Cluster-Based Sample Selection for Document Image Binarization.
2019 International Conference on Document Analysis and Recognition Workshops (ICDARW)(2019)
摘要
The current state-of-the-art, in terms of performance, for solving document image binarization is training artificial neural networks on pre-labelled ground truth data. As such, it faces the same issues as other, more conventional, classification problems; requiring a large amount of training data. However, unlike those conventional classification problems, document image binarization involves having to either manually craft or estimate the binarized ground truth data, which can be error-prone and time-consuming. This is where sample selection, the act of selecting training samples based on some method or metric, might help. By reducing the size of the training dataset in such a way that the binarization performance is not impacted, the required time spent creating the ground truth is also reduced. This paper proposes a cluster-based sample selection method that uses image similarity metrics and the relative neighbourhood graph to reduce the underlying redundancy of the dataset. The method, implemented with affinity propagation and the structural similarity index, reduces the training dataset on average by 49.57% while reducing the binarization performance only by 0.55%.
更多查看译文
关键词
document image binarization,sample selection,neural networks,computer vision
AI 理解论文
溯源树
样例
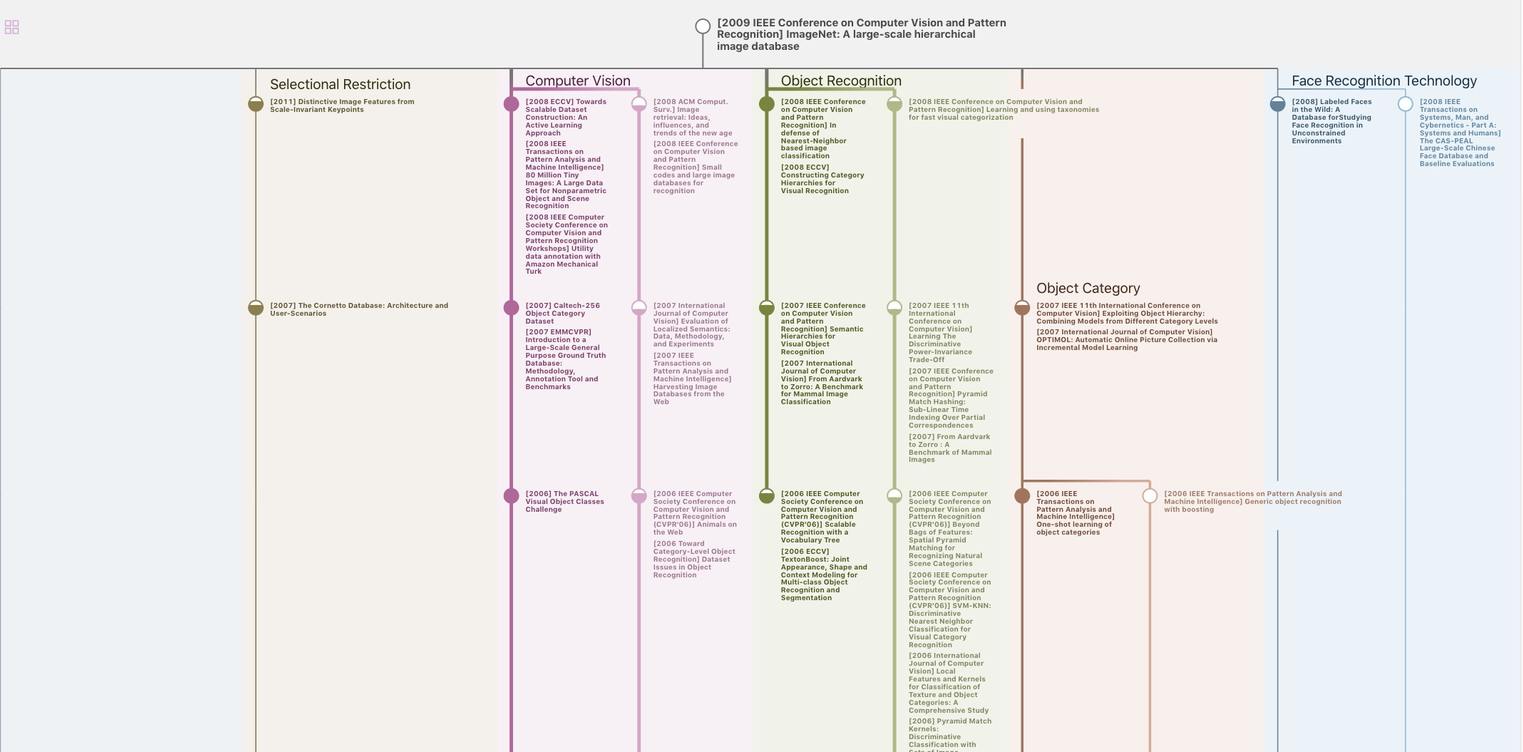
生成溯源树,研究论文发展脉络
Chat Paper
正在生成论文摘要