Scalable Laplacian Regularized Least Squares Classification on Anchor Graph
2019 IEEE International Conference on Big Data, Cloud Computing, Data Science & Engineering (BCD)(2019)
摘要
In this paper, we address the scalability issue of graph-based semi-supervised learnings. In recent years, graph-based semi-supervised learnings using anchor graphs and hierarchical anchor graphs have been proposed as high scalability approaches. However, these techniques utilize linear predictor and cannot infer to unknown data. We propose a Laplacian regularized least squares classification to assume a hierarchical anchor graph (LapRLSC-HAG). The proposed method reduces a memory pressure by the property of the anchor graph or hierarchical anchor graph. Furthermore, we present two strategies optimizing the primal LapRLSC-HAG problems to reduce the computational complexity. To demonstrate the effectiveness of the proposed method, we make experimental evaluations on data sets varying the size from thousands to one million.
更多查看译文
关键词
semi-supervised learning,graph-based learning,manifold regularization,classification,optimization
AI 理解论文
溯源树
样例
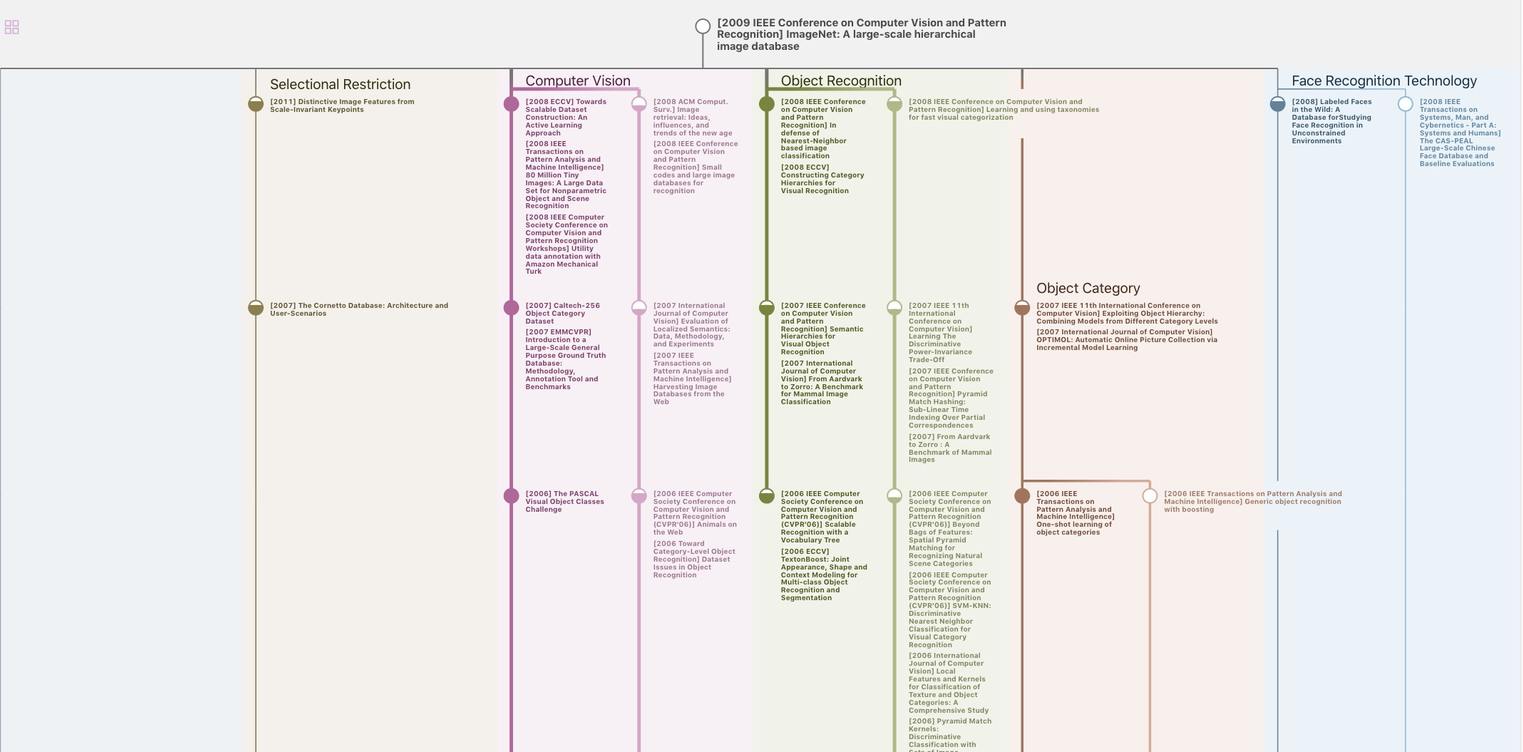
生成溯源树,研究论文发展脉络
Chat Paper
正在生成论文摘要