-II radio galaxy 3C 220.1, the central brightest cluster galaxy (BCG) of a kT ∼ 4 keV cluster at z = 0.61. The temperature of the hot cluster medium drops from ∼5.9 to ∼3.9 keV at ∼35 kpc radius, while the temperature at smaller radii may be substantially lower. The central active galactic nucleus (AGN) outshines the whole cluster in X-rays, with a bolometric luminosity of 2.0 × 1046 erg s−1 (∼10 per cent of the Eddington rate). The system shows a pair of potential X-ray cavities ∼35 kpc east and west of the nucleus. The cavity power is estimated within the range of 1.0 × 1044 and 1.7 × 1045 erg s−1, from different methods. The X-ray enhancements in the radio lobes could be due to inverse Compton emission, with a total 2–10 keV luminosity of ∼8.0 × 1042 erg s−1. We compare 3C 220.1 with other cluster BCGs, including Cygnus A, as there are few BCGs in rich clusters hosting an FR-II galaxy. We also summarize the jet power of FR-II galaxies from different methods. The comparison suggests that the cavity power of FR-II galaxies likely underestimates the jet power. The properties of 3C 220.1 suggest that it is at the transition stage from quasar-mode feedback to radio-mode feedback.
MoreTranslated text
Key words
galaxies: groups: individual: 3C 220.1,galaxies: jets,X-rays: galaxies: clusters
PDF
View via Publisher
AI Read Science
AI Summary
AI Summary is the key point extracted automatically understanding the full text of the paper, including the background, methods, results, conclusions, icons and other key content, so that you can get the outline of the paper at a glance.
Example
Background
Key content
Introduction
Methods
Results
Related work
Fund
Key content
- Pretraining has recently greatly promoted the development of natural language processing (NLP)
- We show that M6 outperforms the baselines in multimodal downstream tasks, and the large M6 with 10 parameters can reach a better performance
- We propose a method called M6 that is able to process information of multiple modalities and perform both single-modal and cross-modal understanding and generation
- The model is scaled to large model with 10 billion parameters with sophisticated deployment, and the 10 -parameter M6-large is the largest pretrained model in Chinese
- Experimental results show that our proposed M6 outperforms the baseline in a number of downstream tasks concerning both single modality and multiple modalities We will continue the pretraining of extremely large models by increasing data to explore the limit of its performance
Try using models to generate summary,it takes about 60s
Must-Reading Tree
Example
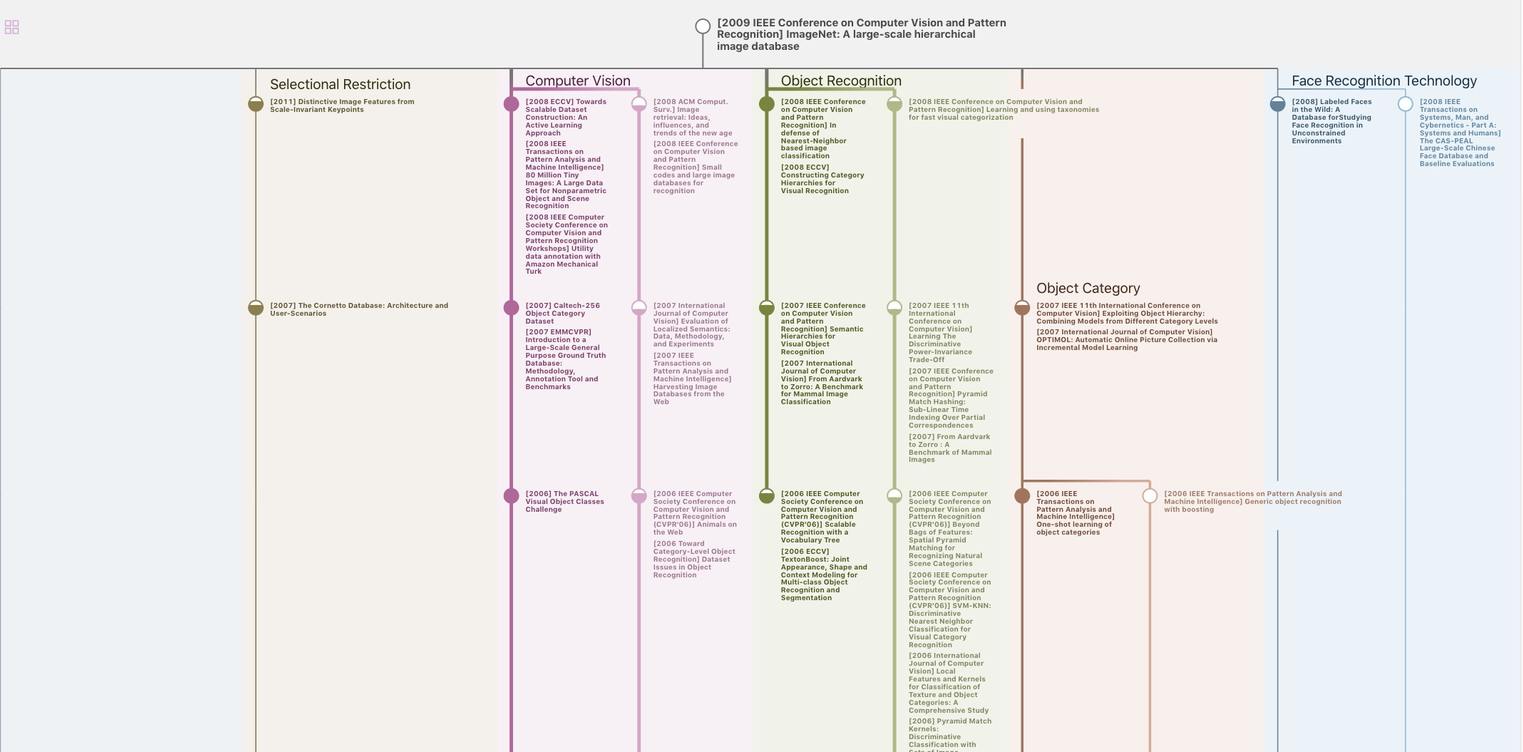
Generate MRT to find the research sequence of this paper
Related Papers
Hidden Treasures in the Unknown 3CR Extragalactic Radio Sky: a Multi-Wavelength Approach
The Astrophysical Journal Supplement Series 2021
被引用6
The Contribution of Non-Central Radio Galaxies to AGN Feedback in Rich Galaxy Clusters
Monthly Notices of the Royal Astronomical Society 2022
被引用4
AGN Feedback in Groups and Clusters of Galaxies
Handbook of X-ray and Gamma-ray Astrophysics 2024
被引用6
MONTHLY NOTICES OF THE ROYAL ASTRONOMICAL SOCIETY 2023
被引用8
Multiple Shock Fronts in RBS 797: the Chandra Window on Shock Heating in Galaxy Clusters
ASTROPHYSICAL JOURNAL 2023
被引用6
GALAXIES 2023
被引用0
A New Hydrodynamic Spherical Accretion Exact Solution and Its Quasi-spherical Perturbations
The Astrophysical Journal 2023
被引用1
MONTHLY NOTICES OF THE ROYAL ASTRONOMICAL SOCIETY 2023
被引用3
Data Disclaimer
The page data are from open Internet sources, cooperative publishers and automatic analysis results through AI technology. We do not make any commitments and guarantees for the validity, accuracy, correctness, reliability, completeness and timeliness of the page data. If you have any questions, please contact us by email: report@aminer.cn
Chat Paper
去 AI 文献库 对话