Urban Localization Inside Cadastral Maps Using A Likelihood Field Representation
2019 IEEE INTELLIGENT TRANSPORTATION SYSTEMS CONFERENCE (ITSC)(2019)
摘要
In this paper we propose the use of existing resources, cadastral plans in particular, to build maps for vehicle localization without requiring the prior passage of a mapping vehicle. This solves the inherent error accumulation in Simultaneous Localization and Mapping algorithms (SLAM). Based on cadastral plans extracted from OpenStreetMaps (OSM), we build prior maps using a Likelihood Field (LF) which takes into account the inaccuracy found in such plans. The built maps are then used to localize a vehicle equipped with an odometer used to predict its next pose, and a LIDAR used to correct the predicted pose using a matching algorithm. We have also compared the difference between using raw scans versus scans processed to include only vertical planes in the matching algorithm. Experiments in real conditions in two urban environments illustrate the benefits of using cadastral plans to constrain the drift of localization algorithms. Moreover, two metrics were used to analyze our results. The conducted tests lead us to choose a set of parameters that suits the map representation proposed herein.
更多查看译文
关键词
inherent error accumulation,Mapping algorithms,cadastral plans,prior maps,built maps,matching algorithm,localization algorithms,map representation,urban localization,cadastral maps,likelihood field representation,existing resources,vehicle localization,prior passage,mapping vehicle
AI 理解论文
溯源树
样例
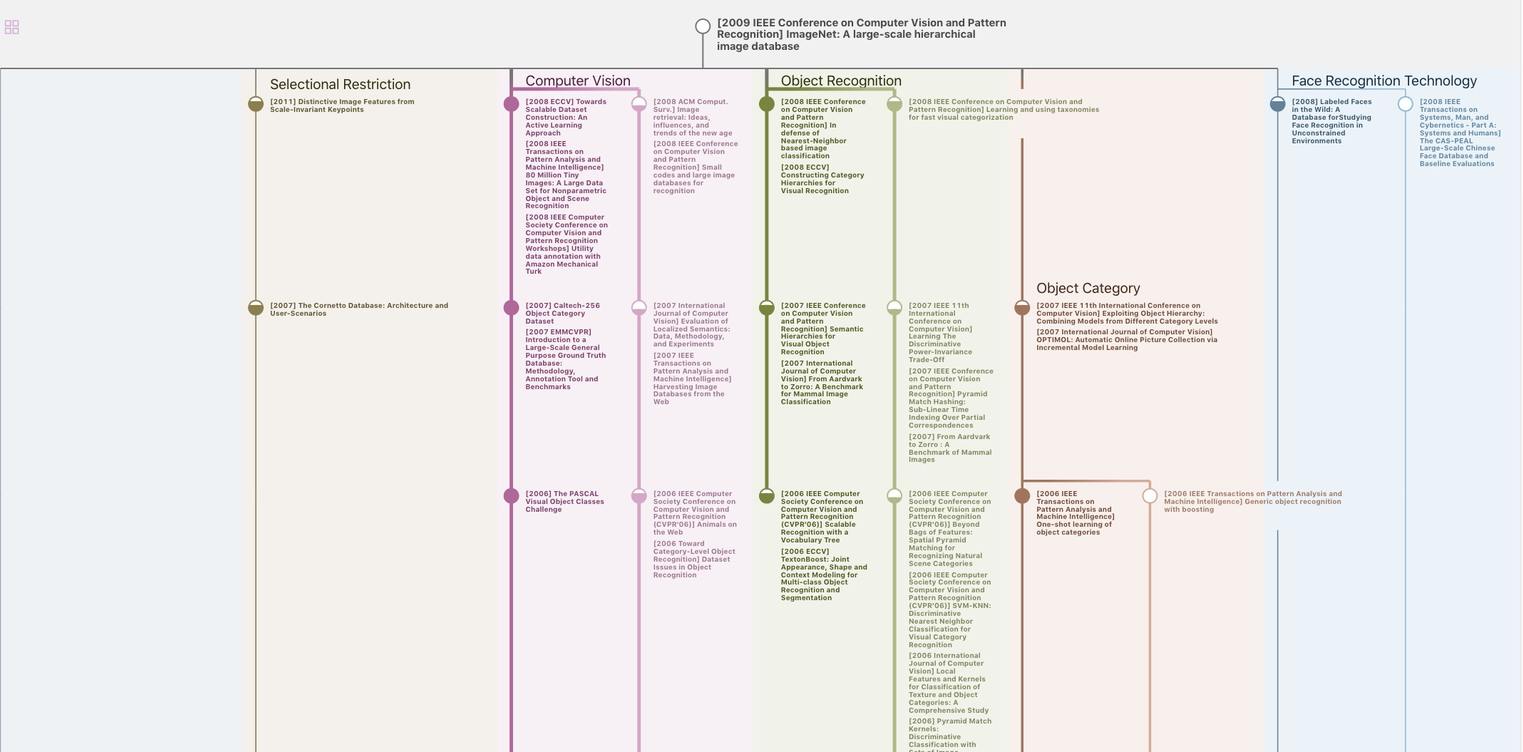
生成溯源树,研究论文发展脉络
Chat Paper
正在生成论文摘要