A Data-Driven Approach for Reconstructing Bifurcation Diagrams of Discrete Dynamical Systems.
LifeTech(2019)
Abstract
In this study, we proposed a data-driven method for reconstructing bifurcation diagrams (BDs) of discrete dynamical systems only from observed time-series, without a priori knowledge of equations governing dynamics of the systems. A deep-autoencoder neural network was utilized to approximate the dynamical equations, in which the system is assumed to be expressed by a linear combination of parameterized basis-functions (BFs). The optimal coefficient values of BFs were searched by a machine learning approach. To reconstruct the BDs of the given systems, we derived principal curves to identify the effective bifurcation parameters from the estimated parameter-space. We validated our method using Logistic map. The proposed algorithm could reconstruct qualitatively similar BDs that preserve important bifurcation structures of the given system, such as period-doubling bifurcations or chaos.
MoreTranslated text
Key words
bifurcation diagram,system identification,machine learning,nonlinear dynamics
AI Read Science
Must-Reading Tree
Example
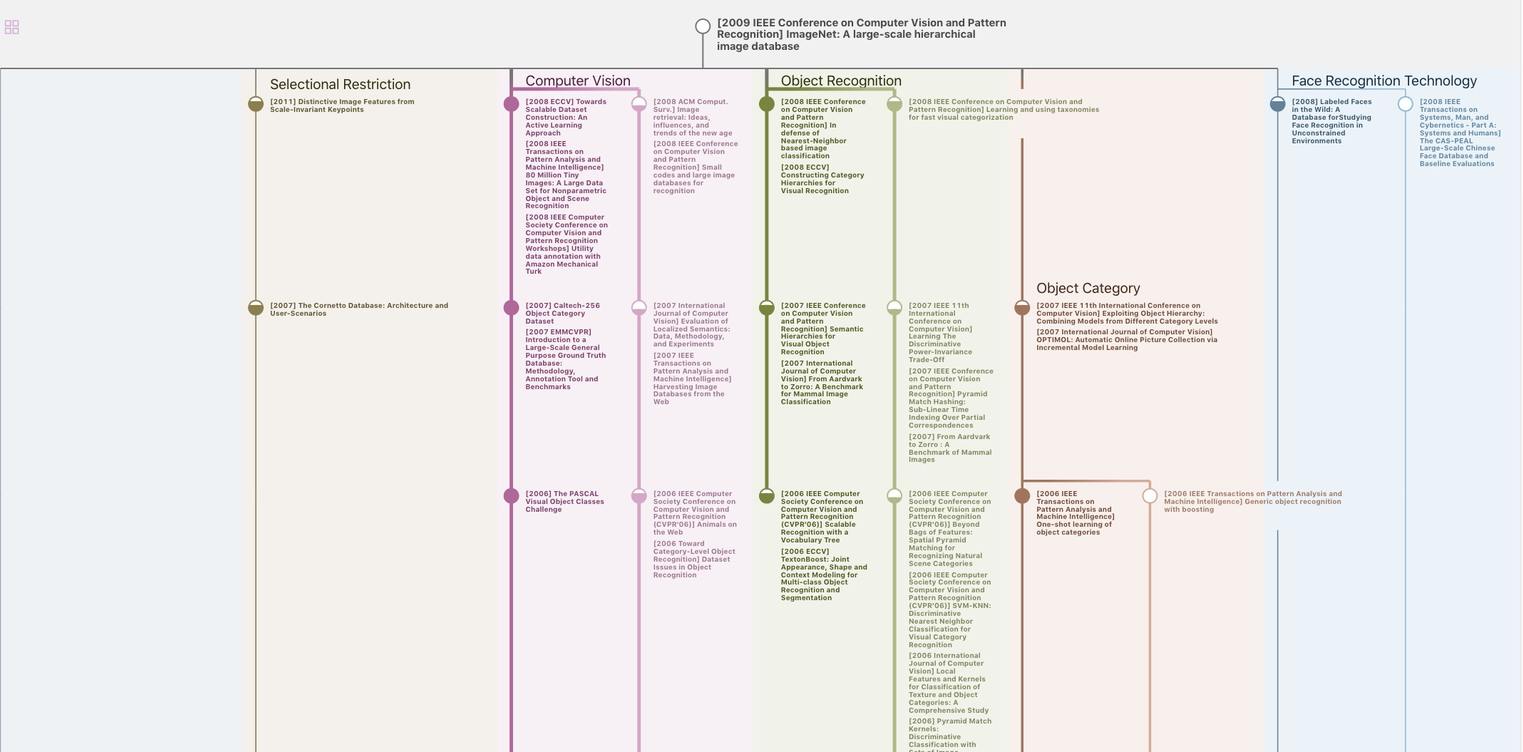
Generate MRT to find the research sequence of this paper
Chat Paper
Summary is being generated by the instructions you defined