The Prevention Dataset: A Novel Benchmark For Prediction Of Vehicles Intentions
2019 IEEE INTELLIGENT TRANSPORTATION SYSTEMS CONFERENCE (ITSC)(2019)
Abstract
Recent advances in autonomous driving have shown the importance of endowing self-driving cars with the ability of predicting the intentions and future trajectories of other traffic participants. In this paper, we introduce the PREVENTION dataset, which provides a large number of accurate and detailed annotations of vehicles trajectories, categories, lanes, and events, including cut-in, cut-out, left/right lane changes, and hazardous maneuvers. Data is collected from 6 sensors of different nature (LiDAR, radar, and cameras), providing both redundancy and complementarity, using an instrumented vehicle driven under naturalistic conditions. The dataset contains 356 minutes, corresponding to 540 km of distance traveled, including more than 4M detections, and more than 3K trajectories. Each vehicle is unequivocally identified with a unique id and the corresponding image, LiDAR and radar coordinates. No other public dataset provides such a rich amount of data on different road scenarios and critical situations and such a long-range coverage around the ego-vehicle (up to 80 m) using a redundant sensor set-up and providing enhanced lane-change annotations of surrounding vehicles. The dataset is ready to develop learning and inference algorithms for predicting vehicles intentions and future trajectories, including inter-vehicle interactions.
MoreTranslated text
Key words
PREVENTION dataset,autonomous driving,traffic participants,vehicles trajectories,LiDAR,instrumented vehicle,redundant sensor set-up,inter-vehicle interactions,vehicles intentions prediction,lane-change annotations
AI Read Science
Must-Reading Tree
Example
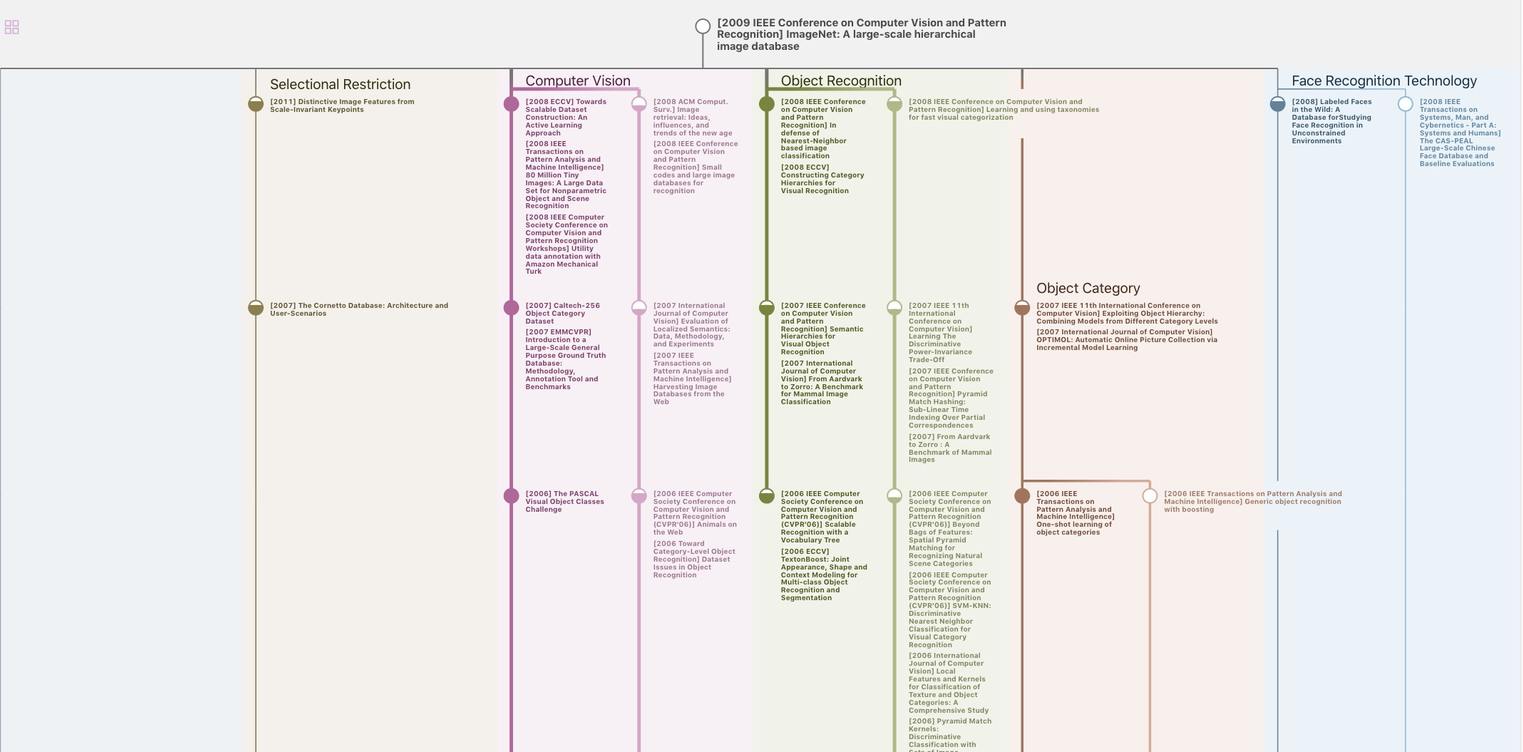
Generate MRT to find the research sequence of this paper
Chat Paper
Summary is being generated by the instructions you defined