Deep Learning for Multimodal Fall Detection
2019 IEEE INTERNATIONAL CONFERENCE ON SYSTEMS, MAN AND CYBERNETICS (SMC)(2019)
摘要
Fall detection systems can help providing quick assistance of the person diminishing the severity of the consequences of a fall. Real-time fall detection is important to decrease fear and time that a person remains laying on the floor after falling. In recent years, multimodal fall detection approaches are developed in order to gain more precision and robustness. In this work, we propose a multimodal fall detection system based on wearable sensors, ambient sensors and vision devices. We used long short-term memory networks (LSTM) and convolutional neural networks (CNN) for our analysis given that they are able to extract features from raw data, and are well suited for real-time detection. To test our proposal, we built a public multimodal dataset for fall detection. After experimentation, our proposed method reached 96.4% in accuracy, and it represented an improvement in precision, recall and F 1 -score over using single LSTM or CNN networks for fall detection.
更多查看译文
关键词
Fall detection,multimodal data,real-time system,deep learning,long short-term memory networks,convolutional neural networks
AI 理解论文
溯源树
样例
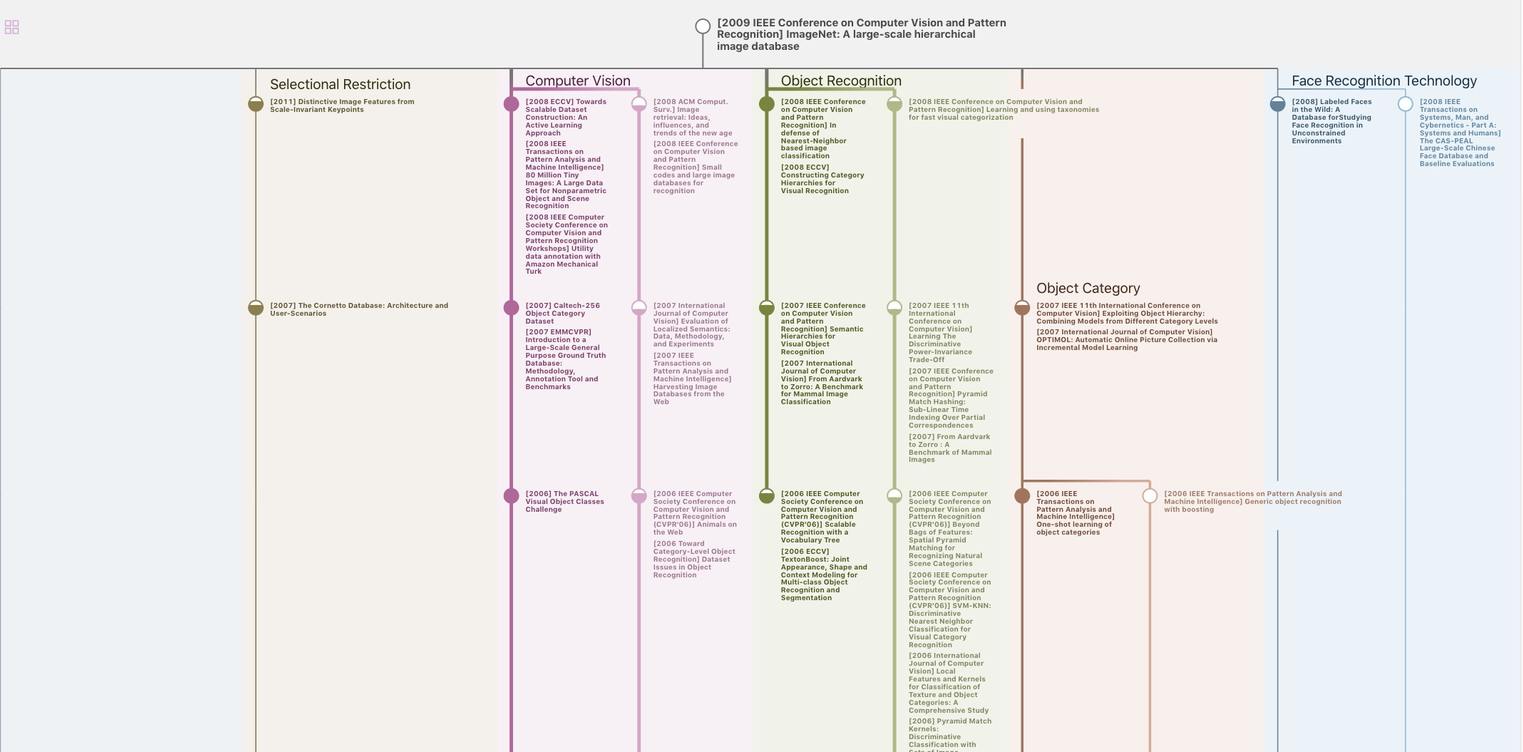
生成溯源树,研究论文发展脉络
Chat Paper
正在生成论文摘要