Semi-Supervised Cross-Modal Retrieval with Label Prediction.
IEEE transactions on multimedia(2020)
摘要
Cross-modal retrieval tasks with image-text, audio-image, etc. are gaining increasing importance due to an abundance of data from multiple modalities. In general, supervised approaches give significant improvement over their unsupervised counterparts at the additional cost of labeling or annotation of the training data. Recently, semi-supervised methods are becoming popular as they provide an elegant framework to balance the conflicting requirement of labeling cost and accuracy. In this work, we propose a novel deep semi-supervised framework, which can seamlessly handle both labeled as well as unlabeled data. The network has two important components: (a) first, the labels for the unlabeled portion of the training data are predicted using the label prediction component, and then (b) a common representation for both the modalities is learned for performing cross-modal retrieval. The two parts of the network are trained sequentially one after the other. Extensive experiments on three benchmark datasets, Wiki, Pascal VOC, and NUS-WIDE demonstrate that the proposed framework outperforms the state-of-the-art for both supervised and semi-supervised settings.
更多查看译文
关键词
Training data,Annotations,Training,Noise measurement,Entropy,Task analysis,Labeling,Semi-Supervised Learning,Multi-Label Prediction,Binary Cross Entropy Loss,Cross-Modal Retrieval
AI 理解论文
溯源树
样例
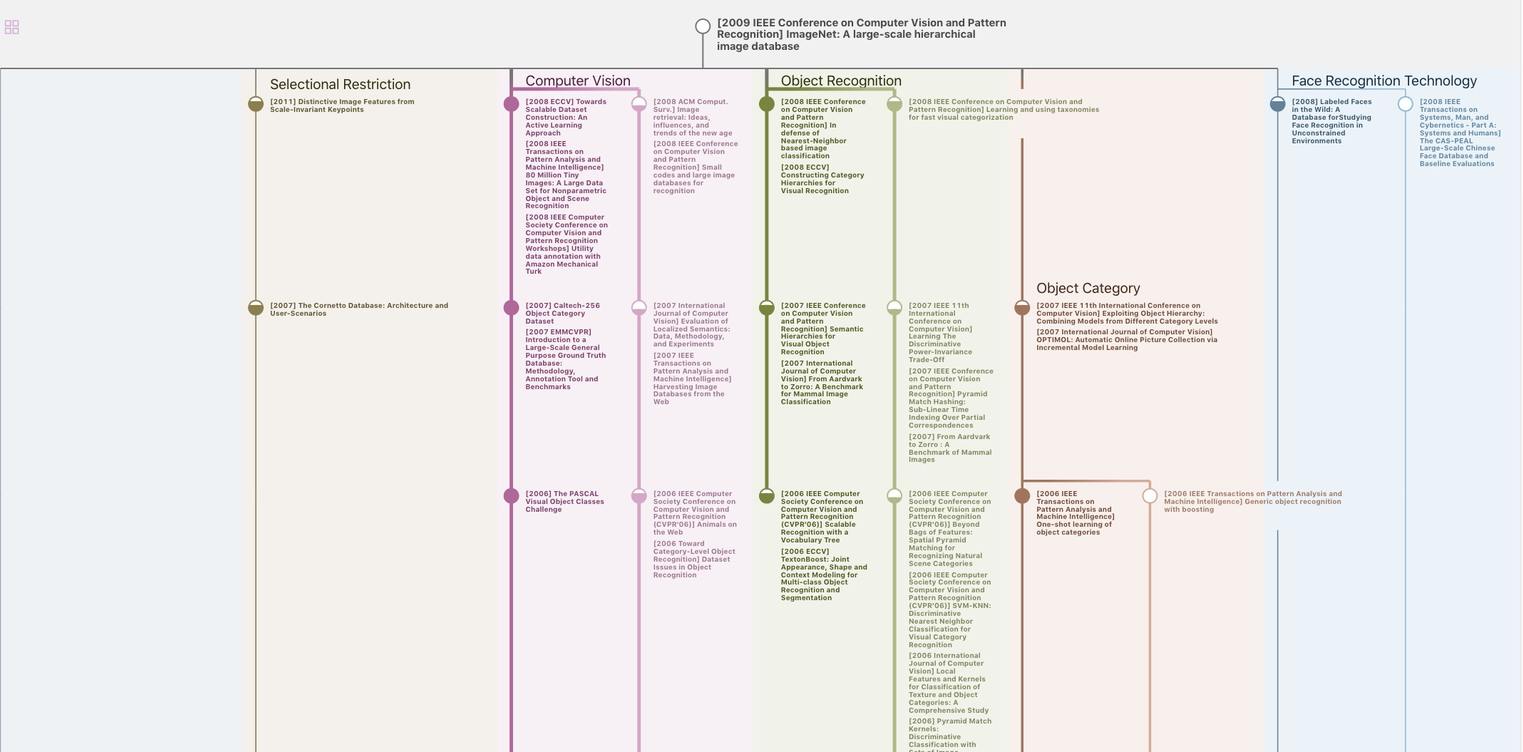
生成溯源树,研究论文发展脉络
Chat Paper
正在生成论文摘要