Sig-NMS-Based Faster R-CNN Combining Transfer Learning for Small Target Detection in VHR Optical Remote Sensing Imagery
IEEE Transactions on Geoscience and Remote Sensing(2019)
摘要
Small target detection is a challenging task in very-high-resolution (VHR) optical remote sensing imagery, because small targets occupy a minuscule number of pixels and are easily disturbed by backgrounds or occluded by others. Although current convolutional neural network (CNN)-based approaches perform well when detecting normal objects, they are barely suitable for detecting small ones. Two practical problems stand in their way. First, current CNN-based approaches are not specifically designed for the minuscule size of small targets (~15 or ~10 pixels in extent). Second, no well-established data sets include labeled small targets and establishing one from scratch is labor-intensive and time-consuming. To address these two issues, we propose an approach that combines Sig-NMS-based Faster R-CNN with transfer learning. Sig-NMS replaces traditional non-maximum suppression (NMS) in the stage of region proposal network and decreases the possibility of missing small targets. Transfer learning can effectively label remote sensing images by automatically annotating both object classes and object locations. We conduct an experiment on three data sets of VHR optical remote sensing images, RSOD, LEVIR, and NWPU VHR-10, to validate our approach. The results demonstrate that the proposed approach can effectively detect small targets in the VHR optical remote sensing images of about
$10\times 10$
pixels and automatically label small targets as well. In addition, our method presents better mean average precisions than other state-of-the-art methods: 1.5% higher when performing on the RSOD data set, 17.8% higher on the LEVIR data set, and 3.8% higher on NWPU VHR-10.
更多查看译文
关键词
Optical imaging,Optical sensors,Remote sensing,Object detection,Optical fiber networks,Task analysis
AI 理解论文
溯源树
样例
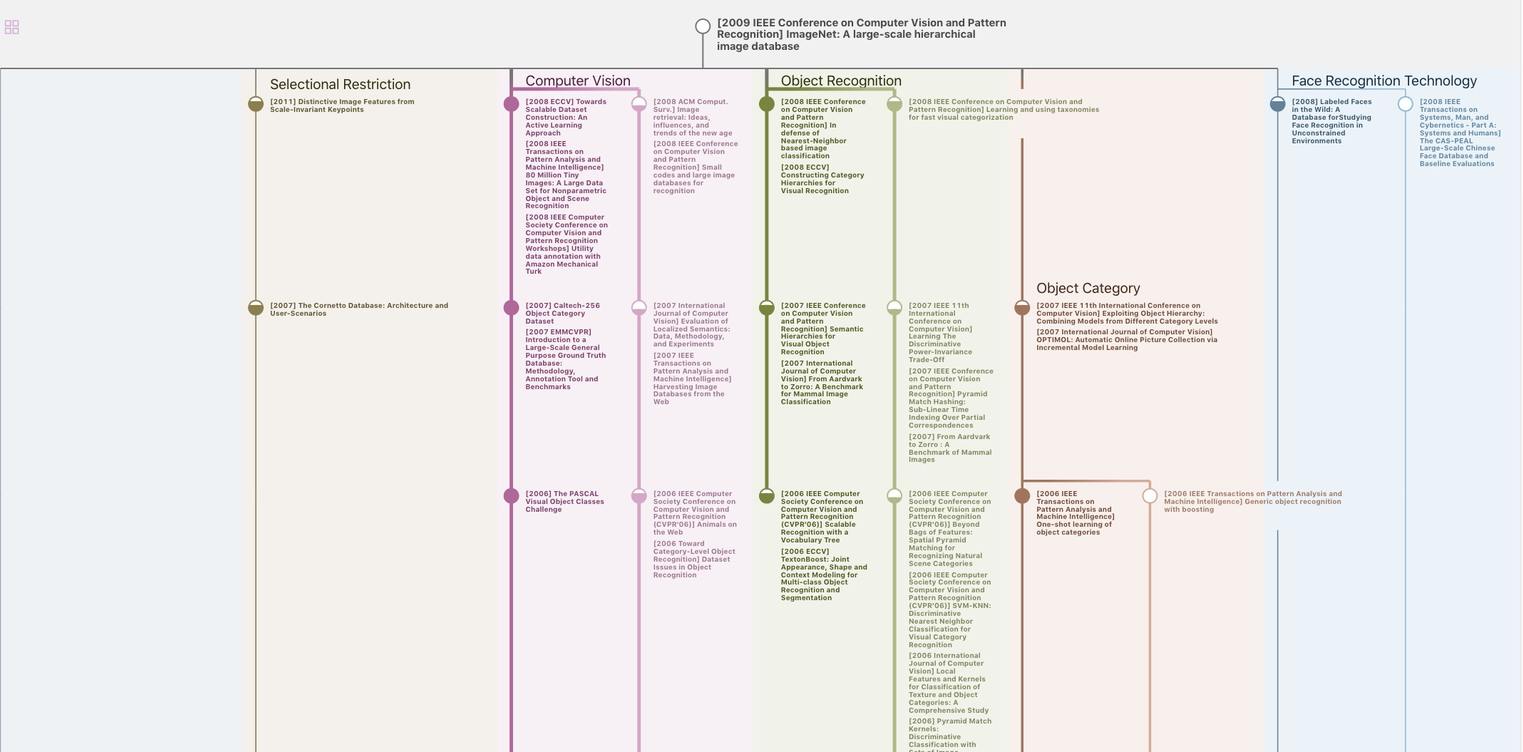
生成溯源树,研究论文发展脉络
Chat Paper
正在生成论文摘要