Probabilistic just-in-time approach for nonlinear modeling with Bayesian nonlinear feature extraction
Chemometrics and Intelligent Laboratory Systems(2020)
摘要
In this work, we propose a probabilistic just-in-time (PJIT) modeling methodology with nonlinear feature extraction for estimating quality variables of interest. In literature, deterministic nonlinear feature extraction methods have been employed to deal with high dimensional input data. However, these methods require prespecifying the latent dimensions, which often results in overfitting. To circumvent this issue, we employ the Bayesian Gaussian process latent variable model (BGPLVM) to extract nonlinear latent variables and determine their dimensions automatically. Owing to the probabilistic framework, the proposed approach involves computing the variational distribution of latent variables for the query sample as well as historical samples, and selecting relevant samples based on a distribution measure for building a local Gaussian process model to predict the quality variable. Furthermore, the applicability of the proposed approach to missing data and multi-rate data is discussed. Two case studies are presented to demonstrate the efficacy of the proposed PJIT model.
更多查看译文
关键词
Nonlinear dimensionality reduction,Soft sensor,Probabilistic models,Missing values
AI 理解论文
溯源树
样例
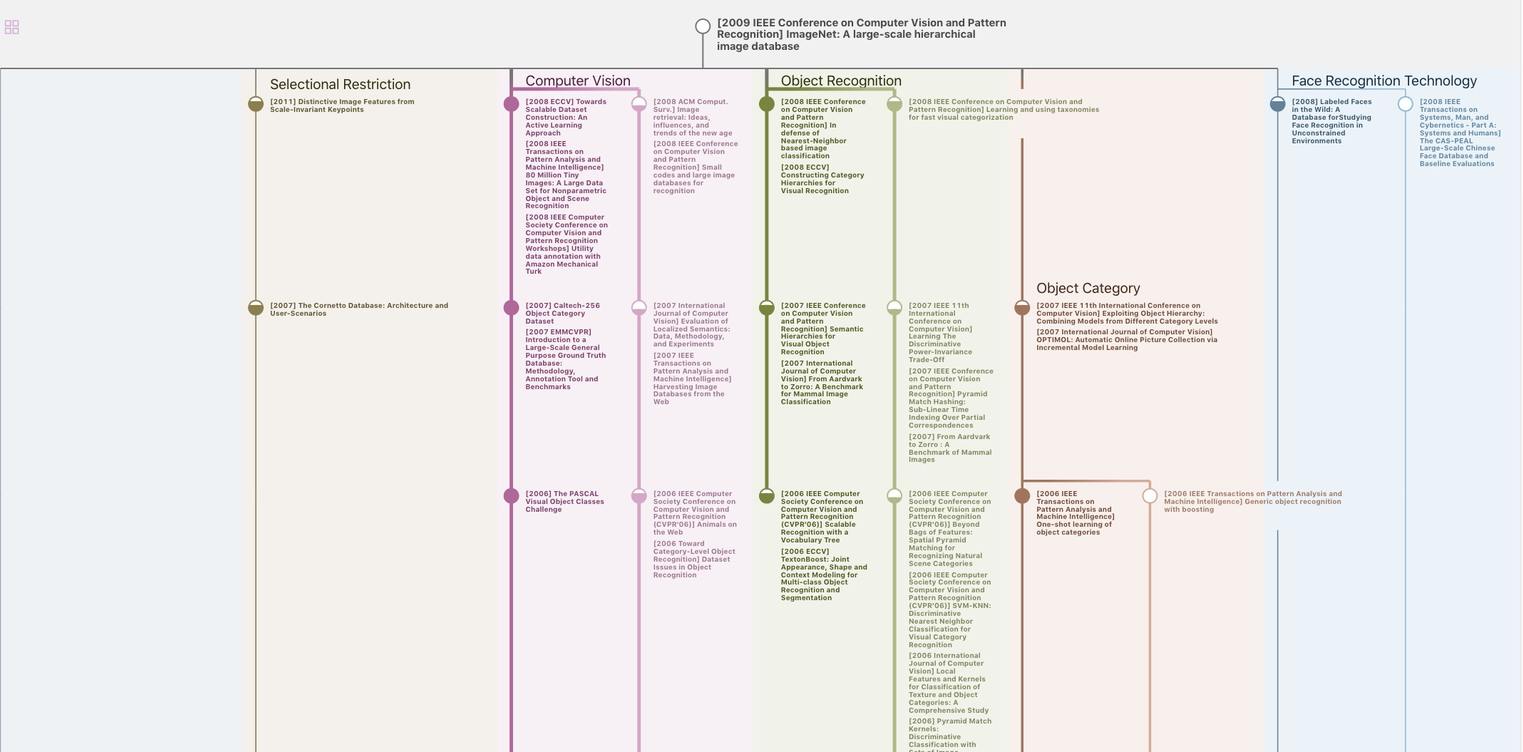
生成溯源树,研究论文发展脉络
Chat Paper
正在生成论文摘要