Learning Embeddings of Intersections on Road Networks.
27TH ACM SIGSPATIAL INTERNATIONAL CONFERENCE ON ADVANCES IN GEOGRAPHIC INFORMATION SYSTEMS (ACM SIGSPATIAL GIS 2019)(2019)
Abstract
Road network is a basic component of intelligent transportation systems (ITS) in smart city. Informative representation of road networks is important as it is essential to a wide variety of ITS applications. In this paper, we propose a neural network representation learning model, namely Intersection of Road Network to Vector (IRN2Vec), to learn embeddings of road intersections that encode rich information in a road network by exploring geo-locality and intrinsic properties of intersections and moving behaviors of road users. In addition to model design, several issues unique to IRN2Vec, including data preparation for model training and various relationships among intersections, are examined. We evaluate the learned embeddings via extensive experiments on three real-world datasets using three downstream test cases, including prediction of traffic signals and crossings on intersections and travel time estimation. Experimental results show that the proposed IRN2Vec outperforms three existing methods, DeepWalk, LINE and Node2vec, in terms of F1-score in predicting traffic signals (22.21% to 23.84%) and crossings (8.65% to 11.65%), and mean absolute error (MAE) in travel time estimation (9.87% to 19.28%).
MoreTranslated text
Key words
Road network,Representation learning,Neural network,Intelligent transportation systems
AI Read Science
Must-Reading Tree
Example
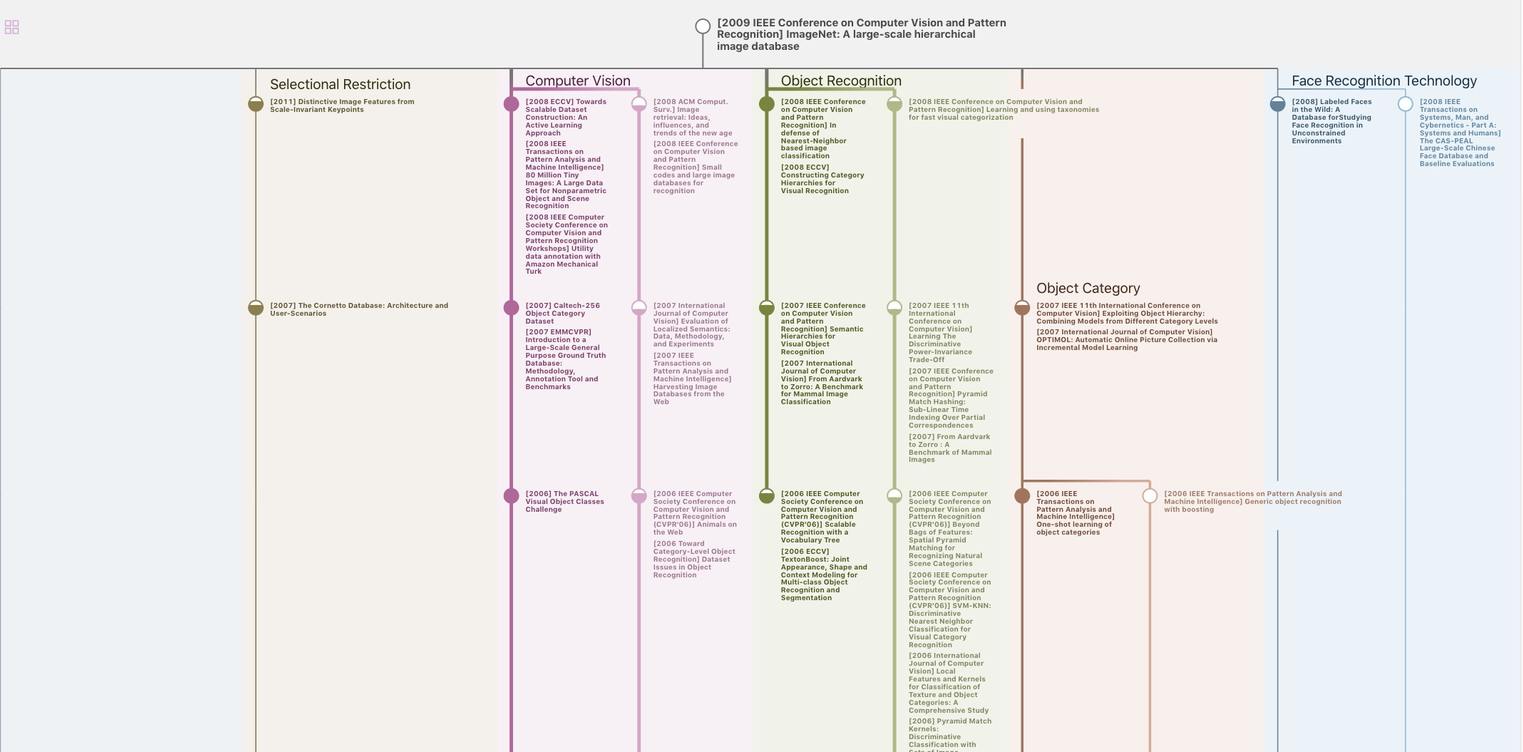
Generate MRT to find the research sequence of this paper
Chat Paper
Summary is being generated by the instructions you defined