A 120-Ke− Full-Well Capacity 160-Μv/e− Conversion Gain 2.8-Μm Backside-Illuminated Pixel with a Lateral Overflow Integration Capacitor
Italian National Conference on Sensors(2019)
关键词
CMOS image sensor,lateral overflow integration capacitor,wide dynamic range,high sensitivity,high full-well capacity,single exposure,backside illumination
AI 理解论文
溯源树
样例
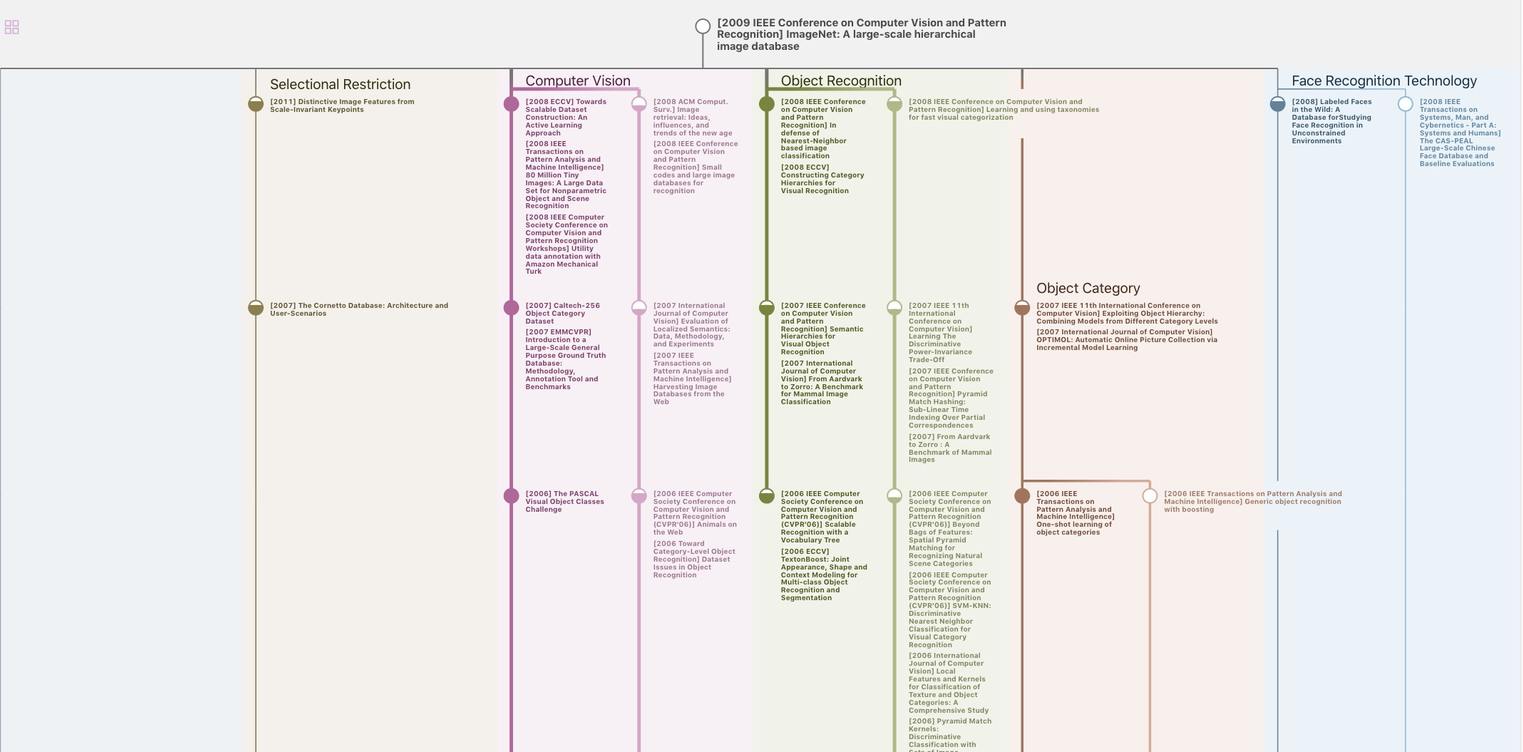
生成溯源树,研究论文发展脉络
Chat Paper
正在生成论文摘要